Some Monotonicity Results For Stochastic Kriging Metamodels In Sequential Settings
INFORMS JOURNAL ON COMPUTING(2018)
摘要
Stochastic kriging (SK) and stochastic kriging with gradient estimators (SKG) are useful methods for effectively approximating the response surface of a simulation model. In this paper, we show that in a fully sequential setting when all model parameters are known, the mean squared errors of the optimal SK and SKG predictors are monotonically decreasing as the number of design points increases. In addition, we prove, under appropriate conditions, that the use of gradient information in the SKG framework generally improves the prediction performance of SK. Motivated by these findings, we propose a sequential procedure for adaptively choosing design points and simulation replications in obtaining SK (SKG) predictors with desired levels of fidelity. We justify the validity of the procedure and carry out numerical experiments to illustrate its performance.
更多查看译文
关键词
simulation, stochastic kriging, sequential sampling
AI 理解论文
溯源树
样例
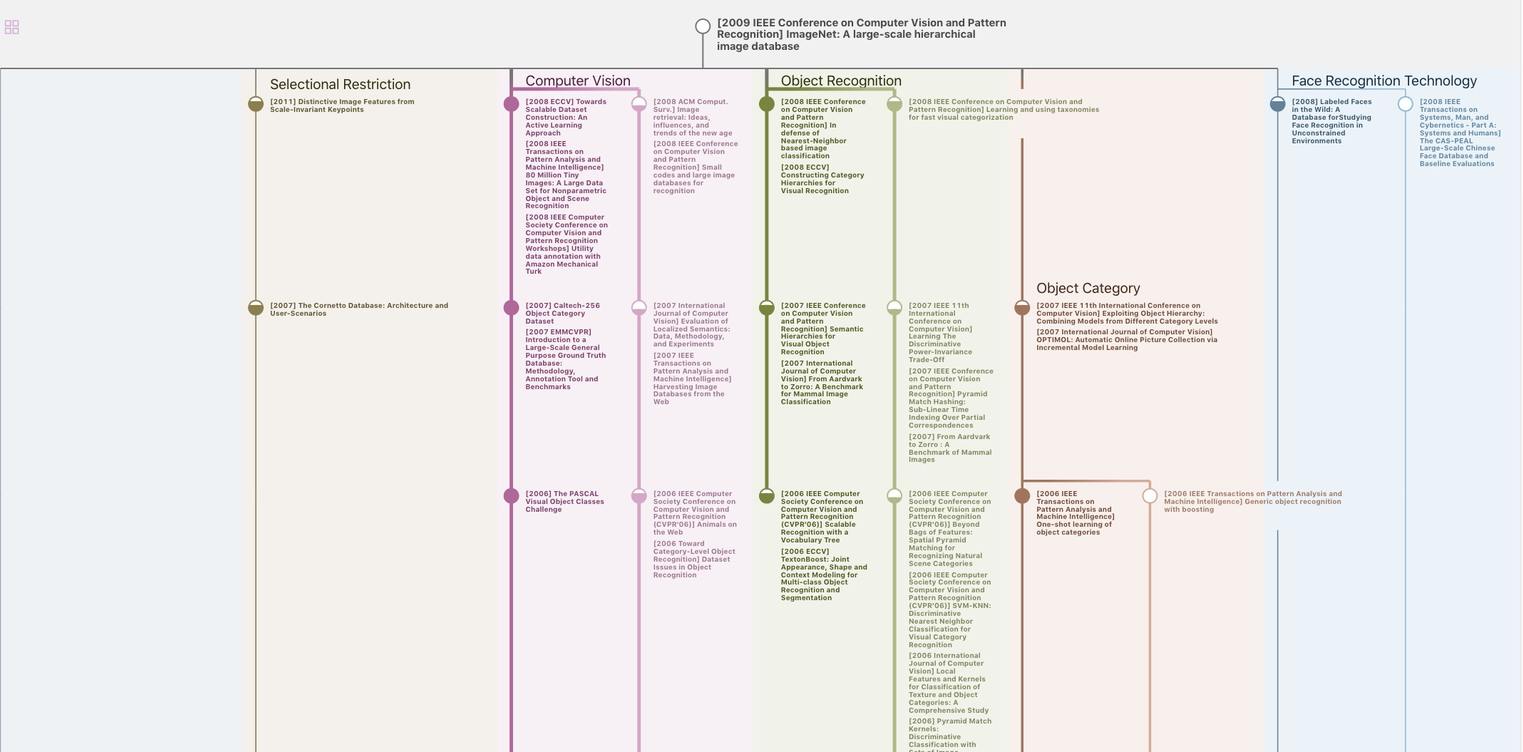
生成溯源树,研究论文发展脉络
Chat Paper
正在生成论文摘要