Three local search-based methods for feature selection in credit scoring
Vietnam J. Computer Science(2018)
摘要
Credit scoring is a crucial problem in both finance and banking. In this paper, we tackle credit scoring as a classification problem where three local search-based methods are studied for feature selection. The feature selection is an interesting technique that can be launched before the data classification task. It permits to keep only the relevant variables and eliminate the redundant ones which enhances the classification accuracy. We study the local search method (LS), the stochastic local search method (SLS) and the variable neighborhood search method (VNS) for feature selection. Then, we combine these methods with the support vector machine (SVM) classifier to find the best described model from a dataset with the correct class variable. The proposed methods (LS+SVM, SLS+SVM and VNS+SVM) are evaluated on both German and Australian credit datasets and compared with some well-known classifiers. The numerical results are promising and show a good performance in favor of our methods.
更多查看译文
关键词
Credit scoring, feature selection, variable selection, classification, support vector machine, optimization, local search, stochastic local search, variable neighborhood search
AI 理解论文
溯源树
样例
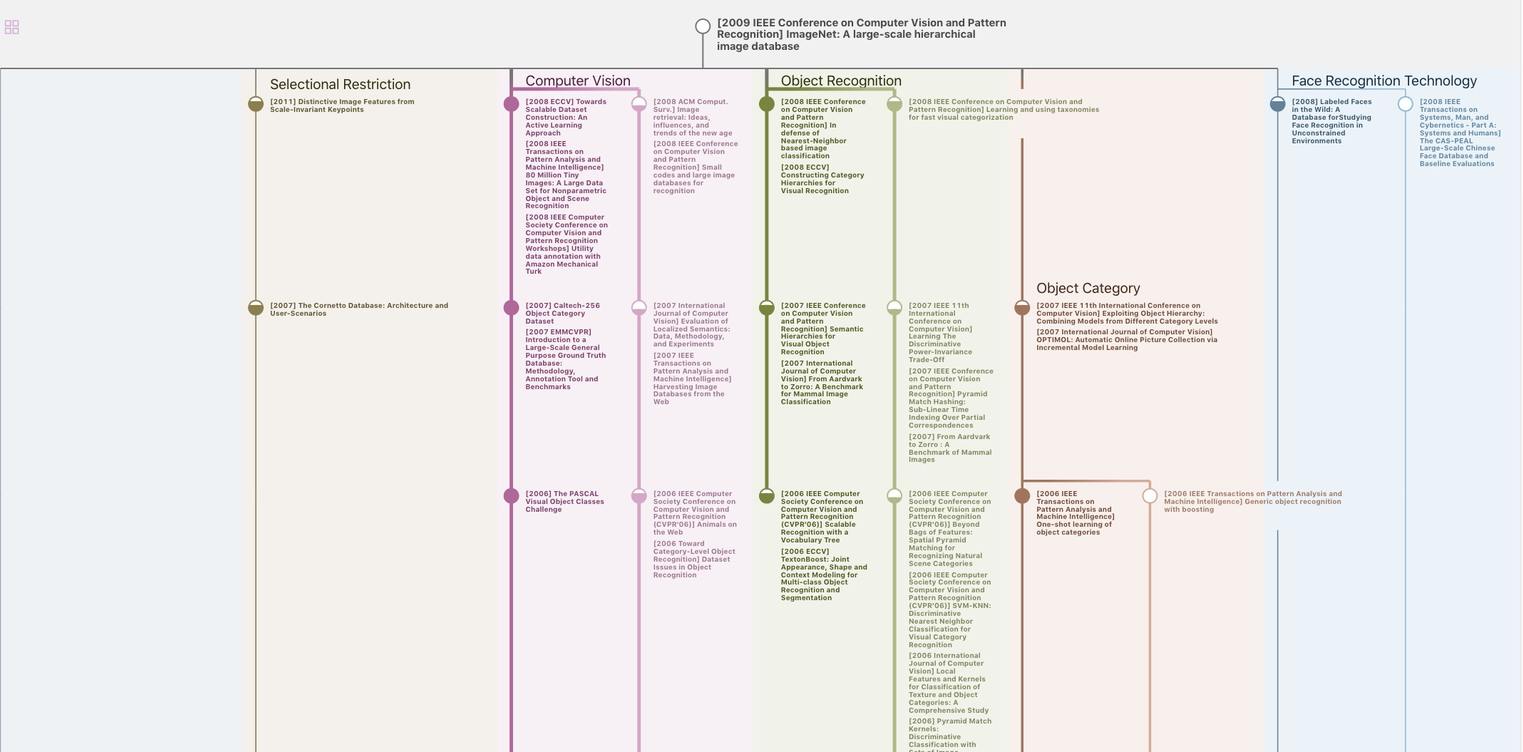
生成溯源树,研究论文发展脉络
Chat Paper
正在生成论文摘要