Locally adaptive multiple kernel k -means algorithm based on shared nearest neighbors
Soft Comput.(2017)
摘要
Most of multiple kernel clustering algorithms aim to find the optimal kernel combination and have to calculate kernel weights iteratively. For the kernel methods, the scale parameter of Gaussian kernel is usually searched in a number of candidate values of the parameter and the best is selected. In this paper, a novel locally adaptive multiple kernel k -means algorithm is proposed based on shared nearest neighbors. Our similarity measure meets the requirements of the clustering hypothesis, which can describe the relations between data points more reasonably by taking local and global structures into consideration. We assign to each data point a local scale parameter and combine the parameter with shared nearest neighbors to construct kernel matrix. According to the local distribution, the local scale parameter of Gaussian kernel is generated adaptively. Experiments show that the proposed algorithm can effectively deal with the clustering problem of data sets with complex structure or multiple scales.
更多查看译文
关键词
Multiple kernel clustering,Kernel k-means,Similarity measure,Clustering analysis
AI 理解论文
溯源树
样例
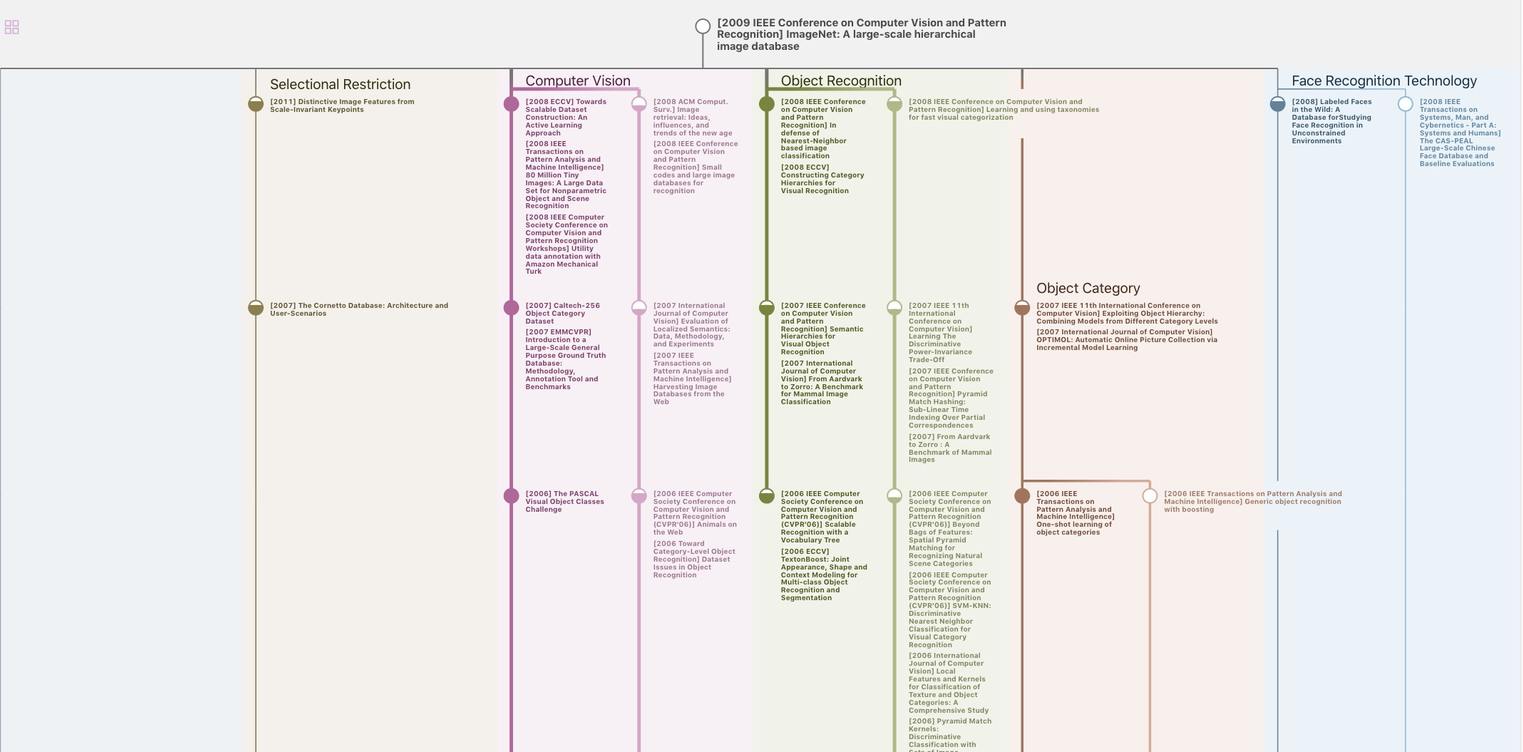
生成溯源树,研究论文发展脉络
Chat Paper
正在生成论文摘要