Hybrid Retrieval-Generation Reinforced Agent for Medical Image Report Generation.
ADVANCES IN NEURAL INFORMATION PROCESSING SYSTEMS 31 (NIPS 2018)(2018)
摘要
Generating long and coherent reports to describe medical images poses challenges to bridging visual patterns with informative human linguistic descriptions. We propose a novel Hybrid Retrieval-Generation Reinforced Agent (HRGR-Agent) which reconciles traditional retrieval-based approaches populated with human prior knowledge, with modern learning-based approaches to achieve structured, robust, and diverse report generation. HRGR-Agent employs a hierarchical decision-making procedure. For each sentence, a high-level retrieval policy module chooses to either retrieve a template sentence from an off-the-shelf template database, or invoke a low-level generation module to generate a new sentence. HRGR-Agent is updated via reinforcement learning, guided by sentence-level and word-level rewards. Experiments show that our approach achieves the state-of-the-art results on two medical report datasets, generating well-balanced structured sentences with robust coverage of heterogeneous medical report contents. In addition, our model achieves the highest detection precision of medical abnormality terminologies, and improved human evaluation performance.
更多查看译文
关键词
reinforcement learning,medical image
AI 理解论文
溯源树
样例
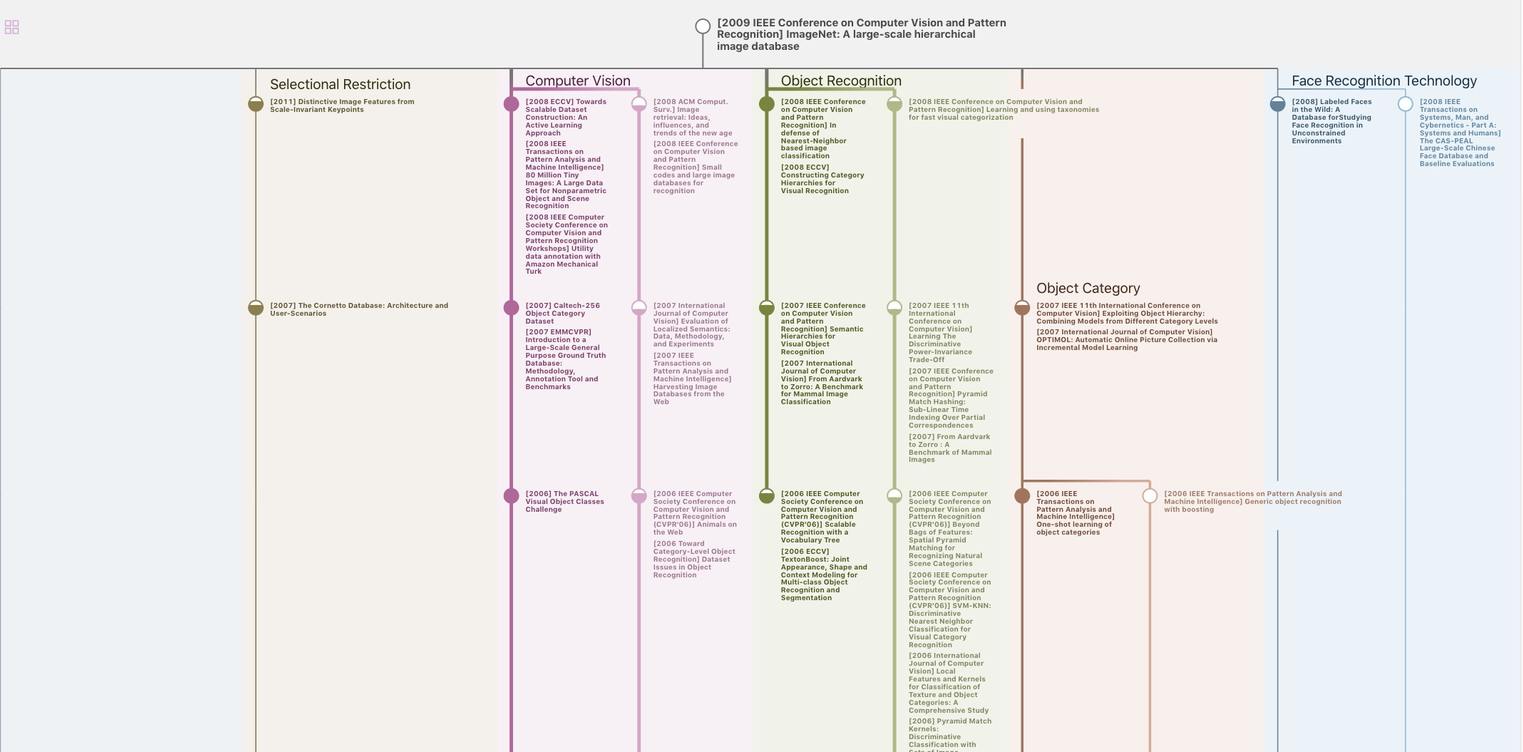
生成溯源树,研究论文发展脉络
Chat Paper
正在生成论文摘要