Modeling Evolution of Topics in Large-Scale Temporal Text Corpora.
ICWSM(2018)
摘要
Large text temporal collections provide insights into social and cultural change over time. To quantify changes in topics in these corpora, embedding methods have been used as a diachronic tool. However, they have limited utility for modeling changes in topics due to the stochastic nature of training. We propose a new computational approach for tracking and detecting temporal evolution of topics in a large collection of texts. This approach for identifying dynamic topics and modeling their evolution combines the advantages of two methods: (1) word embeddings to learn contextual semantic representation of words from temporal snapshots of the data and (2) dynamic network analysis to identify dynamic topics by using dynamic semantic similarity networks developed using embedding models. Experimenting with two large temporal data sets from the legal and real estate domains, we show that this approach performs faster (due to parallelizing different snapshots), uncovers more coherent topics (compared to available dynamic topic modeling approaches), and effectively enables modeling evolution leveraging the network structure.
更多查看译文
AI 理解论文
溯源树
样例
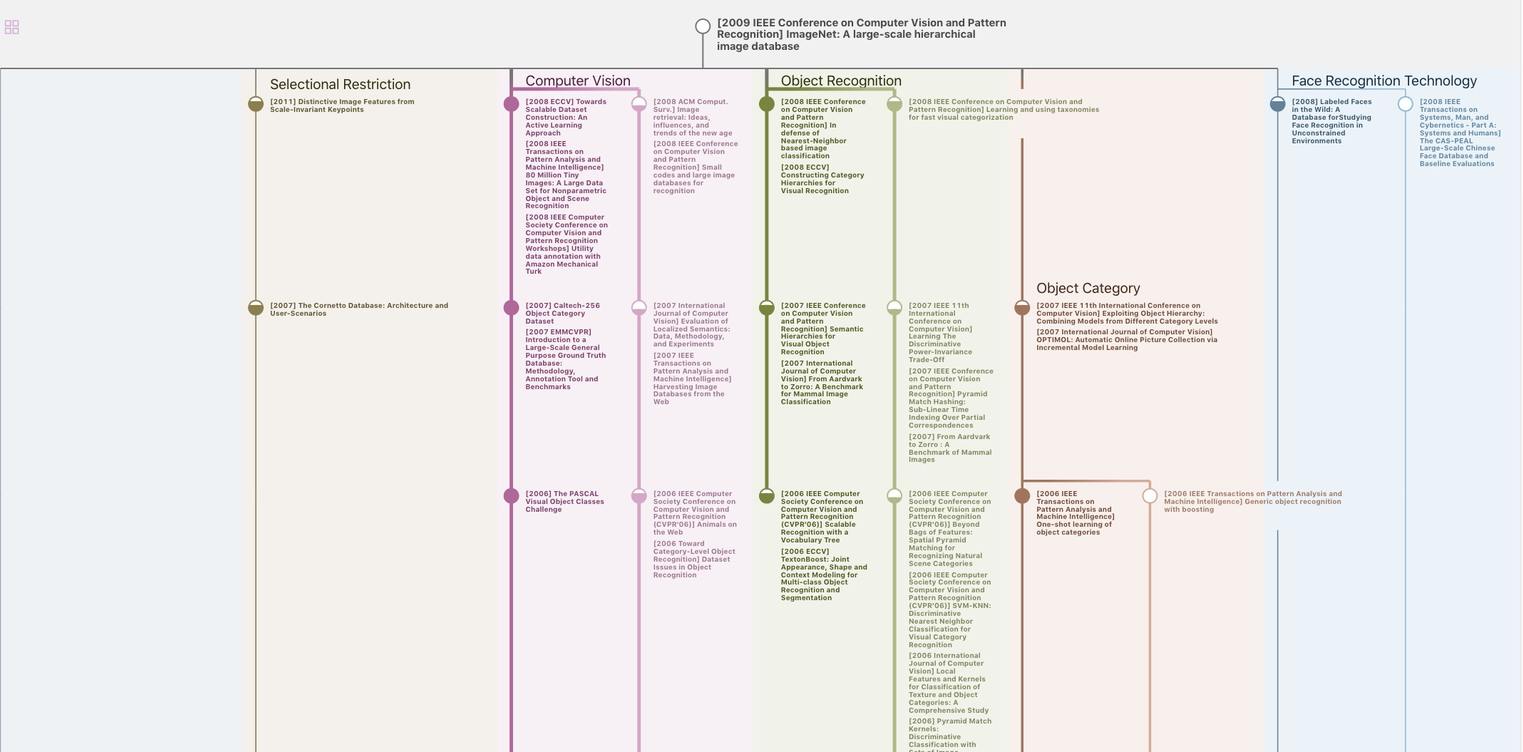
生成溯源树,研究论文发展脉络
Chat Paper
正在生成论文摘要