Deep 2.5D Vehicle Classification with Sparse SfM Depth Prior for Automated Toll Systems
2018 21st International Conference on Intelligent Transportation Systems (ITSC)(2018)
摘要
Automated toll systems rely on proper classification of the passing vehicles. This is especially difficult when the images used for classification only cover parts of the vehicle. To obtain information about the whole vehicle. we reconstruct the vehicle as 3D object and exploit this additional information within a Convolutional Neural Network (CNN). However, when using deep networks for 3D object classification, large amounts of dense 3D models are required for good accuracy, which are often neither available nor feasible to process due to memory requirements. Therefore, in our method we reproject the 3D object onto the image plane using the reconstructed points, lines or both. We utilize this sparse depth prior within an auxiliary network branch that acts as a regularizer during training. We show that this auxiliary regularizer helps to improve accuracy compared to 2D classification on a real-world dataset. Furthermore due to the design of the network, at test time only the 2D camera images are required for classification which enables the usage in portable computer vision systems.
更多查看译文
关键词
deep 2.5D vehicle classification,sparse SfM depth,automated toll systems,passing vehicles,deep networks,3D object classification,dense 3D models,memory requirements,image plane,sparse depth,auxiliary network branch,2D camera images,portable computer vision systems,convolutional neural network
AI 理解论文
溯源树
样例
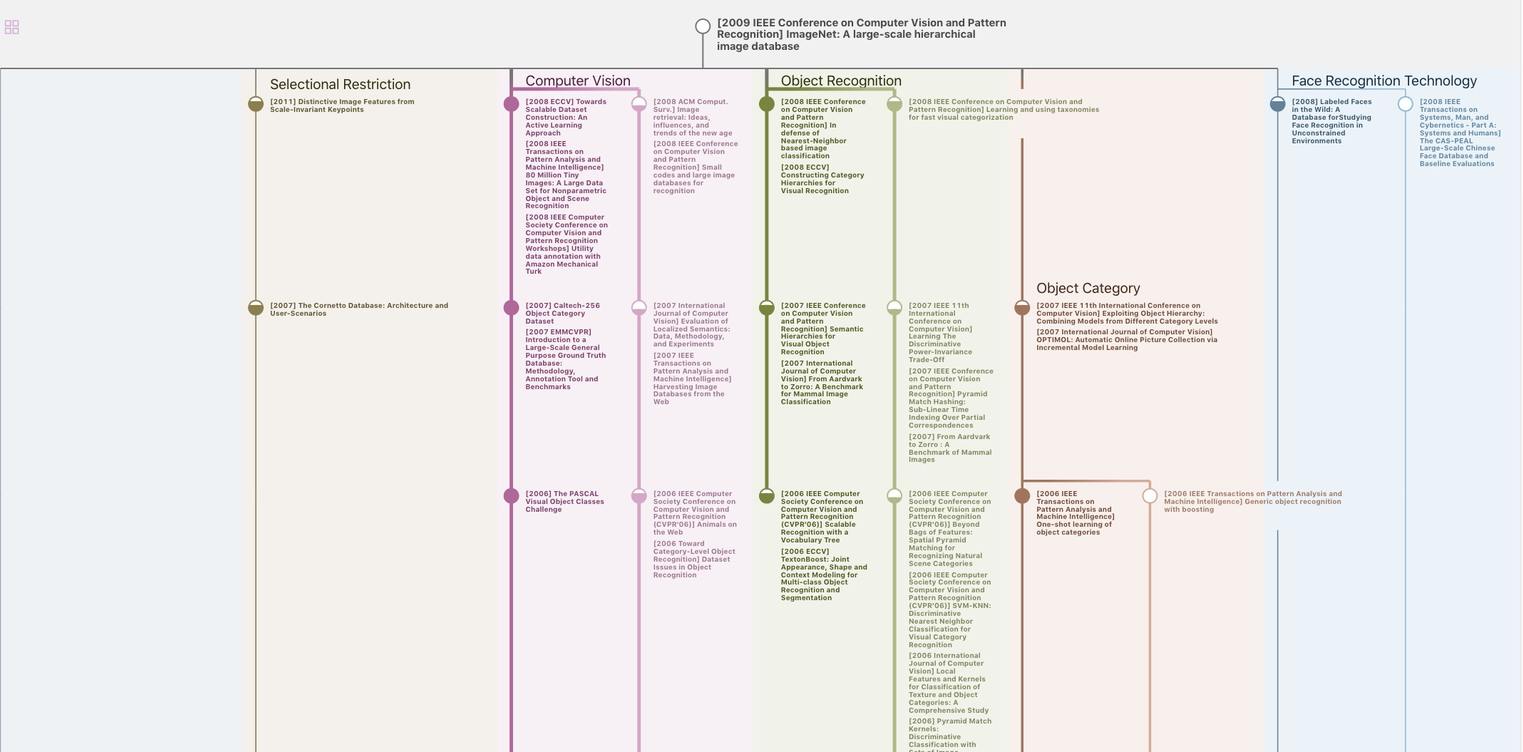
生成溯源树,研究论文发展脉络
Chat Paper
正在生成论文摘要