Detecting Hypopnea and Obstructive Apnea Events Using Convolutional Neural Networks on Wavelet Spectrograms of Nasal Airflow.
ADVANCES IN KNOWLEDGE DISCOVERY AND DATA MINING, PAKDD 2018, PT I(2018)
摘要
We present a novel approach for detecting hypopnea and obstructive apnea events during sleep, using a single channel nasal airflow from polysomnography recordings, applying a Convolutional Neural Network (CNN) to a 2-D image wavelet spectrogram of the nasal signal. We compare this approach to directly training a 1-D CNN on the raw nasal airflow signal. The evaluation was conducted on a large dataset consisting of 69,264 examples from 1,507 subjects. Our results showed that both approaches achieved good accuracy, with the 2-D CNN outperforming the 1-D CNN. The higher accuracy and the less complex architecture of the 2-D CNN show that converting biological signals into spectrograms and using them in conjunction with CNNs is a promising method for sleep apnea recognition.
更多查看译文
关键词
Sleep disorder,Obstructive Sleep Apnea,Convolutional Neural Networks,Wavelets,Supervised learning
AI 理解论文
溯源树
样例
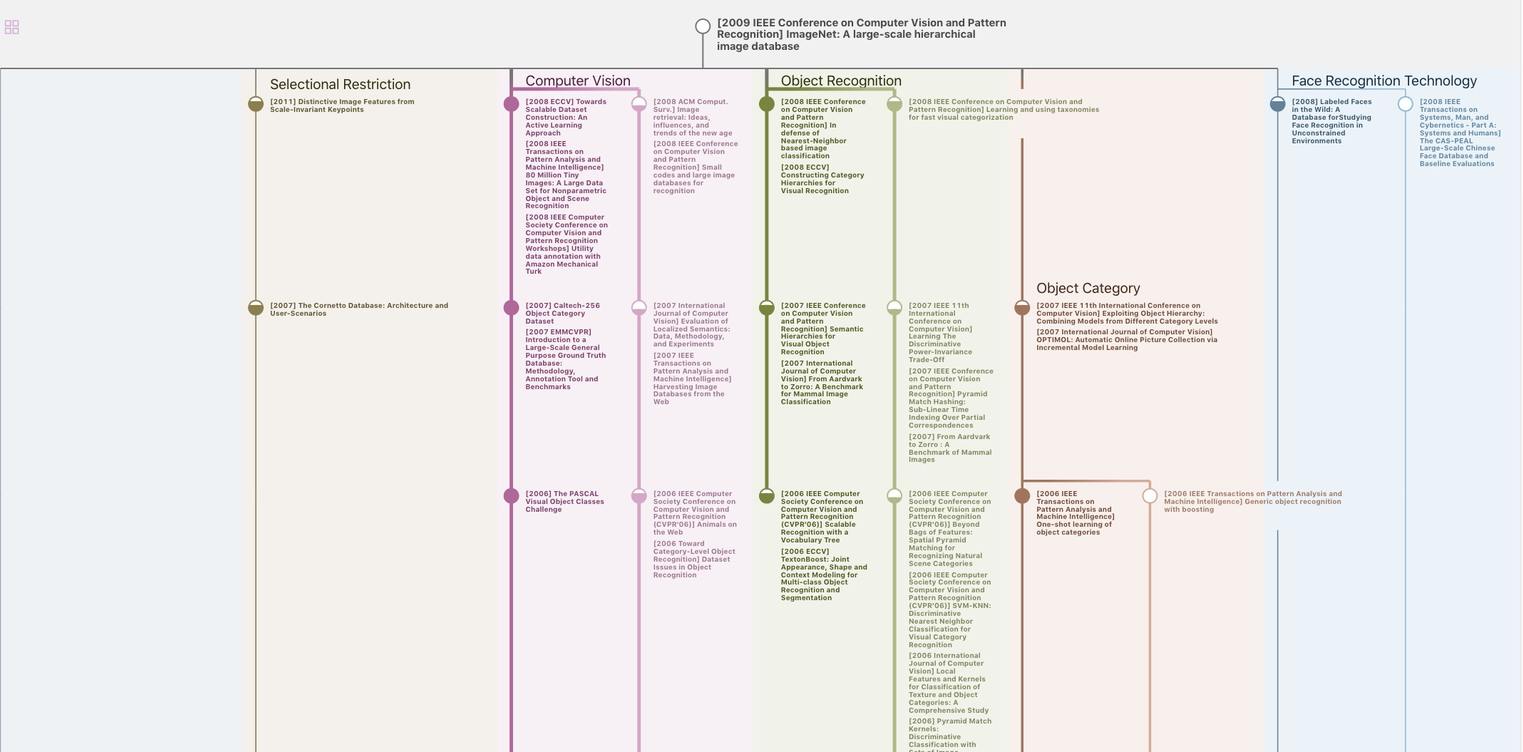
生成溯源树,研究论文发展脉络
Chat Paper
正在生成论文摘要