Global SNR Estimation of Speech Signals using Entropy and Uncertainty Estimates from Dropout Networks
19TH ANNUAL CONFERENCE OF THE INTERNATIONAL SPEECH COMMUNICATION ASSOCIATION (INTERSPEECH 2018), VOLS 1-6: SPEECH RESEARCH FOR EMERGING MARKETS IN MULTILINGUAL SOCIETIES(2018)
摘要
This paper demonstrates two novel methods to estimate the global SNR of speech signals. In both methods, Deep Neural Network-Hidden Markov Model (DNN-HMM) acoustic model used in speech recognition systems is leveraged for the additional task of SNR estimation. In the first method, the entropy of the DNN-HMM output is computed. Recent work on bayesian deep learning has shown that a DNN-HMM trained with dropout can be used to estimate model uncertainty by approximating it as a deep Gaussian process. In the second method, this approximation is used to obtain model uncertainty estimates. Noise specific regressors are used to predict the SNR from the entropy and model uncertainty. The DNN-HMM is trained on GRID corpus and tested on different noise profiles from the DEMAND noise database at SNR levels ranging from -10 dB to 30 dB.
更多查看译文
关键词
SNR estimation, Entropy, Bayesian Uncertainty, Dropout, Neural Networks
AI 理解论文
溯源树
样例
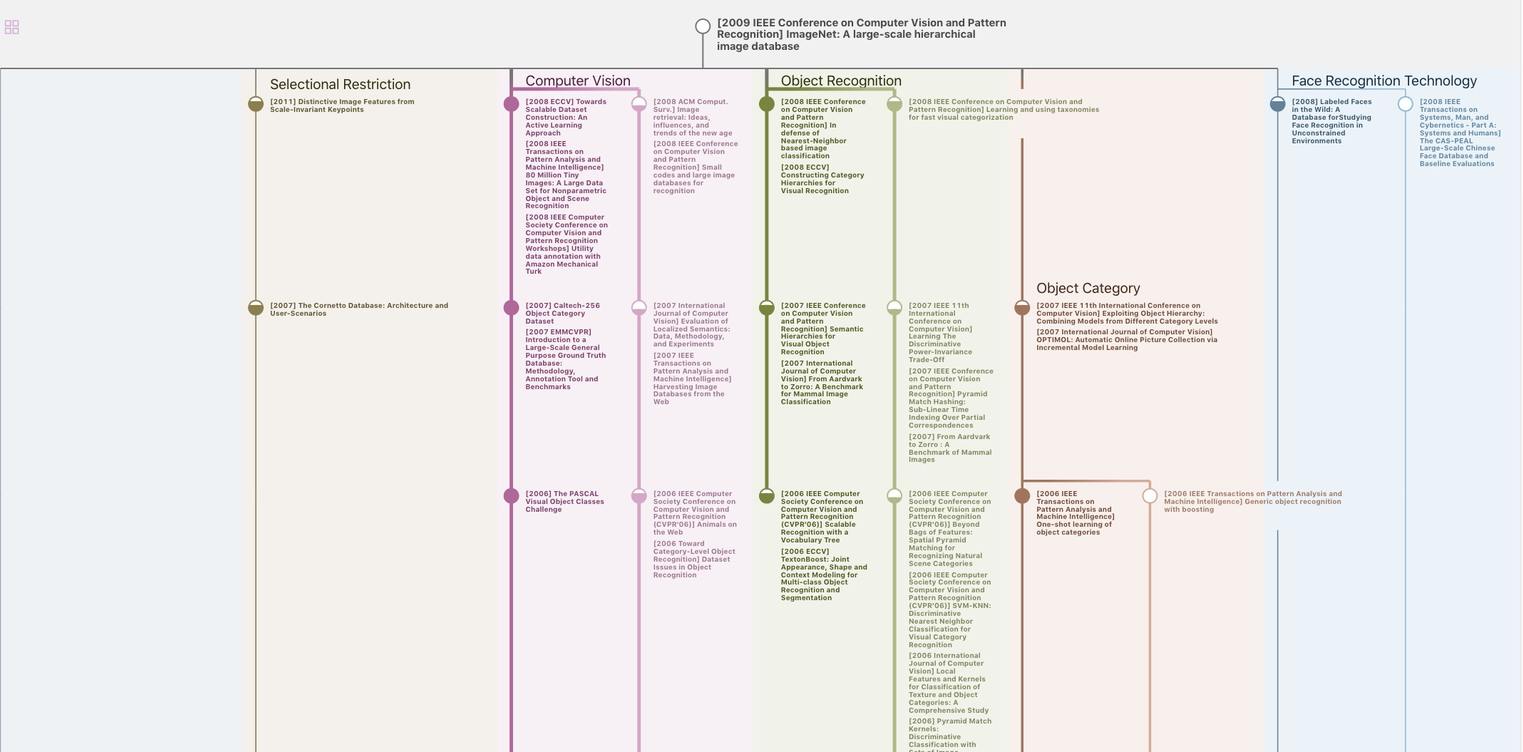
生成溯源树,研究论文发展脉络
Chat Paper
正在生成论文摘要