Learning Safe Policies with Expert Guidance.
ADVANCES IN NEURAL INFORMATION PROCESSING SYSTEMS 31 (NIPS 2018)(2018)
摘要
We propose a framework for ensuring safe behavior of a reinforcement learning agent when the reward function may be difficult to specify. In order to do this, we rely on the existence of demonstrations from expert policies, and we provide a theoretical framework for the agent to optimize in the space of rewards consistent with its existing knowledge. We propose two methods to solve the resulting optimization: an exact ellipsoid-based method and a method in the spirit of the "follow-the-perturbed-leader" algorithm. Our experiments demonstrate the behavior of our algorithm in both discrete and continuous problems. The trained agent safely avoids states with potential negative effects while imitating the behavior of the expert in the other states.
更多查看译文
关键词
the agent,reward function,the space,the existence,theoretical framework
AI 理解论文
溯源树
样例
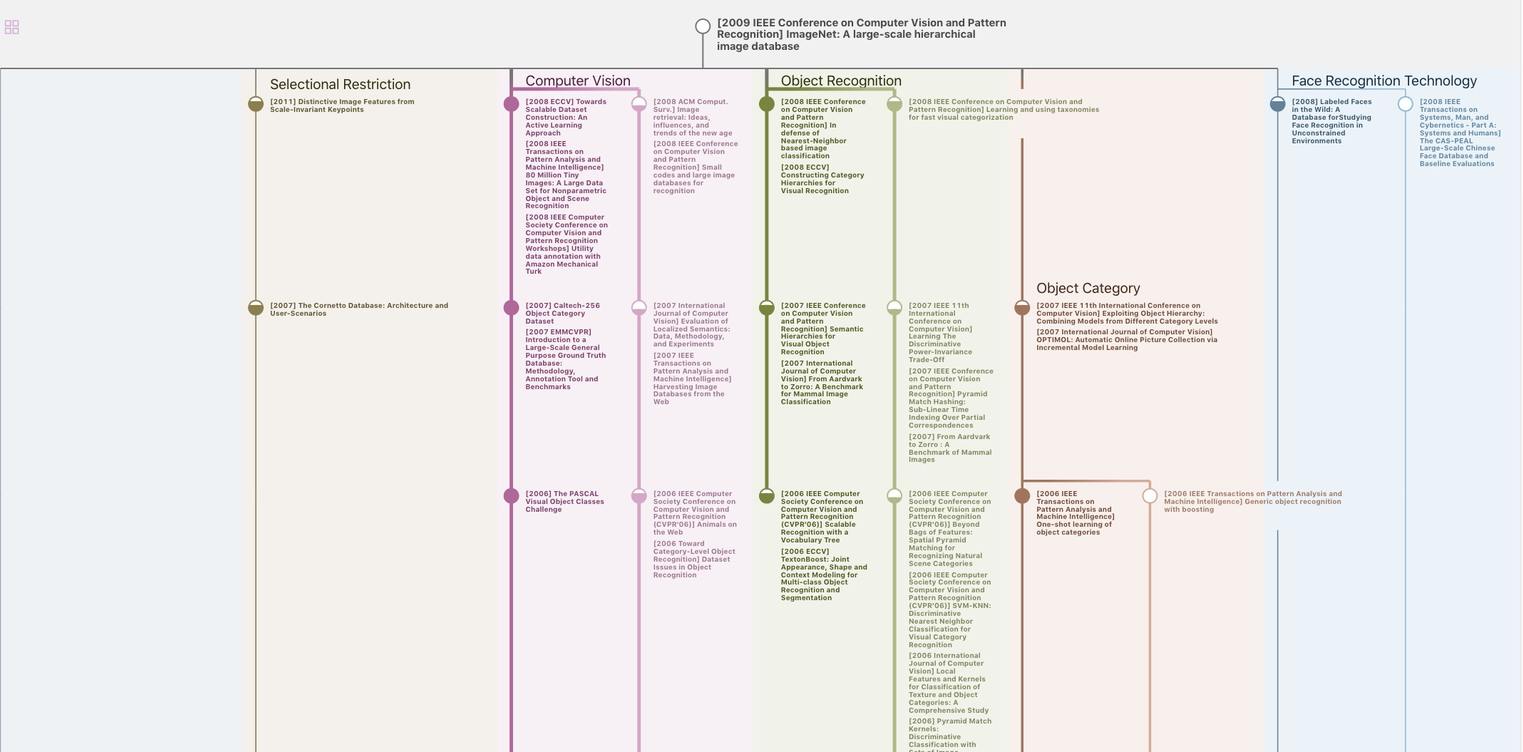
生成溯源树,研究论文发展脉络
Chat Paper
正在生成论文摘要