Approximately optimizing NDCG using pair-wise loss.
Information Sciences(2018)
摘要
The Normalized Discounted Cumulative Gain (NDCG) is used to measure the performance of ranking algorithms. Much of the work on learning to rank by optimizing NDCG directly or indirectly is based on list-wise approaches. In our work, we approximately optimize a variant of NDCG called NDCGβ using pair-wise approaches. NDCGβ utilizes the linear discounting function. We first prove that the DCG error of NDCGβ is equal to the weighted pair-wise loss; then, on that basis, RankBoostndcg and RankSVMndcg are proposed to optimize the upper bound of the pair-wise 0–1 loss function. The experimental results from applying our approaches and ten other state-of-the-art methods to five public datasets show the superiority of the proposed methods, especially RankSVMndcg. In addition, RankBoostndcg are less influenced by the initial weight distribution.
更多查看译文
关键词
Learning to rank,Pair-wise method,Optimization of NDCG,DCG error,Ranking loss
AI 理解论文
溯源树
样例
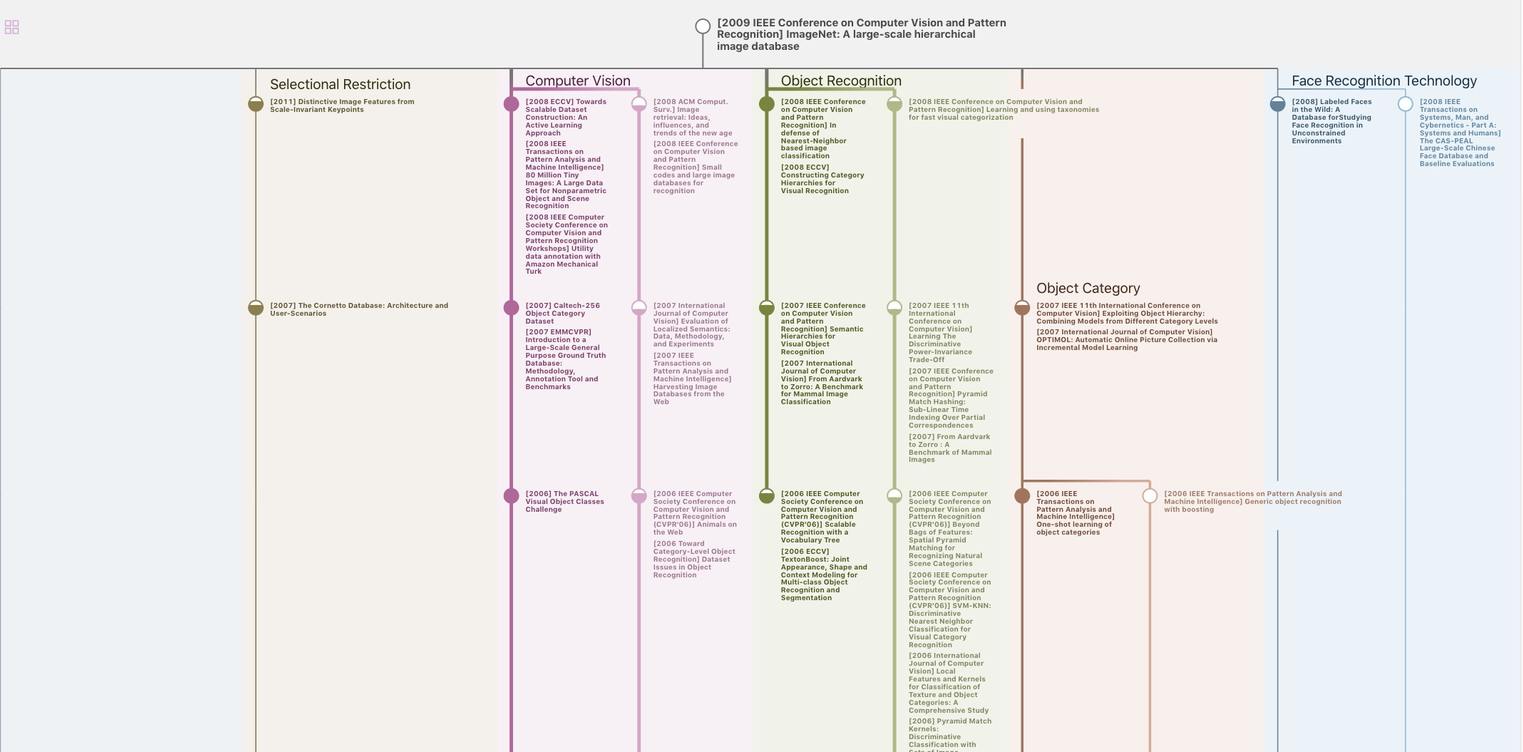
生成溯源树,研究论文发展脉络
Chat Paper
正在生成论文摘要