Projection-Free Algorithms in Statistical Estimation.
arXiv: Machine Learning(2018)
摘要
Frank-Wolfe algorithm (FW) and its variants have gained a surge of interests in machine learning community due to its projection-free property. Recently people have reduced the gradient evaluation complexity of FW algorithm to $log(frac{1}{epsilon})$ for the smooth and strongly convex objective. This complexity result is especially significant in learning problem, as the overwhelming data size makes a single evluation of gradient computational expensive. However, in high-dimensional statistical estimation problems, the objective is typically not strongly convex, and sometimes even non-convex. In this paper, we extend the state-of-the-art FW type algorithms for the large-scale, high-dimensional estimation problem. We show that as long as the objective satisfies {em restricted strong convexity}, and we are not optimizing over statistical limit of the model, the $log(frac{1}{epsilon})$ gradient evaluation complexity could still be attained.
更多查看译文
AI 理解论文
溯源树
样例
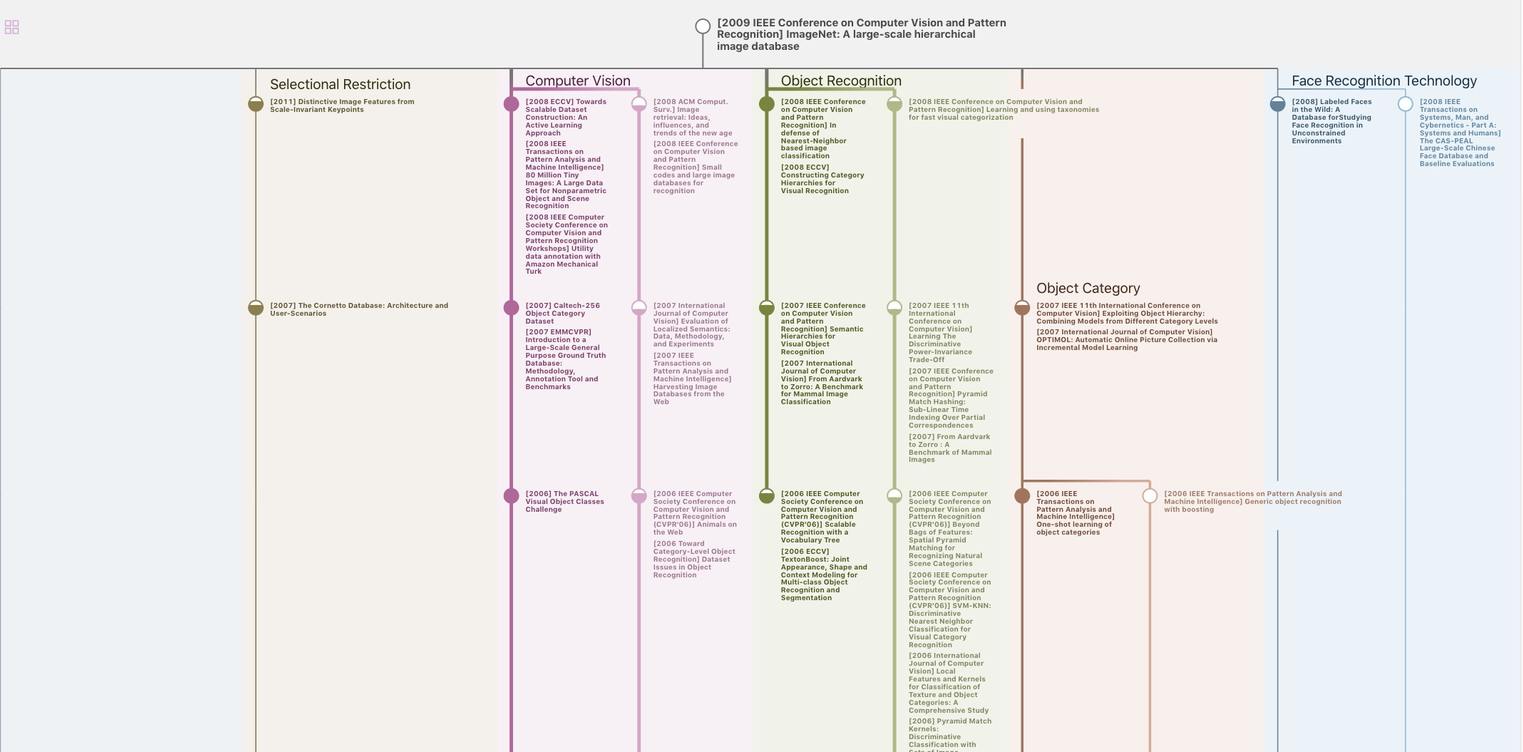
生成溯源树,研究论文发展脉络
Chat Paper
正在生成论文摘要