Classifying cardiac arrhythmic episodes via data compression.
Neurocomputing(2018)
摘要
The rapid development of the cloud computing technology is favoring the emergence of new platforms offering a new broad range of possibilities for data analytics. An example of this is SCOOP, a scientific cloud computing platform designed to massively collect data from Implantable Cardioverter Defibrillators (ICDs) generating cooperatively new knowledge in the cardiac electrophysiology field. In this work, we propose a new automatic and computationally fast methodology for classifying the cardiac arrhythmic episodes recorded by current ICDs into 8 and 3 arrhythmic categories (8 and 3-class schemes, respectively). Similarity among episodes is determined via data compression based techniques. Then, a statistical classifier is designed by using previous similarities as a kernel. Our proposal is characterized by: (1) requiring minimal signal preprocessing; (2) dealing with episodes of different duration with blank intervals; and (3) considering simultaneously heart activation events and signal waveform as its underlying clinical criterion. To avoid bias in the classification of new episodes, performance was evaluated without considering previous patient information on the training stage. A set of 6233 arrhythmic episodes from 599 patients extracted from the SCOOP platform were considered in this work. Test accuracy rates (and kappa coefficients) close to 78% (0.6) and 90% (0.8) were achieved in both 8 and 3-class schemes, respectively. This fact shows that our methodology can be used as a data analytics cardiology tool supporting physicians in their cardiac diagnosis, forecasting its future use in ICDs and related systems.
更多查看译文
关键词
Implantable Cardioverter Defibrillator,Cardiac arrhythmic episode,Automatic classification,Data compression,Kernel method
AI 理解论文
溯源树
样例
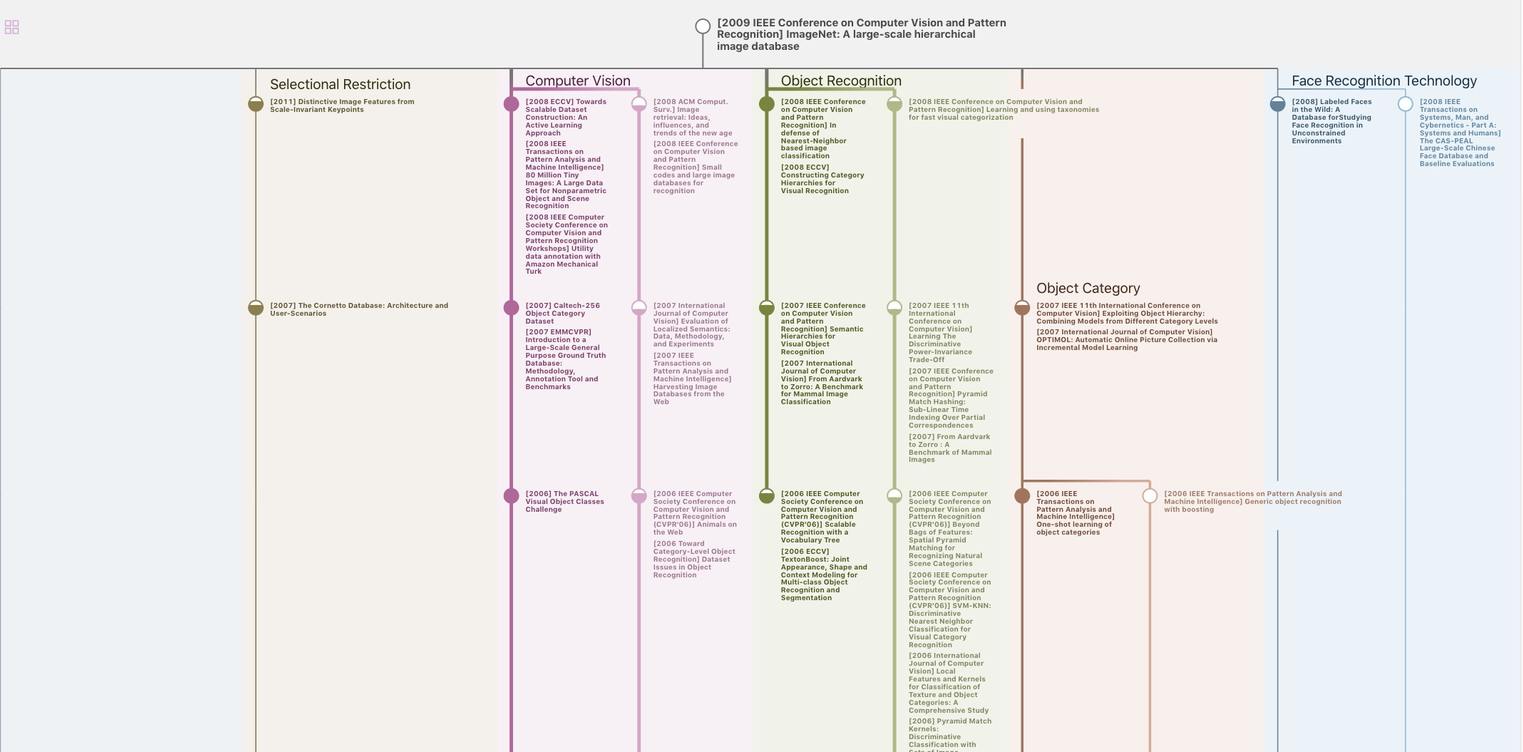
生成溯源树,研究论文发展脉络
Chat Paper
正在生成论文摘要