DeepProduct: Mobile Product Search With Portable Deep Features.
TOMCCAP(2018)
摘要
Features extracted by deep networks have been popular in many visual search tasks. This article studies deep network structures and training schemes for mobile visual search. The goal is to learn an effective yet portable feature representation that is suitable for bridging the domain gap between mobile user photos and (mostly) professionally taken product images while keeping the computational cost acceptable for mobile-based applications. The technical contributions are twofold. First, we propose an alternative of the contrastive loss popularly used for training deep Siamese networks, namely robust contrastive loss, where we relax the penalty on some positive and negative pairs to alleviate overfitting. Second, a simple multitask fine-tuning scheme is leveraged to train the network, which not only utilizes knowledge from the provided training photo pairs but also harnesses additional information from the large ImageNet dataset to regularize the fine-tuning process. Extensive experiments on challenging real-world datasets demonstrate that both the robust contrastive loss and the multitask fine-tuning scheme are effective, leading to very promising results with a time cost suitable for mobile product search scenarios.
更多查看译文
关键词
Mobile product search, contrastive loss, deep learning, efficiency
AI 理解论文
溯源树
样例
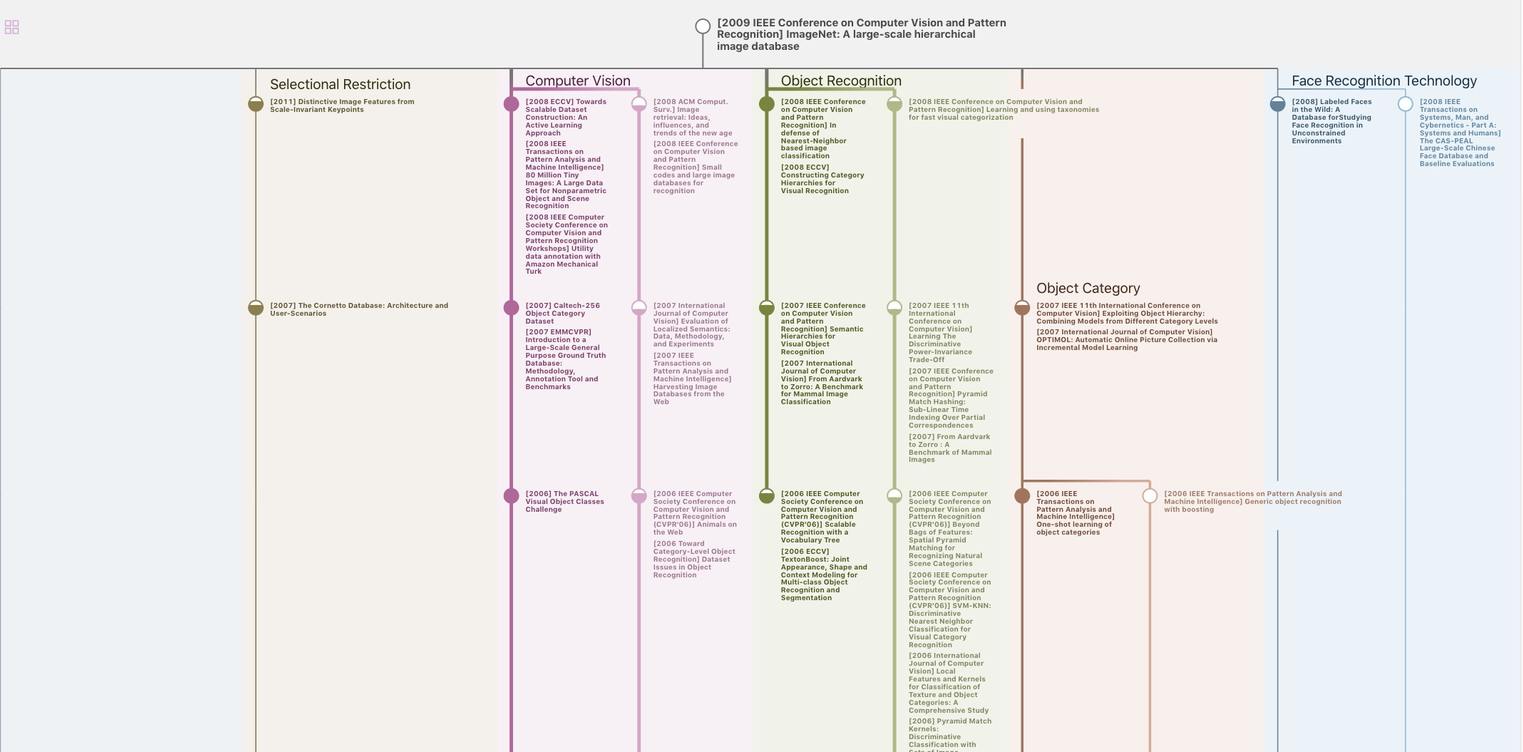
生成溯源树,研究论文发展脉络
Chat Paper
正在生成论文摘要