Tight Bounds for Collaborative PAC Learning via Multiplicative Weights.
ADVANCES IN NEURAL INFORMATION PROCESSING SYSTEMS 31 (NIPS 2018)(2018)
摘要
We study the collaborative PAC learning problem recently proposed in Blum et al. [3], in which we have k players and they want to learn a target function collaboratively, such that the learned function approximates the target function well on all players' distributions simultaneously. The quality of the collaborative learning algorithm is measured by the ratio between the sample complexity of the algorithm and that of the learning algorithm for a single distribution (called the overhead). We obtain a collaborative learning algorithm with overhead O(ln k), improving the one with overhead O(ln(2) k) in [3]. We also show that an Omega(ln k) overhead is inevitable when k is polynomial bounded by the VC dimension of the hypothesis class. Finally, our experimental study has demonstrated the superiority of our algorithm compared with the one in Blum et al. [3] on real-world datasets.
更多查看译文
关键词
sample complexity,learning algorithm,pac learning,collaborative pac learning,target function,experimental study,vc dimension
AI 理解论文
溯源树
样例
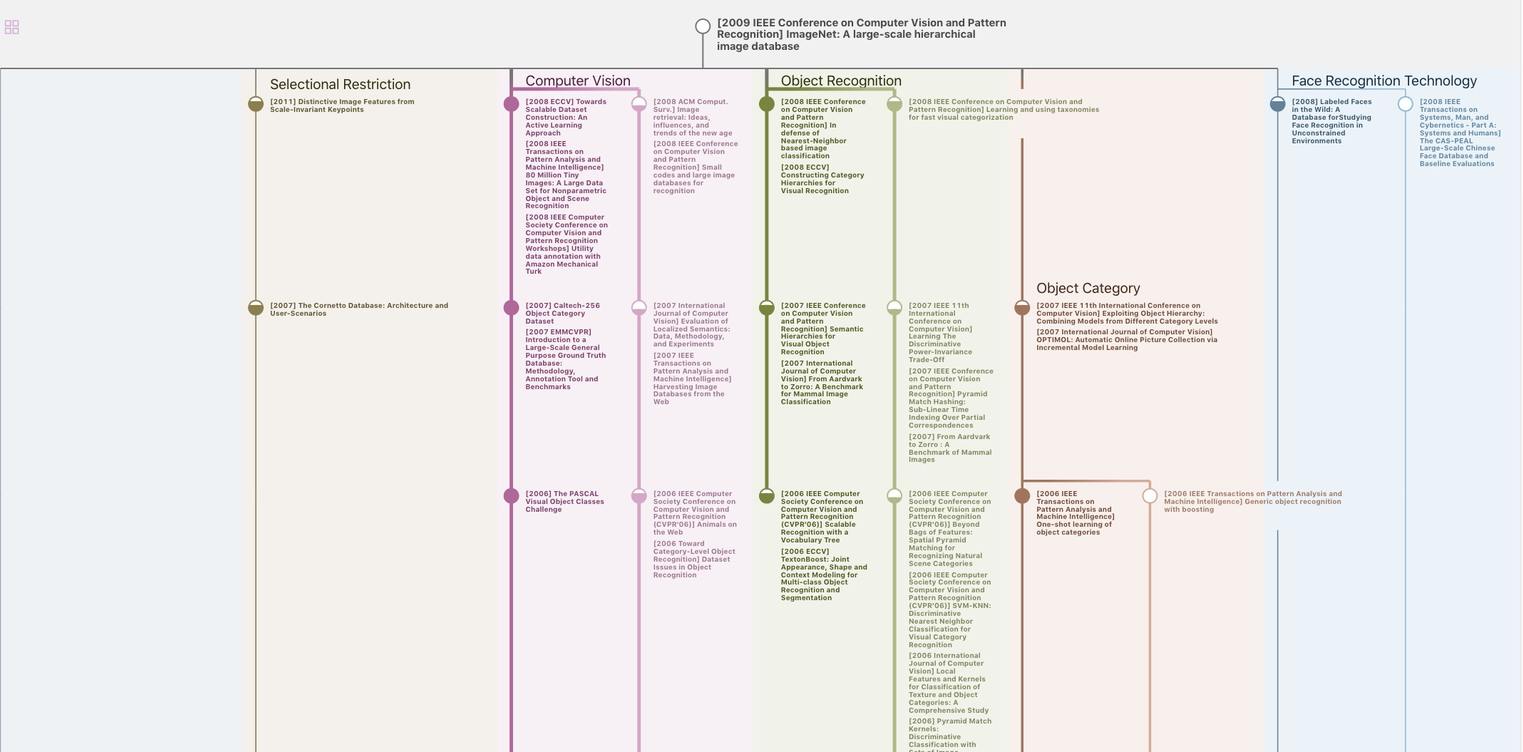
生成溯源树,研究论文发展脉络
Chat Paper
正在生成论文摘要