Dcs-Based Mbsbl Joint Reconstruction Of Multi-Sensors Data For Energy-Efficient Telemonitoring Of Human Activity
INTERNATIONAL JOURNAL OF DISTRIBUTED SENSOR NETWORKS(2018)
摘要
The joint reconstruction of nonsparse multi-sensors data with high quality is a challenging issue in human activity telemonitoring. In this study, we proposed a novel joint reconstruction algorithm combining distributed compressed sensing with multiple block sparse Bayesian learning. Its basic idea is that based on the joint sparsity model, the distributed compressed sensing technique is first applied to simultaneously compress the multi-sensors data for gaining the high-correlation information regarding activity as well as the energy efficiency of sensors, and then, the multiple block sparse Bayesian learning technique is employed to jointly recover nonsparse multi-sensors data with high fidelity by exploiting the joint block sparsity. The multi-sensors acceleration data from an open wearable action recognition database are selected to assess the practicality of our proposed technique. The sparse representation classification model is used to classify activity patterns using the jointly reconstructed data in order to further examine the effectiveness of our proposed method. The results showed that when compression rates are selected properly, our proposed technique can gain the best joint reconstruction performance as well as energy efficiency of sensors, which greatly contributes to the best sparse representation classification-based activity classification performance. This has a great potential for energy-efficient telemonitoring of human activity.
更多查看译文
关键词
Distributed compressed sensing, block sparse Bayesian learning, joint reconstruction, telemonitoring of human activity
AI 理解论文
溯源树
样例
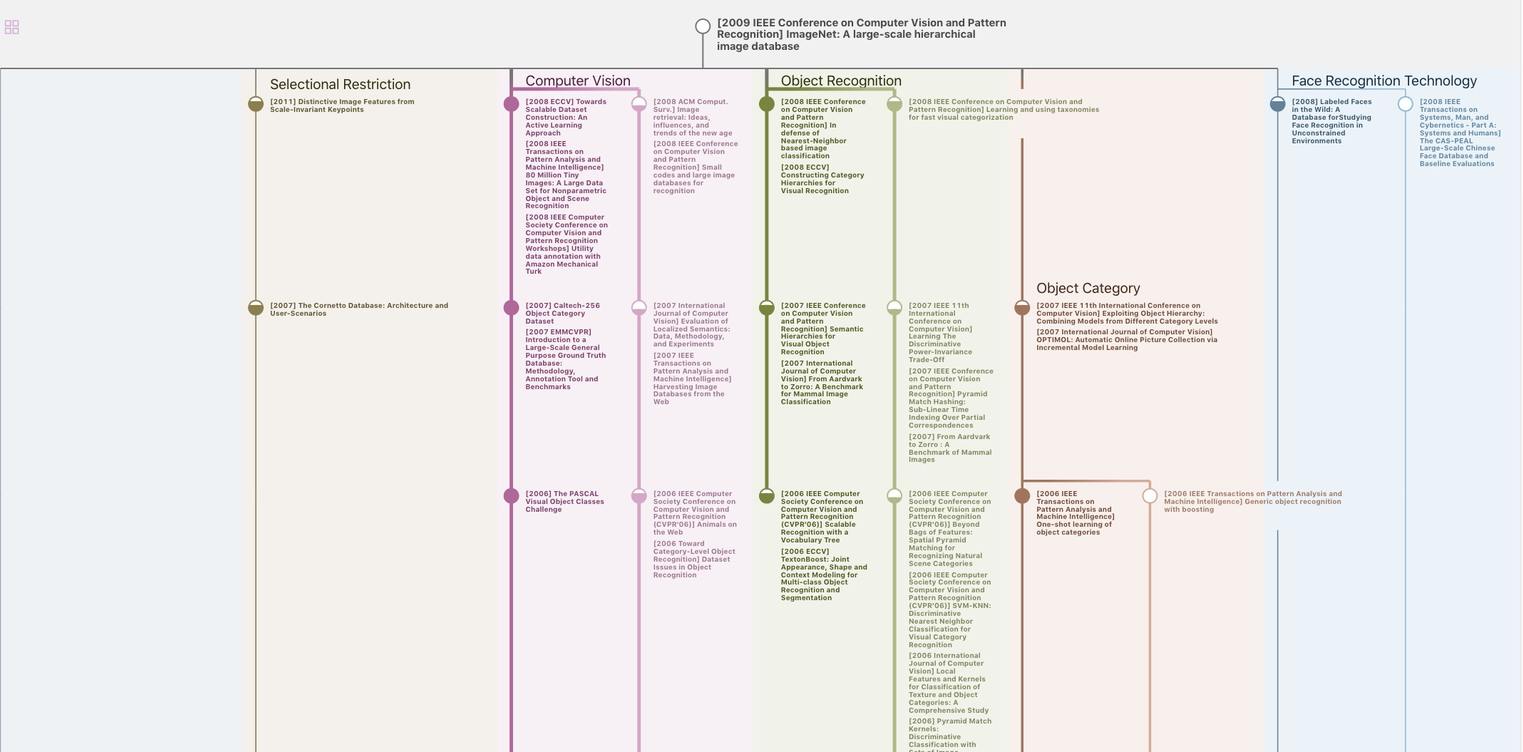
生成溯源树,研究论文发展脉络
Chat Paper
正在生成论文摘要