Distribution-free junta testing.
STOC(2019)
摘要
We study the problem of testing whether an unknown n-variable Boolean function is a k-junta in the distribution-free property testing model, where the distance between functions is measured with respect to an arbitrary and unknown probability distribution over {0,1}n. Our first main result is that distribution-free k-junta testing can be performed, with one-sided error, by an adaptive algorithm that uses Õ(k2)/ε queries (independent of n). Complementing this, our second main result is a lower bound showing that any non-adaptive distribution-free k-junta testing algorithm must make Ω(2k/3) queries even to test to accuracy ε = 1/3. These bounds establish that while the optimal query complexity of non-adaptive k-junta testing is 2Θ(k), for adaptive testing it is poly(k), and thus show that adaptivity provides an exponential improvement in the distribution-free query complexity of testing juntas.
更多查看译文
关键词
Property testing, distribution-free model
AI 理解论文
溯源树
样例
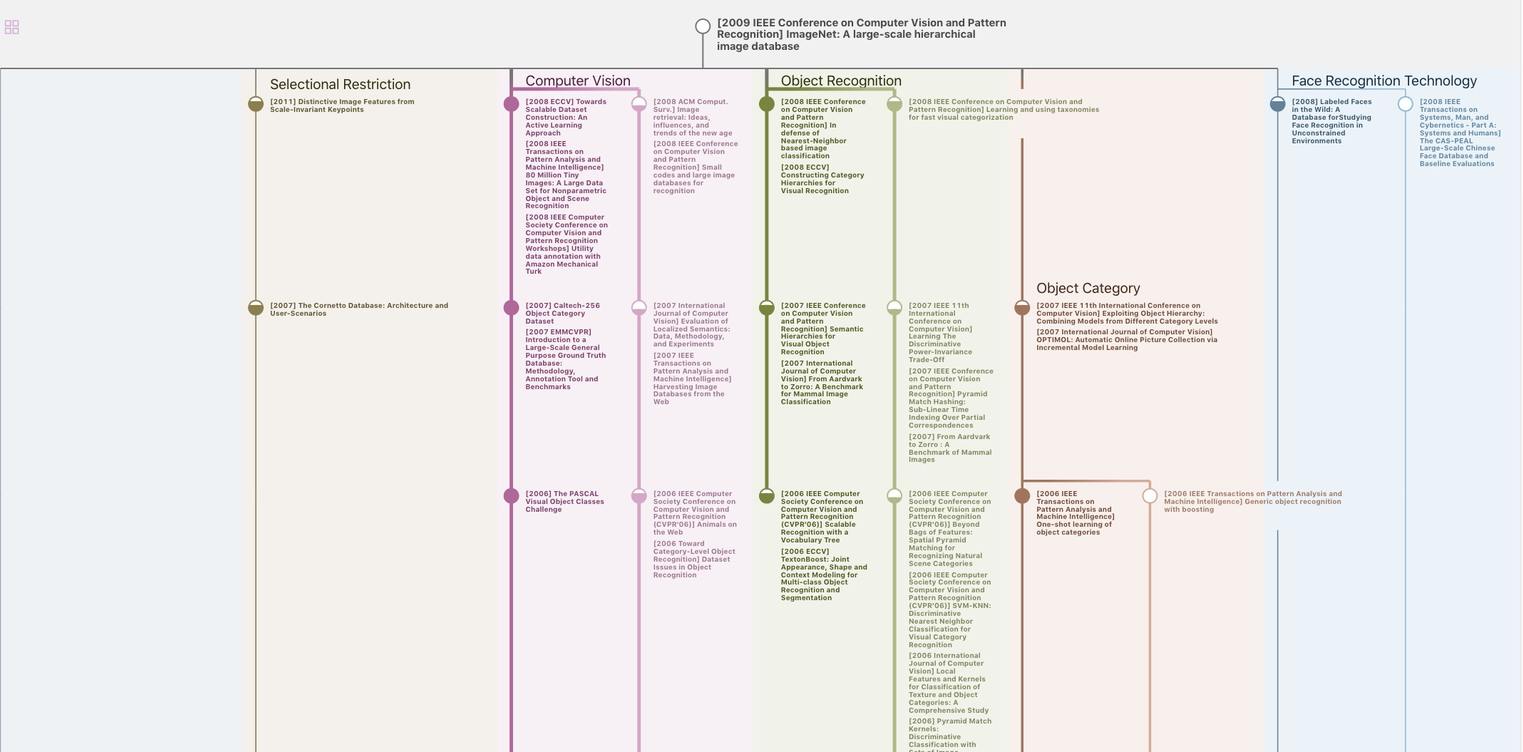
生成溯源树,研究论文发展脉络
Chat Paper
正在生成论文摘要