An Optimal Rewiring Strategy for Reinforcement Social Learning in Cooperative Multiagent Systems.
arXiv: Multiagent Systems(2018)
摘要
Multiagent coordination in cooperative multiagent systems (MASs) has been widely studied in both fixed-agent repeated interaction setting and the static social learning framework. However, two aspects of dynamics in real-world multiagent scenarios are currently missing in existing works. First, the network topologies can be dynamic where agents may change their connections through rewiring during the course of interactions. Second, the game matrix between each pair of agents may not be static and usually not known as a prior. Both the network dynamic and game uncertainty increase the coordination difficulty among agents. In this paper, we consider a multiagent dynamic social learning environment in which each agent can choose to rewire potential partners and interact with randomly chosen neighbors in each round. We propose an optimal rewiring strategy for agents to select most beneficial peers to interact with for the purpose of maximizing the accumulated payoff in repeated interactions. We empirically demonstrate the effectiveness and robustness of our approach through comparing with benchmark strategies. The performance of three representative learning strategies under our social learning framework with our optimal rewiring is investigated as well.
更多查看译文
关键词
cooperative multiagent systems,reinforcement social learning,optimal rewiring strategy
AI 理解论文
溯源树
样例
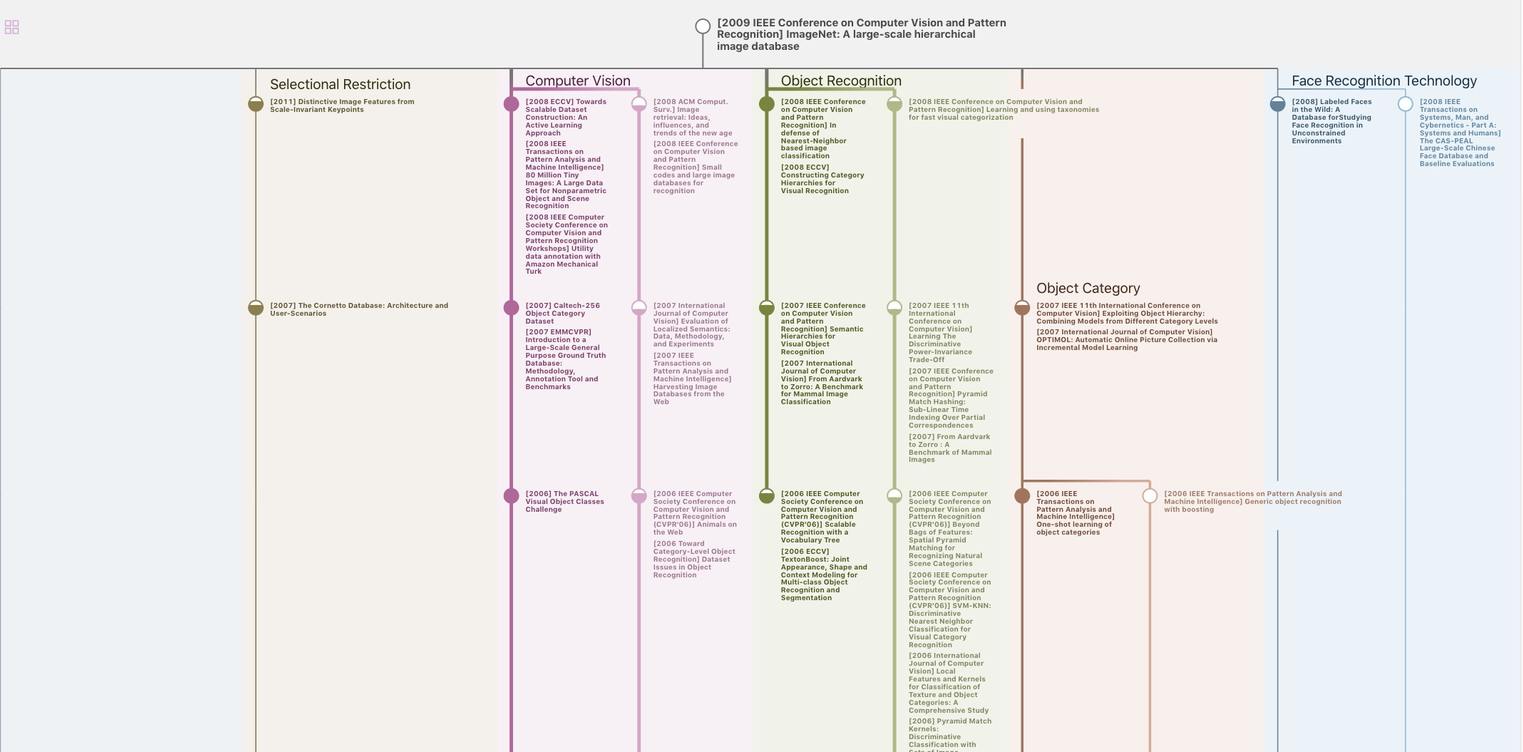
生成溯源树,研究论文发展脉络
Chat Paper
正在生成论文摘要