Dynamic affinity-based classification of multi-class imbalanced data with one-versus-one decomposition: a fuzzy rough set approach
Knowl. Inf. Syst.(2017)
摘要
Class imbalance occurs when data elements are unevenly distributed among classes, which poses a challenge for classifiers. The core focus of the research community has been on binary-class imbalance, although there is a recent trend toward the general case of multi-class imbalanced data. The IFROWANN method, a classifier based on fuzzy rough set theory, stands out for its performance in two-class imbalanced problems. In this paper, we consider its extension to multi-class data by combining it with one-versus-one decomposition. The latter transforms a multi-class problem into two-class sub-problems. Binary classifiers are applied to these sub-problems, after which their outcomes are aggregated into one prediction. We enhance the integration of IFROWANN in the decomposition scheme in two steps. Firstly, we propose an adaptive weight setting for the binary classifier, addressing the varying characteristics of the sub-problems. We call this modified classifier IFROWANN- 𝒲_IR . Second, we develop a new dynamic aggregation method called WV–FROST that combines the predictions of the binary classifiers with the global class affinity before making a final decision. In a meticulous experimental study, we show that our complete proposal outperforms the state-of-the-art on a wide range of multi-class imbalanced datasets.
更多查看译文
关键词
Imbalanced data,Multi-class classification,One-versus-one,Fuzzy rough set theory
AI 理解论文
溯源树
样例
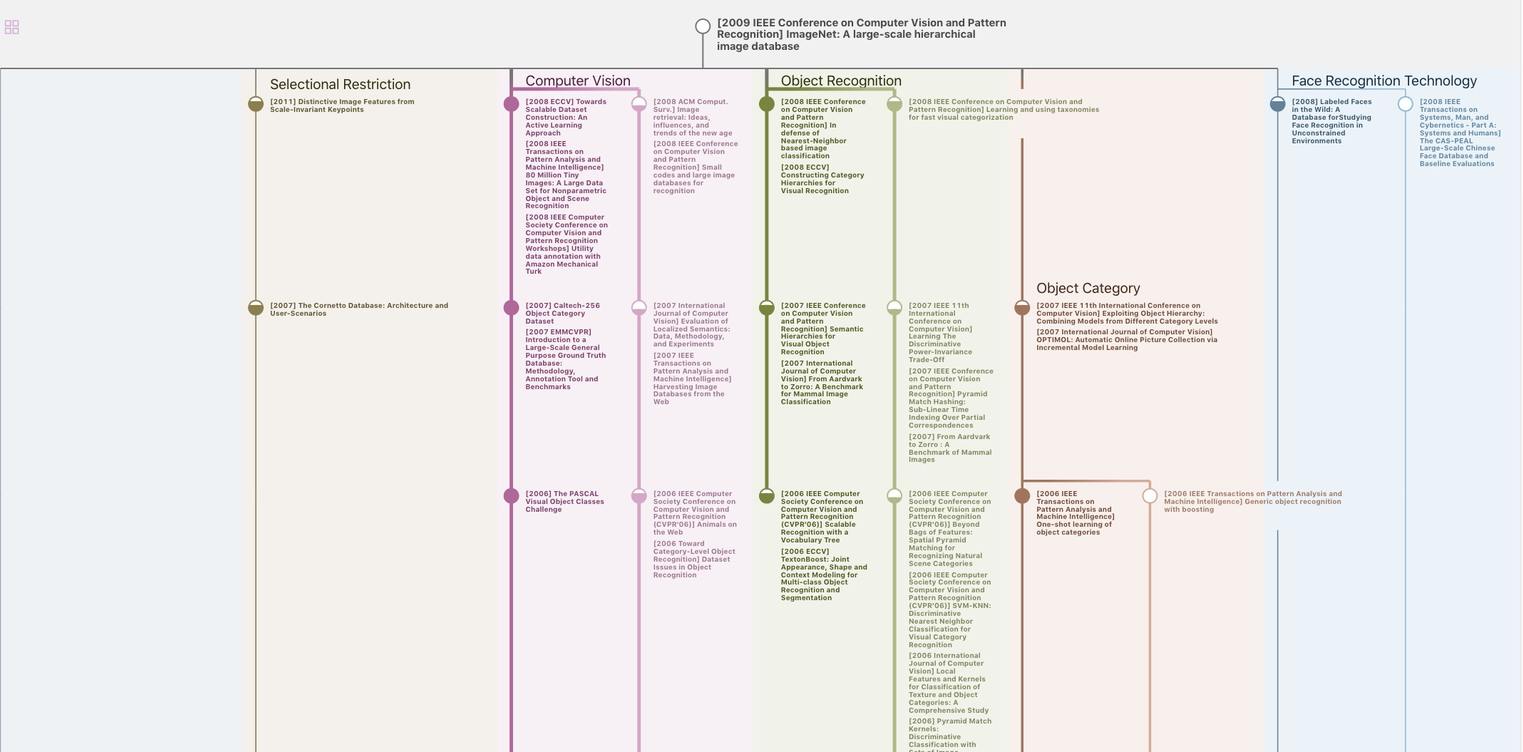
生成溯源树,研究论文发展脉络
Chat Paper
正在生成论文摘要