Educational Sensitive Information Retrieval: Analysis, Application, and Optimization.
IEEE ACCESS(2018)
摘要
Social networking and cloud services will collect a large number of high-dimensional and complex data for third party statistical analysis and data mining. Although data analysis is beneficial to users and external parties, they constitute a serious privacy risk disclosure of user sensitive information. First of all, the authors use random forest, decision tree, and support vector machines methods with a discriminant component model to analyze the data set of the new curriculum reform in Shaanxi Province and make an accurate prediction of the impact on bad habits of students' comprehensive performance. Second, though various kinds of data transformation for privacy protection have been achieved and applied, there is little research designing two preference classification tasks simultaneously. In order to prevent the privacy leakage of sensitive information in the data analysis, the data space projection algorithm based on multiclass discriminant is used to deal with the linear and nonlinear. Clearly, the role of sensitive task and insensitive task can be exchanged. The goal is to preserve the statistical properties of one preference classification as far as possible and to realize the data security of the other preference classification.
更多查看译文
关键词
Sensitive information,discriminant component,analysis information,extraction classification
AI 理解论文
溯源树
样例
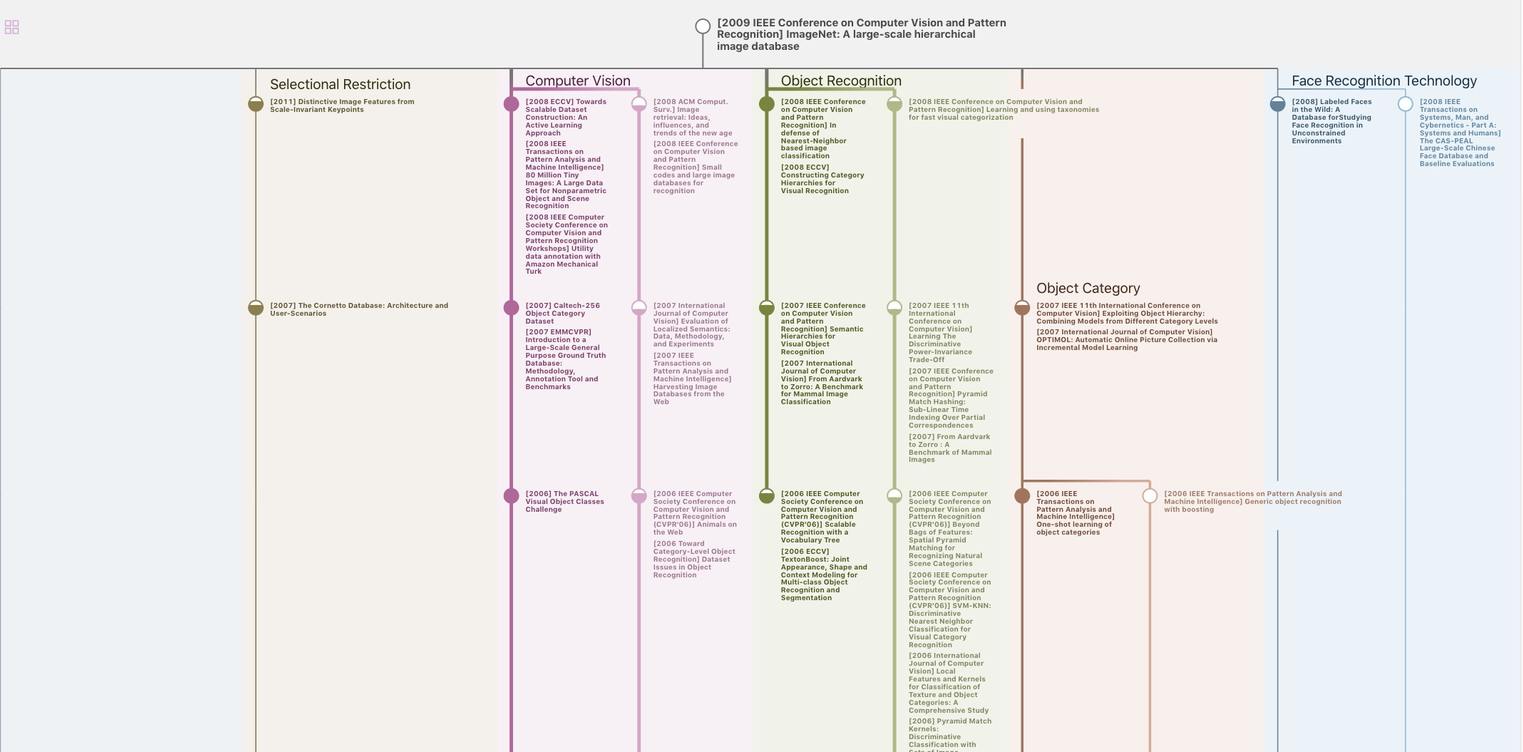
生成溯源树,研究论文发展脉络
Chat Paper
正在生成论文摘要