On Coresets For Logistic Regression
neural information processing systems(2018)
摘要
Coresets are one of the central methods to facilitate the analysis of large data. We continue a recent line of research applying the theory of coresets to logistic regression. First, we show the negative result that no strongly sublinear sized coresets exist for logistic regression. To deal with intractable worst-case instances we introduce a complexity measure mu(X), which quantifies the hardness of compressing a data set for logistic regression. mu(X) has an intuitive statistical interpretation that may be of independent interest. For data sets with bounded mu(X)-complexity, we show that a novel sensitivity sampling scheme produces the first provably sublinear (1 +/- epsilon)-coreset. We illustrate the performance of our method by comparing to uniform sampling as well as to state of the art methods in the area. The experiments are conducted on real world benchmark data for logistic regression.
更多查看译文
关键词
logistic regression,data sets,data set,curriculum learning
AI 理解论文
溯源树
样例
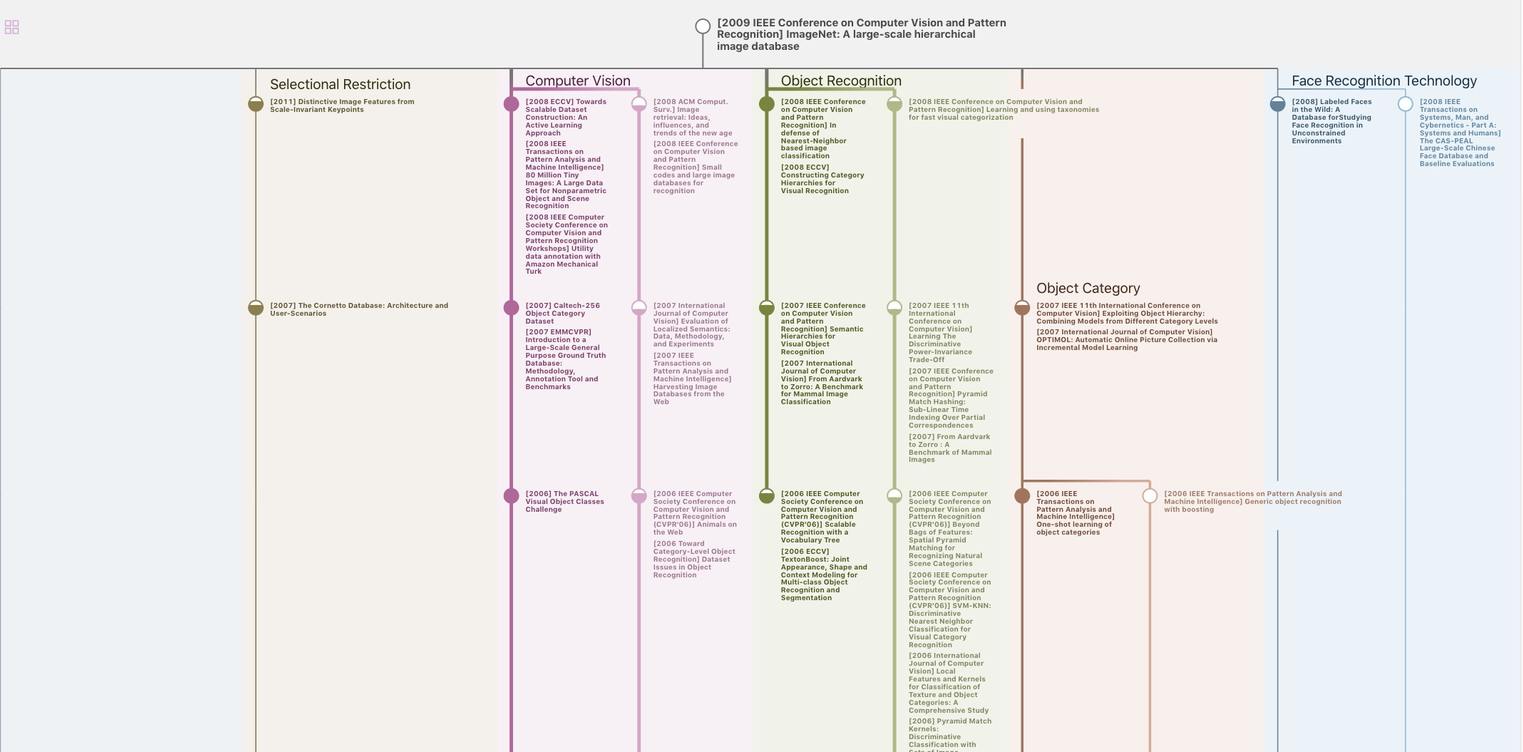
生成溯源树,研究论文发展脉络
Chat Paper
正在生成论文摘要