Learning And Testing Causal Models With Interventions
ADVANCES IN NEURAL INFORMATION PROCESSING SYSTEMS 31 (NIPS 2018)(2018)
摘要
We consider testing and learning problems on causal Bayesian networks as defined by Pearl [Pea09]. Given a causal Bayesian network M on a graph with n discrete variables and bounded in-degree and bounded "confounded components", we show that O(log n) interventions on an unknown causal Bayesian network X on the same graph, and O(n/epsilon(2)) samples per intervention, suffice to efficiently distinguish whether X = M or whether there exists some intervention under which X and M are farther than epsilon in total variation distance. We also obtain sample/time/intervention efficient algorithms for: (i) testing the identity of two unknown causal Bayesian networks on the same graph; and (ii) learning a causal Bayesian network on a given graph. Although our algorithms are non-adaptive, we show that adaptivity does not help in general: Omega(log n) interventions are necessary for testing the identity of two unknown causal Bayesian networks on the same graph, even adaptively. Our algorithms are enabled by a new subadditivity inequality for the squared Hellinger distance between two causal Bayesian networks.
更多查看译文
关键词
efficient algorithms,total variation distance,learning problems,discrete variables
AI 理解论文
溯源树
样例
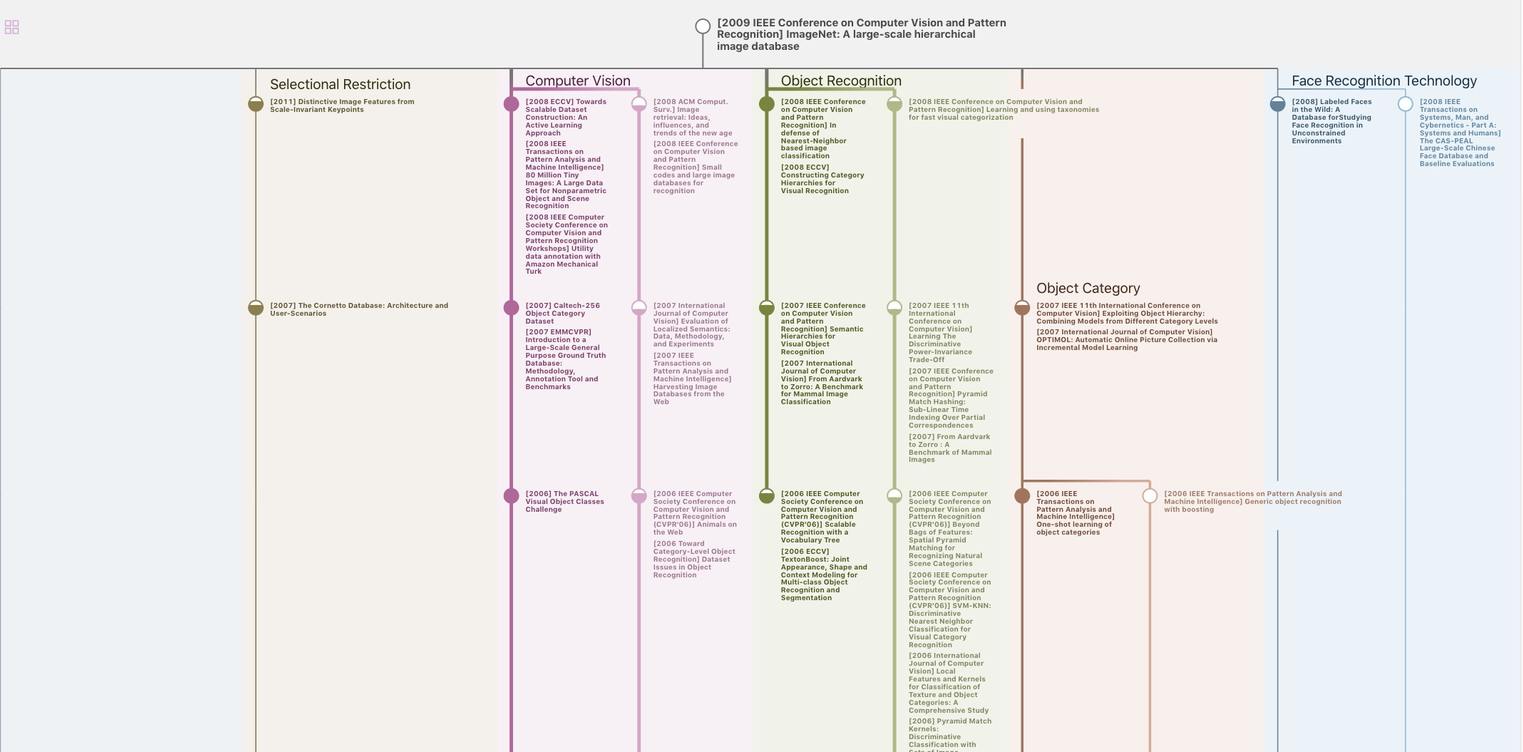
生成溯源树,研究论文发展脉络
Chat Paper
正在生成论文摘要