From Credit Assignment to Entropy Regularization: Two New Algorithms for Neural Sequence Prediction
PROCEEDINGS OF THE 56TH ANNUAL MEETING OF THE ASSOCIATION FOR COMPUTATIONAL LINGUISTICS (ACL), VOL 1(2018)
摘要
In this work, we study the credit assignment problem in reward augmented maximum likelihood (RAML) learning, and establish a theoretical equivalence between the token-level counterpart of RAML and the entropy regularized reinforcement learning. Inspired by the connection, we propose two sequence prediction algorithms, one extending RAML with fine-grained credit assignment and the other improving Actor-Critic with a systematic entropy regularization. On two benchmark datasets, we show the proposed algorithms outperform RAML and Actor-Critic respectively, providing new alternatives to sequence prediction.
更多查看译文
AI 理解论文
溯源树
样例
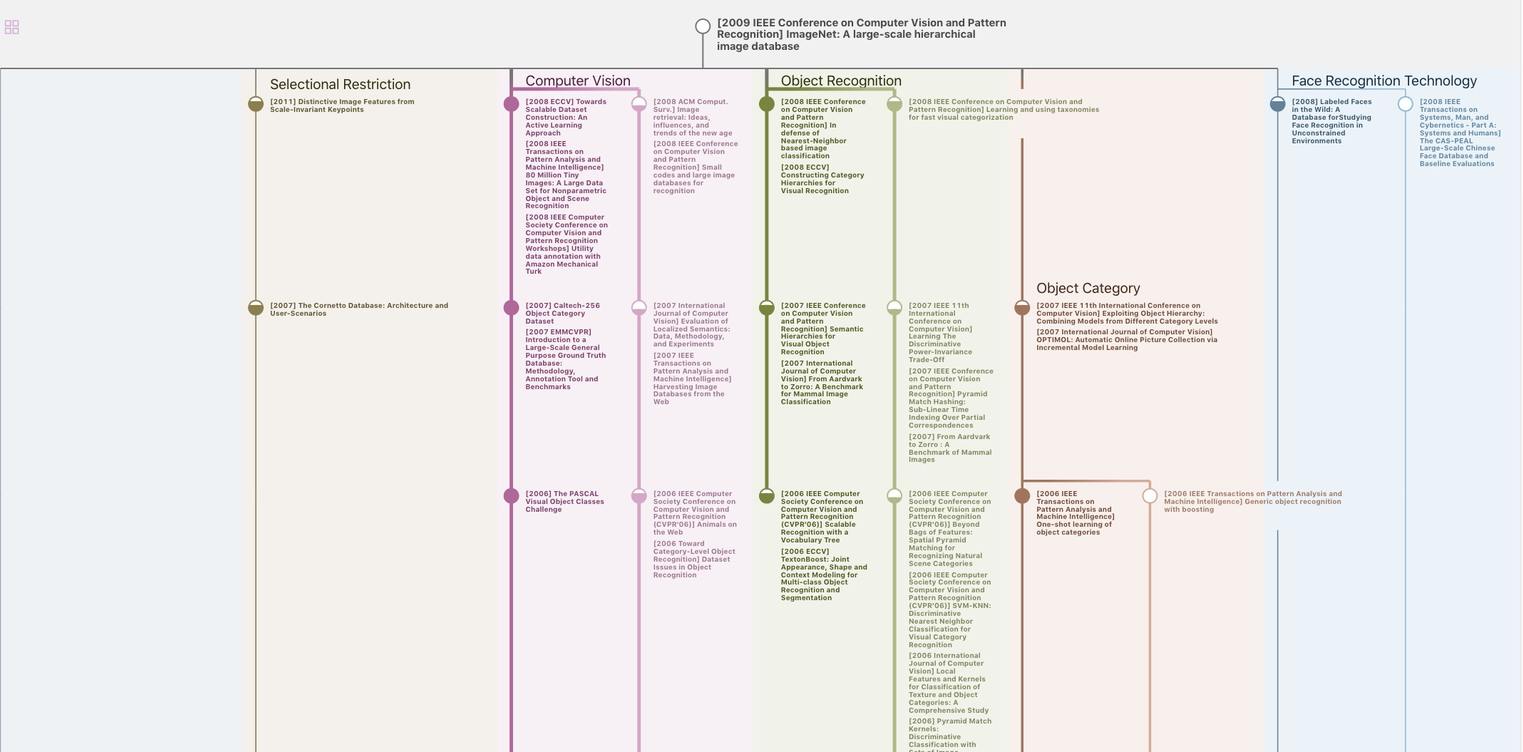
生成溯源树,研究论文发展脉络
Chat Paper
正在生成论文摘要