Reducing Incarceration Through Prioritized Interventions
PROCEEDINGS OF THE 1ST ACM SIGCAS CONFERENCE ON COMPUTING AND SUSTAINABLE SOCIETIES (COMPASS 2018)(2018)
摘要
The most vulnerable individuals in society often struggle with long-lasting, multi-faceted challenges like mental illness, substance abuse, chronic health conditions, and homelessness. Individuals experiencing these difficulties tend to interact with public services and departments frequently, but many communities are struggling to identify those individuals, let alone meet their needs in meaningful and cost-effective ways. In this paper, we describe our work with Johnson County, Kansas, that uses machine learning to prioritize outreach to individuals most at risk of being booked into jail within the next year. For the first time, we brought together Johnson County's jail, emergency medical, and mental health data, identified individuals who touch multiple systems, and built a model to predict individual jail bookings. Our system significantly outperformed both a random baseline and several simple heuristics that domain experts are likely to use and implement. By focusing on 200 individuals (which is the intervention capacity of Johnson County) who had interacted with both mental health services and the criminal justice system, we predicted jail bookings in the following year with 51% precision, which outperforms a baseline heuristic model by 1.5 times, and is 4.6 times better than a random baseline. This work provides a framework and prototype system for Johnson County as well as many other jurisdictions that are part of the Data Driven Justice Initiative as they develop intervention models to proactively connect social and mental health workers with individuals in need of care to avoid incarceration.
更多查看译文
关键词
frequent utilizers, criminal justice system, machine learning, predictive intervention system
AI 理解论文
溯源树
样例
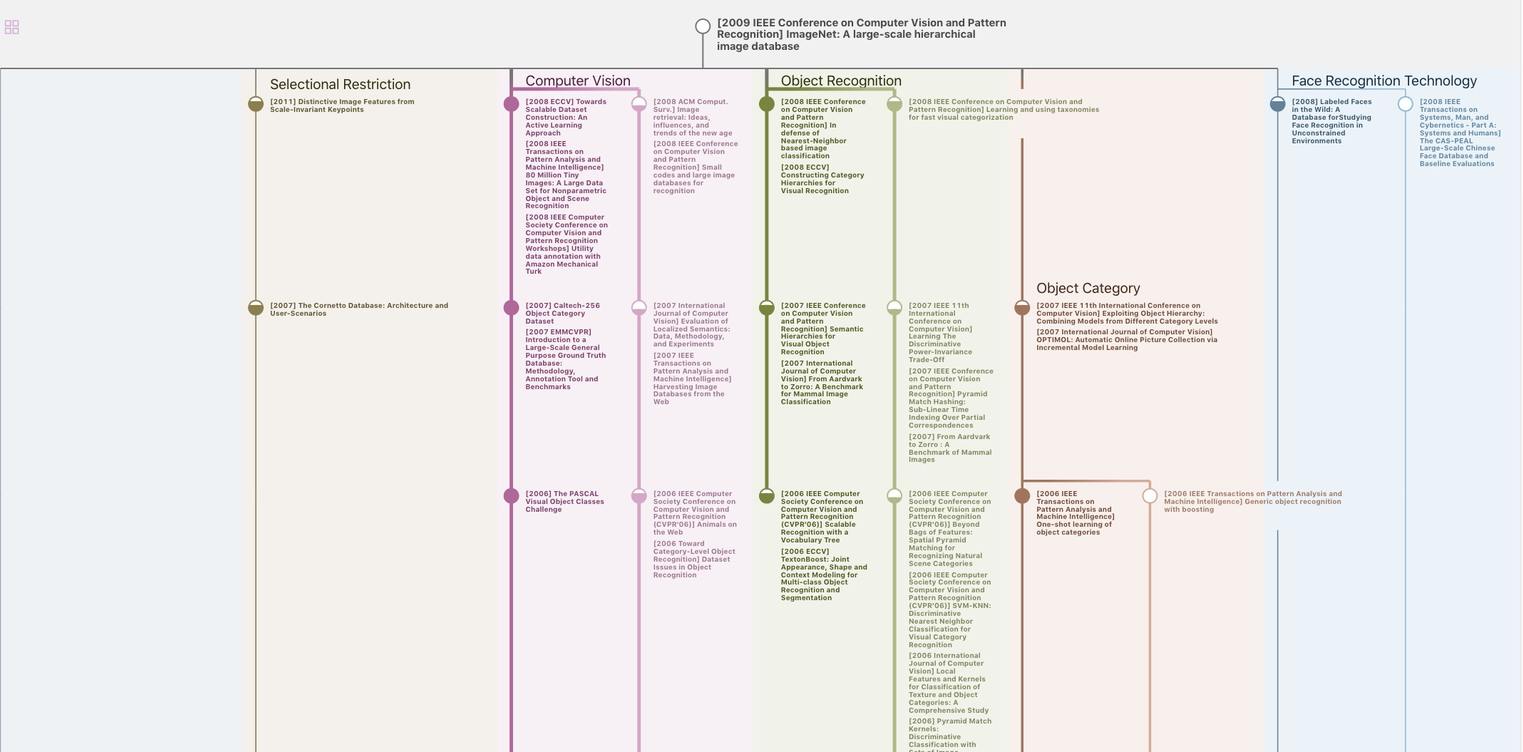
生成溯源树,研究论文发展脉络
Chat Paper
正在生成论文摘要