Neural machine translation for low-resource languages without parallel corpora
Machine Translation(2017)
摘要
The problem of a total absence of parallel data is present for a large number of language pairs and can severely detriment the quality of machine translation. We describe a language-independent method to enable machine translation between a low-resource language (LRL) and a third language, e.g. English. We deal with cases of LRLs for which there is no readily available parallel data between the low-resource language and any other language, but there is ample training data between a closely-related high-resource language (HRL) and the third language. We take advantage of the similarities between the HRL and the LRL in order to transform the HRL data into data similar to the LRL using transliteration. The transliteration models are trained on transliteration pairs extracted from Wikipedia article titles. Then, we automatically back-translate monolingual LRL data with the models trained on the transliterated HRL data and use the resulting parallel corpus to train our final models. Our method achieves significant improvements in translation quality, close to the results that can be achieved by a general purpose neural machine translation system trained on a significant amount of parallel data. Moreover, the method does not rely on the existence of any parallel data for training, but attempts to bootstrap already existing resources in a related language.
更多查看译文
关键词
Neural machine translation, Low-resource languages, Closely related languages, Transliteration
AI 理解论文
溯源树
样例
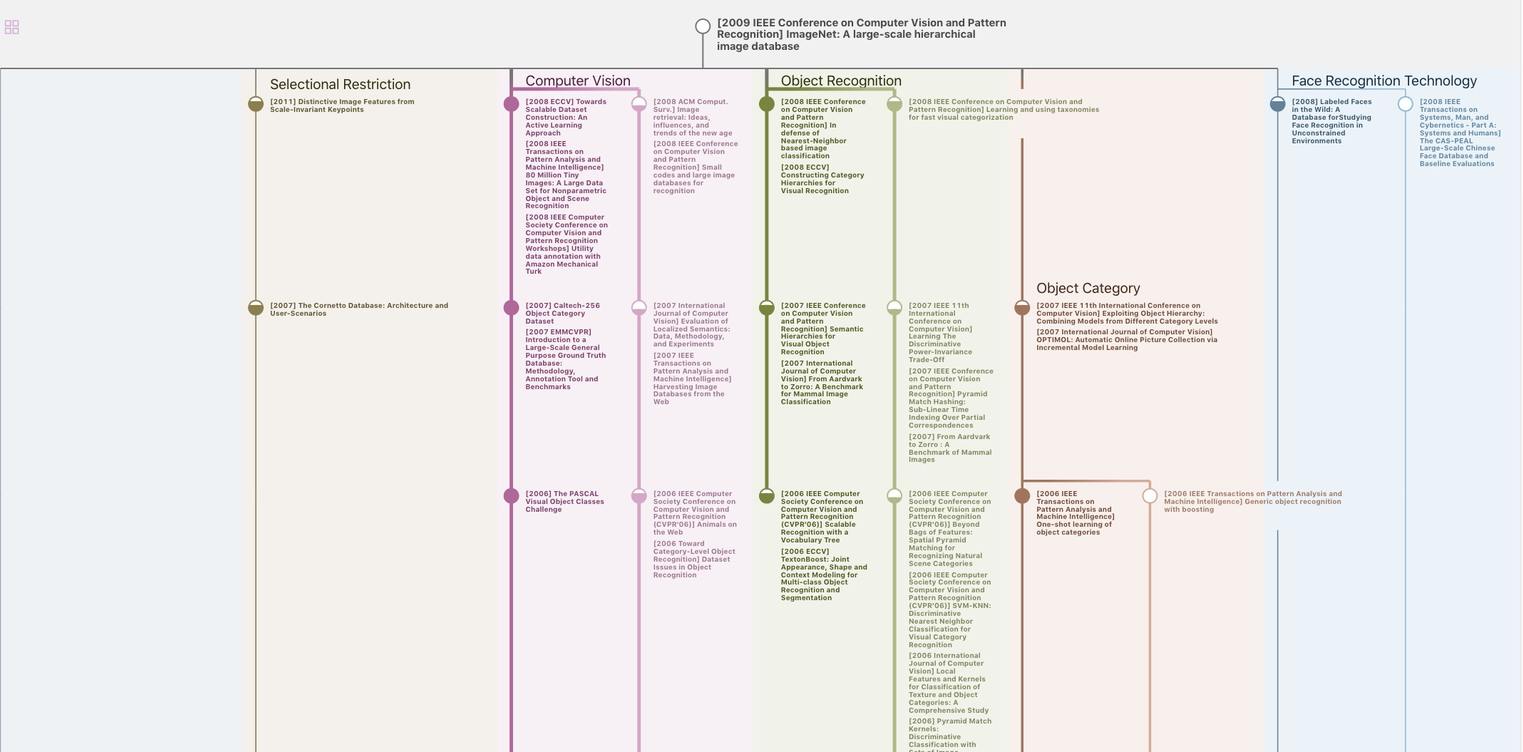
生成溯源树,研究论文发展脉络
Chat Paper
正在生成论文摘要