Arbitrary Style Transfer with Deep Feature Reshuffle
2018 IEEE/CVF Conference on Computer Vision and Pattern Recognition(2018)
摘要
This paper introduces a novel method by reshuffling deep features (i.e., permuting the spacial locations of a feature map) of the style image for arbitrary style transfer. We theoretically prove that our new style loss based on reshuffle connects both global and local style losses respectively used by most parametric and non-parametric neural style transfer methods. This simple idea can effectively address the challenging issues in existing style transfer methods. On one hand, it can avoid distortions in local style patterns, and allow semantic-level transfer, compared with neural parametric methods. On the other hand, it can preserve globally similar appearance to the style image, and avoid wash-out artifacts, compared with neural non-parametric methods. Based on the proposed loss, we also present a progressive feature-domain optimization approach. The experiments show that our method is widely applicable to various styles, and produces better quality than existing methods.
更多查看译文
关键词
arbitrary style transfer,deep feature reshuffle,feature map,style image,optimization approach,nonparametric neural style transfer methods,parametric neural style transfer methods
AI 理解论文
溯源树
样例
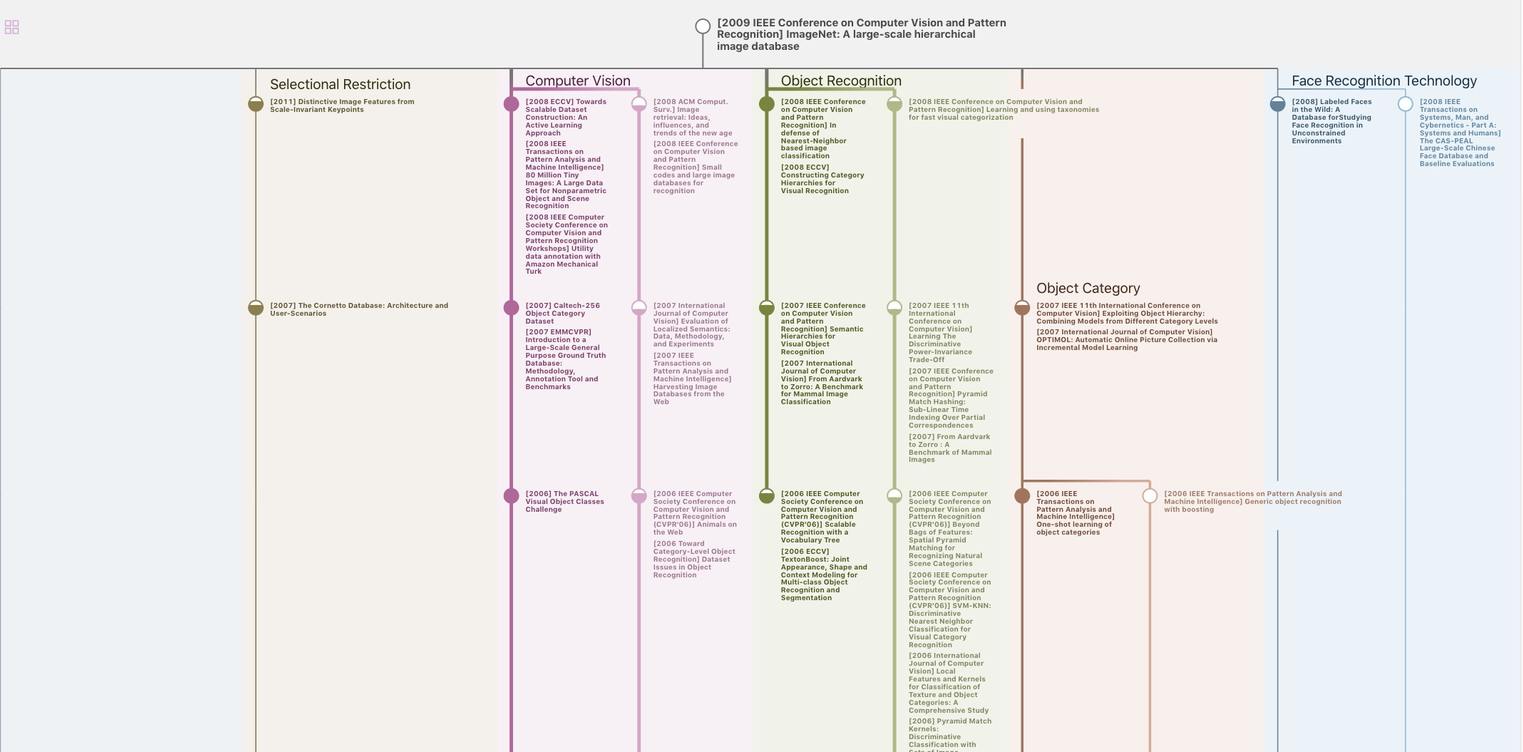
生成溯源树,研究论文发展脉络
Chat Paper
正在生成论文摘要