VectorDefense: Vectorization as a Defense to Adversarial Examples.
arXiv: Computer Vision and Pattern Recognition(2018)
摘要
Training deep neural networks on images represented as grids of pixels has brought to light an interesting phenomenon known as adversarial examples. Inspired by how humans reconstruct abstract concepts, we attempt to codify the input bitmap image into a set of compact, interpretable elements to avoid being fooled by the adversarial structures. We take the first step in this direction by experimenting with image vectorization as an input transformation step to map the adversarial examples back into the natural manifold of MNIST handwritten digits. We compare our method vs. state-of-the-art input transformations and further discuss the trade-offs between a hand-designed and a learned transformation defense.
更多查看译文
关键词
vectorization,vectordefense,examples
AI 理解论文
溯源树
样例
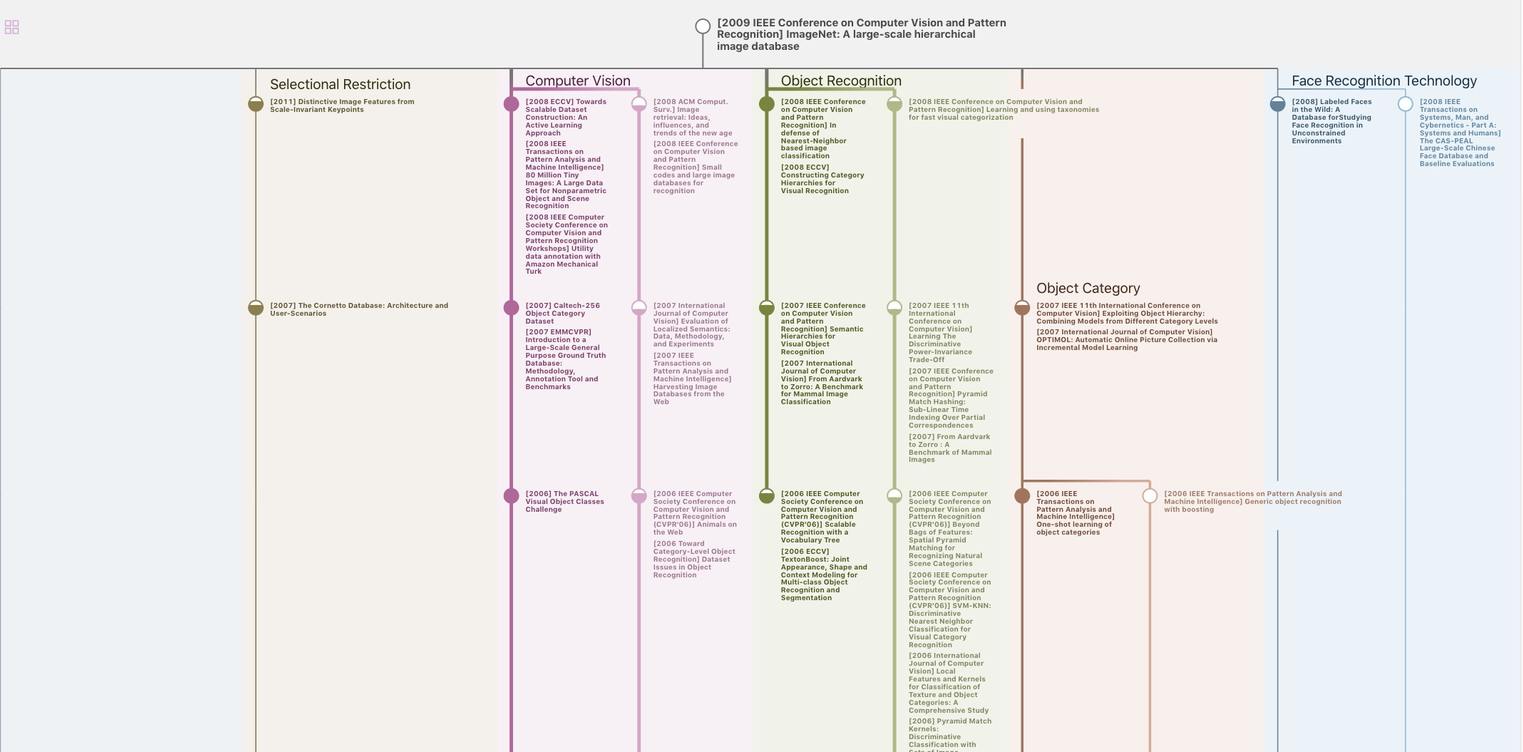
生成溯源树,研究论文发展脉络
Chat Paper
正在生成论文摘要