HFMV: hybridizing formal methods and machine learning for verification of analog and mixed-signal circuits
2018 55TH ACM/ESDA/IEEE DESIGN AUTOMATION CONFERENCE (DAC)(2018)
摘要
With increasing design complexity and robustness requirement, analog and mixed-signal (AMS) verification manifests itself as a key bottleneck. While formal methods and machine learning have been proposed for AMS verification, these two techniques suffer from their own limitations, with the former being specifically limited by scalability and the latter by the inherent uncertainty in learning-based models. We present a new direction in AMS verification by proposing a hybrid formal/machine-learning verification technique (HFMV) to combine the best of the two worlds. HFMV adds formalism on the top of a probabilistic learning model while providing a sense of coverage for extremely rare failure detection. HFMV intelligently and iteratively reduces uncertainty of the learning model by a proposed formally-guided active learning strategy and discovers potential rare failure regions in complex high-dimensional parameter spaces. It leads to reliable failure prediction in the case of a failing circuit, or a high-confidence pass decision in the case of a good circuit. We demonstrate that HFMV is able to employ a modest amount of data to identify hard-to-find rare failures which are completely missed by state-of-the-art sampling methods even with high volume sampling data.
更多查看译文
关键词
machine learning,AMS verification,learning-based models,hybrid formal/machine-learning verification technique,HFMV,probabilistic learning model,active learning strategy,formal methods,mixed-signal circuits
AI 理解论文
溯源树
样例
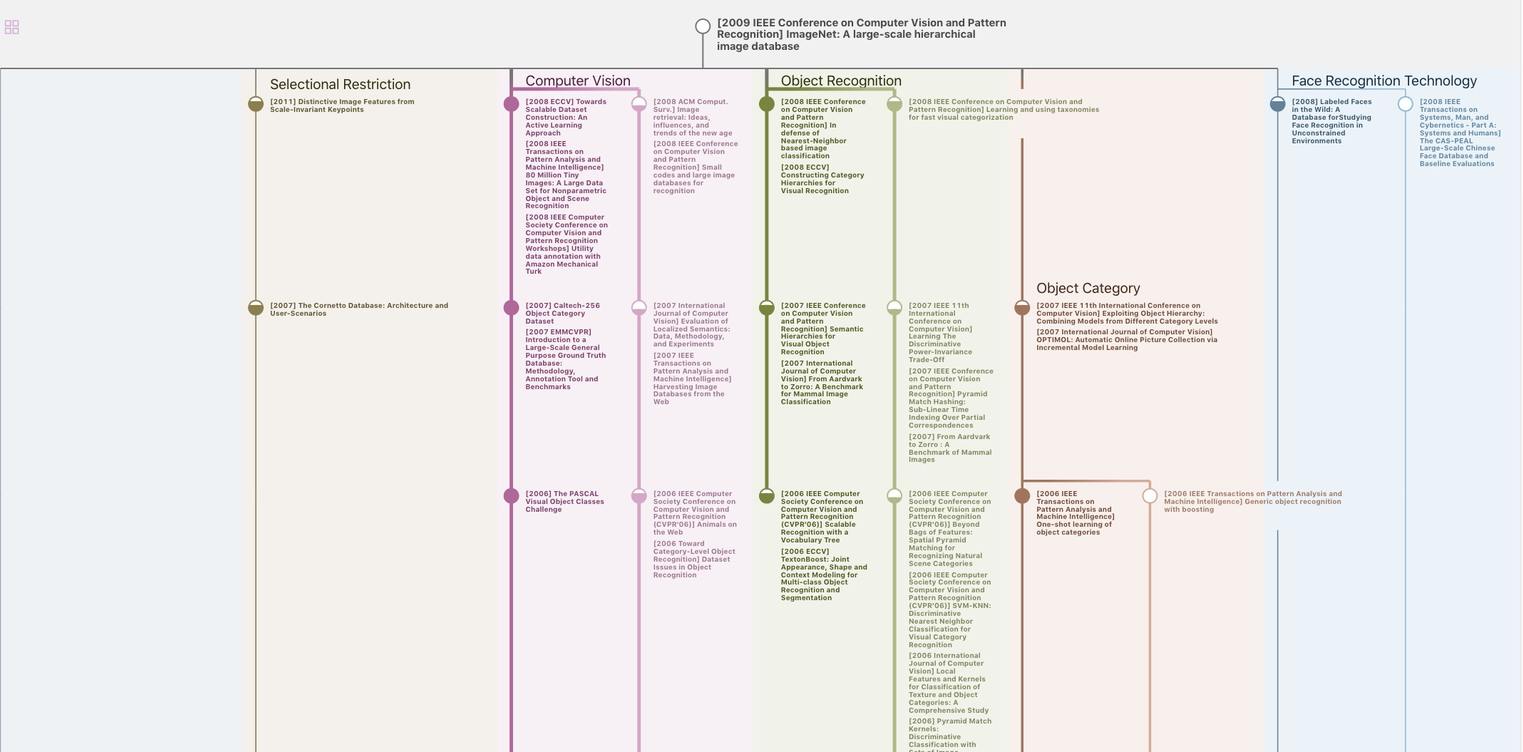
生成溯源树,研究论文发展脉络
Chat Paper
正在生成论文摘要