Decision Quality Enhancement in Minimum-Based Possibilistic Classification for Numerical Data
SoCPaR(2018)
摘要
Two Bayesian-like possibilistic classifiers based on the transformation of Dubois et al. in the continuous case have been proposed to deal with numerical data. For these two classifiers, namely Naive Possibilistic Classifier (NPC) and Flexible Naive Possibilistic Classifier (FNPC), the minimum operator has led to less accurate classification when compared to the one produced by the product rule. In this paper, we investigate the use of the Generalized Minimum-based (G-Min) algorithm that has been recently suggested as an alternative to the minimum operator for combining possibilistic estimates. The main objective is to enhance the quality of decision within minimum-based possibilistic classifiers for numerical data. Experimental evaluations are conducted on 15 numerical datasets taken from University of California Irvine (UCI) and show that using the G-Min algorithm largely improves the classification accuracy within minimum-based NPC as well as minimum-based FNPC.
更多查看译文
关键词
Naive Possibilistic Classifier,Flexible Naive Possibilistic Classifier,Minimum operator,G-Min algorithm,Numerical data
AI 理解论文
溯源树
样例
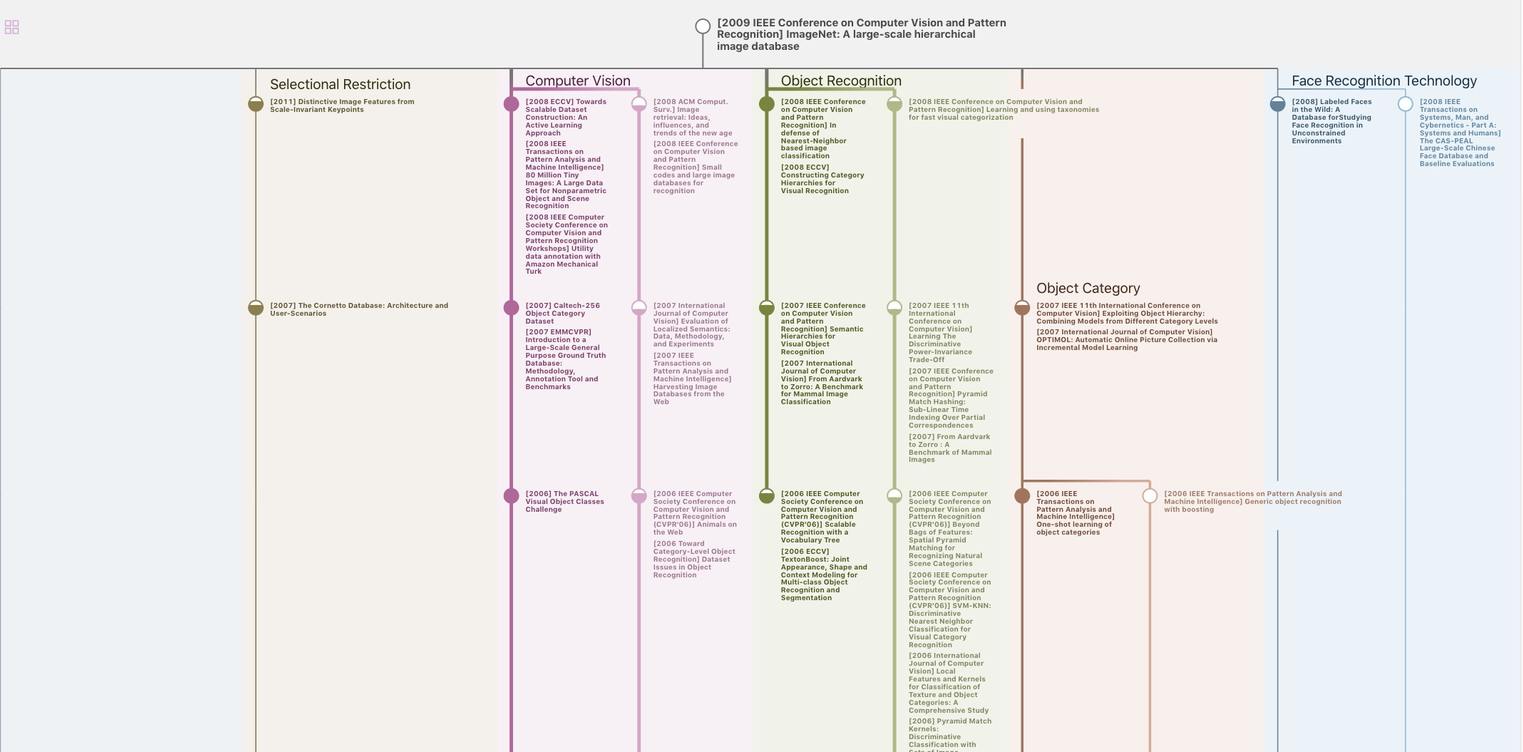
生成溯源树,研究论文发展脉络
Chat Paper
正在生成论文摘要