Heterogeneous subgraph features for information networks
GRADES/NDA@SIGMOD/PODS(2018)
摘要
Networks play an increasingly important role in modelling real-world systems due to their utility in representing complex connections. For predictive analyses, the engineering of node features in such networks is of fundamental importance to machine learning applications, where the lack of external information often introduces the need for features that are based purely on network topology. Existing feature extraction approaches have so far focused primarily on networks with just one type of node and thereby disregarded the information contained in the topology of heterogeneous networks, or used domain specific approaches that incorporate node labels based on external knowledge. Here, we generalize the notion of heterogeneity and present an approach for the efficient extraction and representation of heterogeneous subgraph features. We evaluate their performance for rank- and label-prediction tasks and explore the implications of feature importance for prominent subgraphs. Our experiments reveal that heterogeneous subgraph features reach the predictive power of manually engineered features that incorporate domain knowledge. Furthermore, we find that heterogeneous subgraph features outperform state-of-the-art neural node embeddings in both tasks and across all data sets.
更多查看译文
关键词
Heterogeneous networks, information networks, node features, feature engineering, graph encodings
AI 理解论文
溯源树
样例
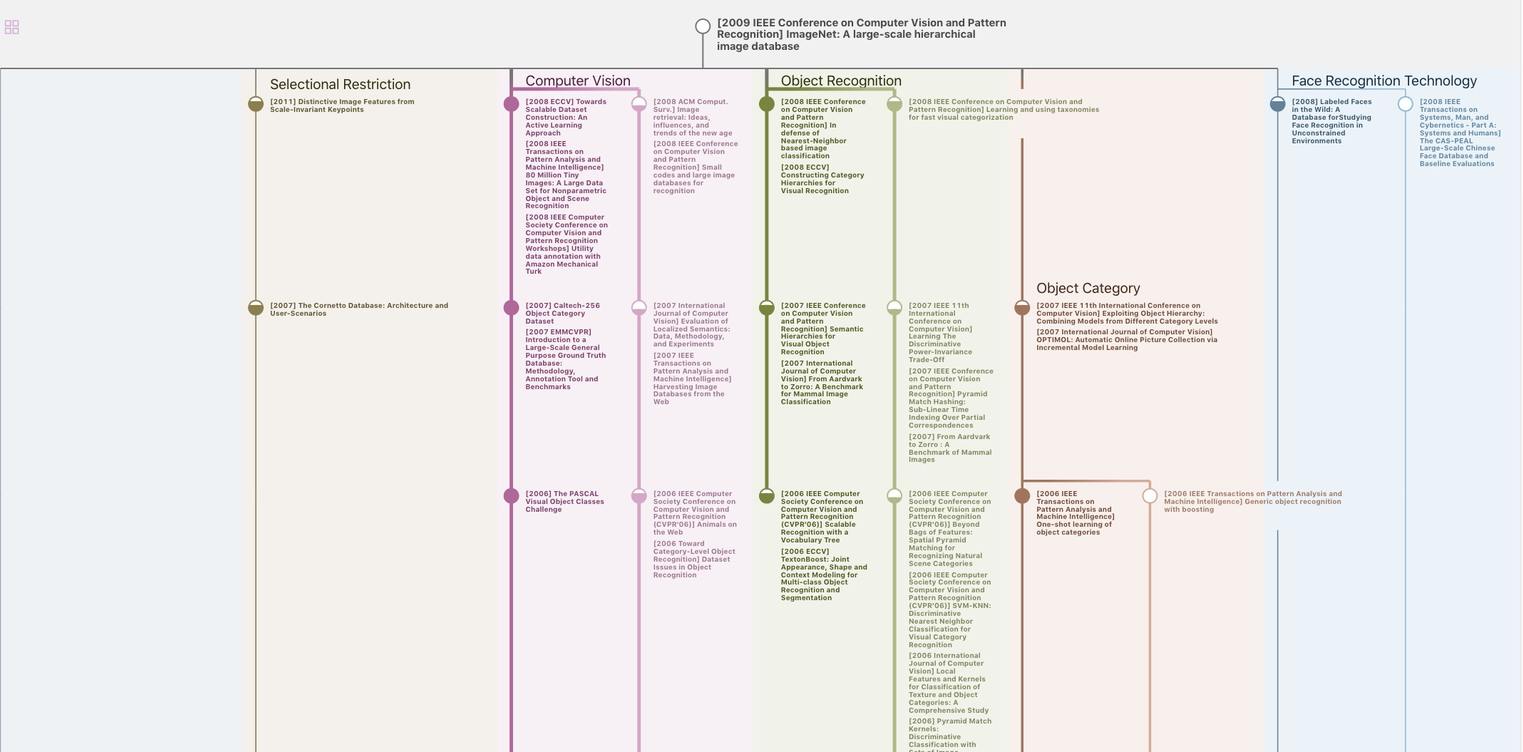
生成溯源树,研究论文发展脉络
Chat Paper
正在生成论文摘要