A Kernelized Morphable Model For 3d Brain Tumor Analysis
IMAGE ANALYSIS AND RECOGNITION (ICIAR 2018)(2018)
摘要
Abnormal tissue analysis in brain volumes is a difficult task, due to the shape variability that the brain tumors exhibit between patients. The main problem in these processes is that the common techniques use linear representations of the input data which makes unsuitable to model complex shapes as brain tumors. In this paper, we present a kernelized morphable model (3D-KMM) for brain tumor analysis in which the model variations are captured through nonlinear mappings by using kernel principal component analysis. We learn complex shape variations through a high-dimensional representation of the input data. Then from the trained model, we recover the pre-images from the features vectors and perform a non-rigid matching procedure to fit the modeled tumor to a given brain volume. The results show that by using a kernelized morphable model, the non-rigid properties (i.e., nonlinearities and shape variations) of the abnormal tissues can be learned. Finally, our approach proves to be more accurate than the classic morphable model for shape analysis.
更多查看译文
关键词
Brain tumor analysis, Kernelized morphable model, 3D Shape fitting
AI 理解论文
溯源树
样例
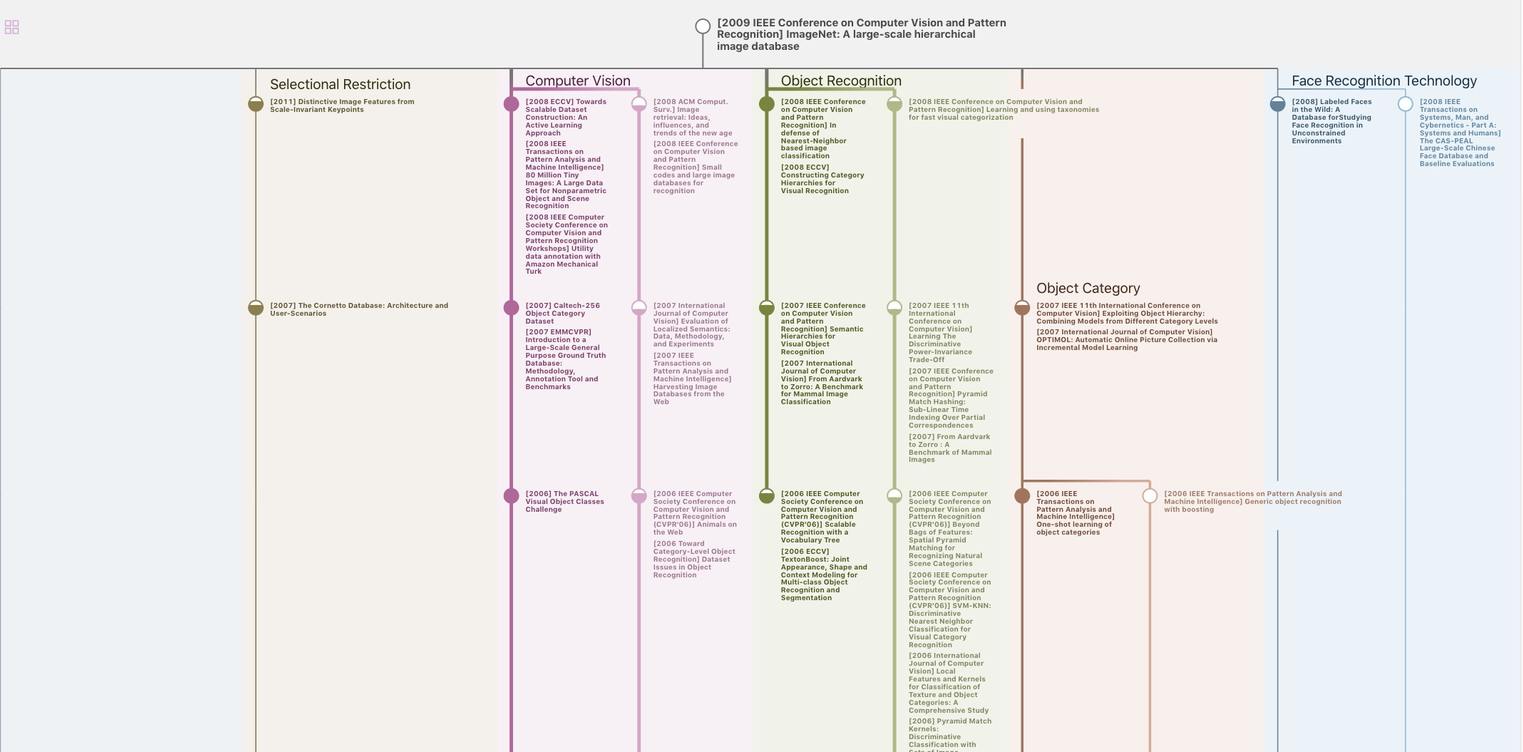
生成溯源树,研究论文发展脉络
Chat Paper
正在生成论文摘要