Defo-Net: Learning Body Deformation using Generative Adversarial Networks
2018 IEEE International Conference on Robotics and Automation (ICRA)(2018)
摘要
Modelling the physical properties of everyday objects is a fundamental prerequisite for autonomous robots. We present a novel generative adversarial network (Defo-Net), able to predict body deformations under external forces from a single RGB-D image. The network is based on an invertible conditional Generative Adversarial Network (IcGAN) and is trained on a collection of different objects of interest generated by a physical finite element model simulator. Defo-Net inherits the generalisation properties of GANs. This means that the network is able to reconstruct the whole 3-D appearance of the object given a single depth view of the object and to generalise to unseen object configurations. Contrary to traditional finite element methods, our approach is fast enough to be used in real-time applications. We apply the network to the problem of safe and fast navigation of mobile robots carrying payloads over different obstacles and floor materials. Experimental results in real scenarios show how a robot equipped with an RGB-D camera can use the network to predict terrain deformations under different payload configurations and use this to avoid unsafe areas.
更多查看译文
关键词
RGB-D image,finite element methods,mobile robots,single depth view,physical finite element model simulator,autonomous robots,Generative Adversarial networks,body deformation,DEFO-NET
AI 理解论文
溯源树
样例
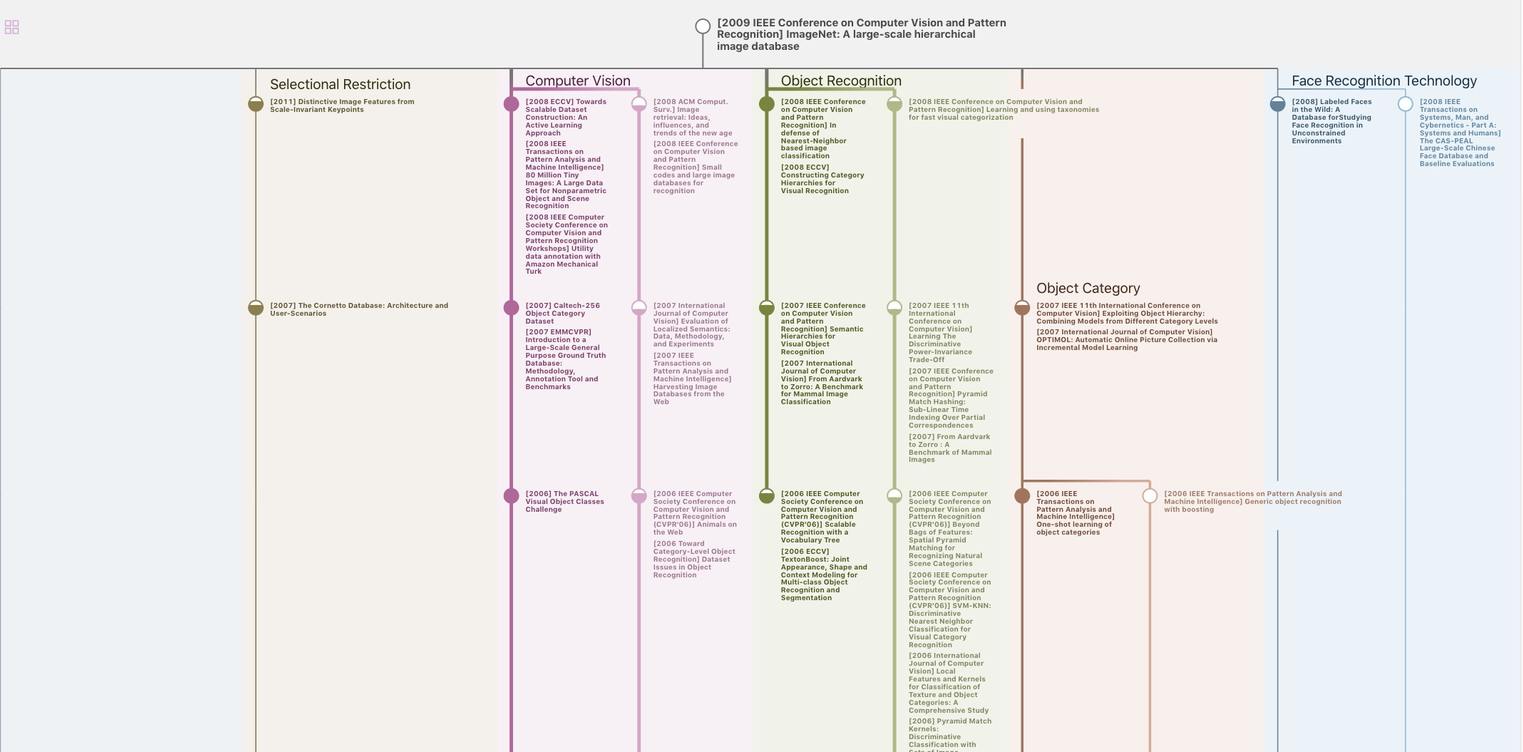
生成溯源树,研究论文发展脉络
Chat Paper
正在生成论文摘要