Variational Inverse Control with Events: A General Framework for Data-Driven Reward Definition.
ADVANCES IN NEURAL INFORMATION PROCESSING SYSTEMS 31 (NIPS 2018)(2018)
摘要
The design of a reward function often poses a major practical challenge to real-world applications of reinforcement learning. Approaches such as inverse reinforcement learning attempt to overcome this challenge, but require expert demonstrations, which can be difficult or expensive to obtain in practice. We propose variational inverse control with events (VICE), which generalizes inverse reinforcement learning methods to cases where full demonstrations are not needed, such as when only samples of desired goal states are available. Our method is grounded in an alternative perspective on control and reinforcement learning, where an agent's goal is to maximize the probability that one or more events will happen at some point in the future, rather than maximizing cumulative rewards. We demonstrate the effectiveness of our methods on continuous control tasks, with a focus on high-dimensional observations like images where rewards are hard or even impossible to specify.
更多查看译文
关键词
reinforcement learning,reward function,inverse reinforcement learning
AI 理解论文
溯源树
样例
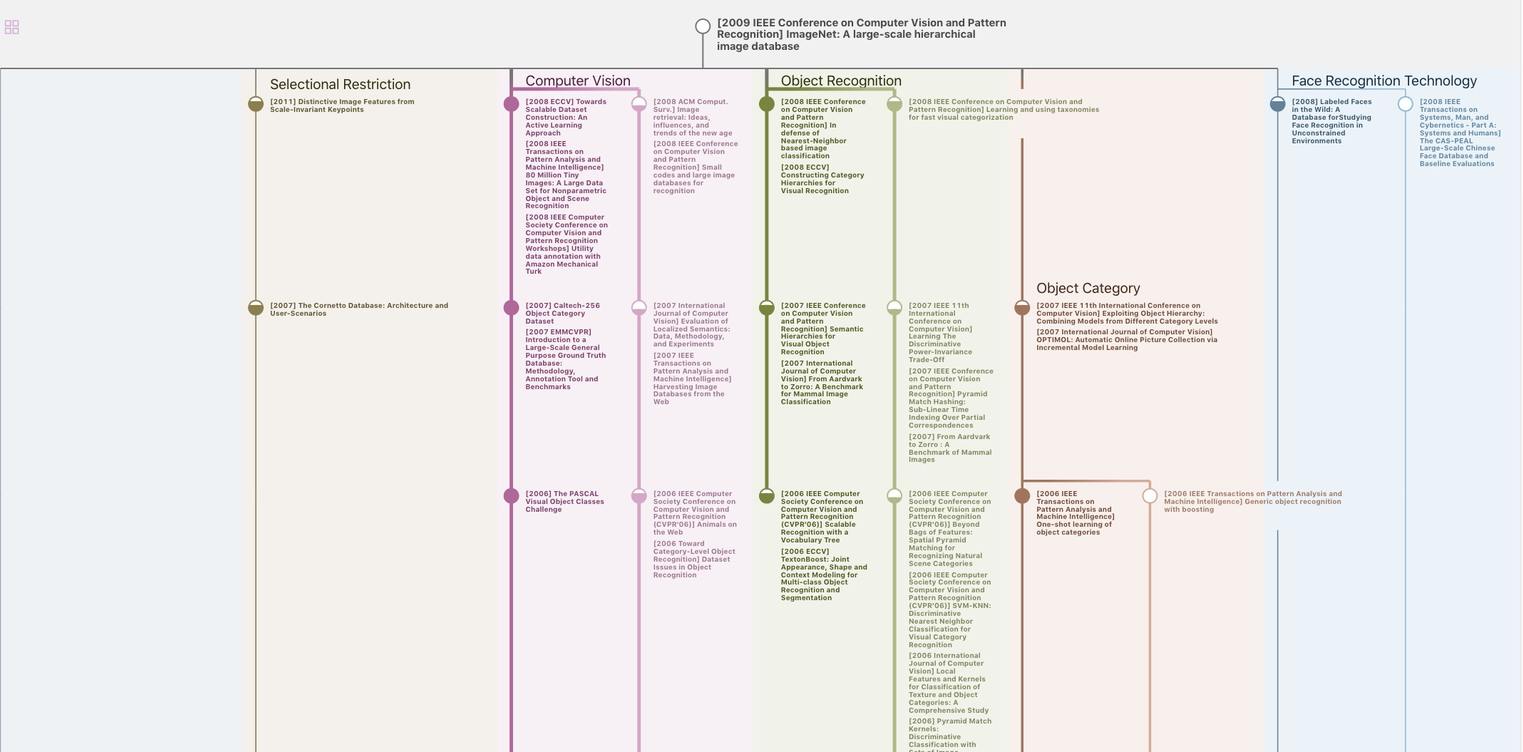
生成溯源树,研究论文发展脉络
Chat Paper
正在生成论文摘要