Feature Importance for Human Epithelial (HEp-2) Cell Image Classification.
JOURNAL OF IMAGING(2018)
摘要
Indirect Immuno-Fluorescence (IIF) microscopy imaging of human epithelial (HEp-2) cells is a popular method for diagnosing autoimmune diseases. Considering large data volumes, computer-aided diagnosis (CAD) systems, based on image-based classification, can help in terms of time, effort, and reliability of diagnosis. Such approaches are based on extracting some representative features from the images. This work explores the selection of the most distinctive features for HEp-2 cell images using various feature selection (FS) methods. Considering that there is no single universally optimal feature selection technique, we also propose hybridization of one class of FS methods (filter methods). Furthermore, the notion of variable importance for ranking features, provided by another type of approaches (embedded methods such as Random forest, Random uniform forest) is exploited to select a good subset of features from a large set, such that addition of new features does not increase classification accuracy. In this work, we have also, with great consideration, designed class-specific features to capture morphological visual traits of the cell patterns. We perform various experiments and discussions to demonstrate the effectiveness of FS methods along with proposed and a standard feature set. We achieve state-of-the-art performance even with small number of features, obtained after the feature selection.
更多查看译文
关键词
feature selection,HEp-2 cell image classification,filter methods,hybridization,random forest,class-specific features
AI 理解论文
溯源树
样例
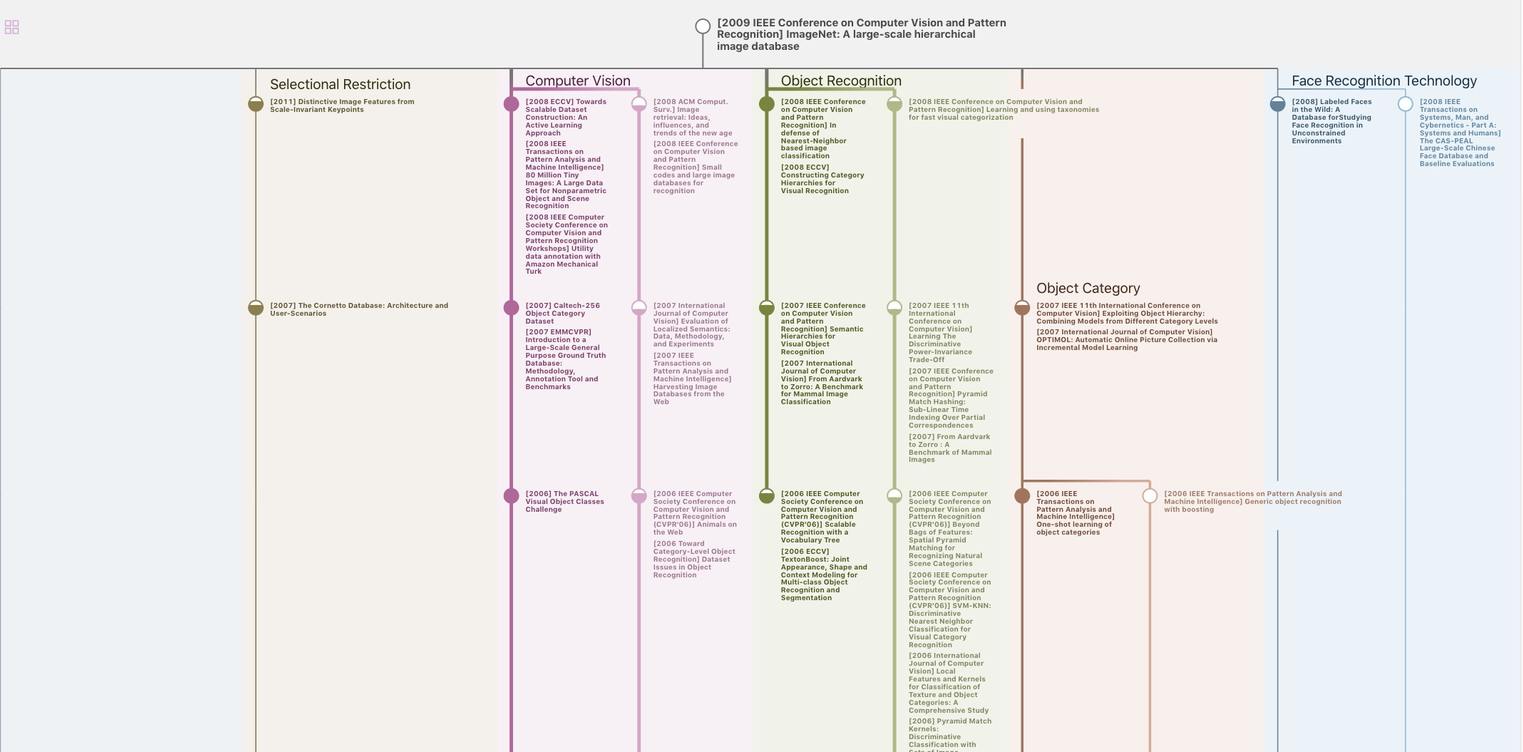
生成溯源树,研究论文发展脉络
Chat Paper
正在生成论文摘要