Weighted Low-Rank Approximation of Matrices and Background Modeling
arXiv (Cornell University)(2018)
摘要
We primarily study a special a weighted low-rank approximation of matrices and then apply it to solve the background modeling problem. We propose two algorithms for this purpose: one operates in the batch mode on the entire data and the other one operates in the batch-incremental mode on the data and naturally captures more background variations and computationally more effective. Moreover, we propose a robust technique that learns the background frame indices from the data and does not require any training frames. We demonstrate through extensive experiments that by inserting a simple weight in the Frobenius norm, it can be made robust to the outliers similar to the $\ell_1$ norm. Our methods match or outperform several state-of-the-art online and batch background modeling methods in virtually all quantitative and qualitative measures.
更多查看译文
关键词
background,matrices,modeling,low-rank
AI 理解论文
溯源树
样例
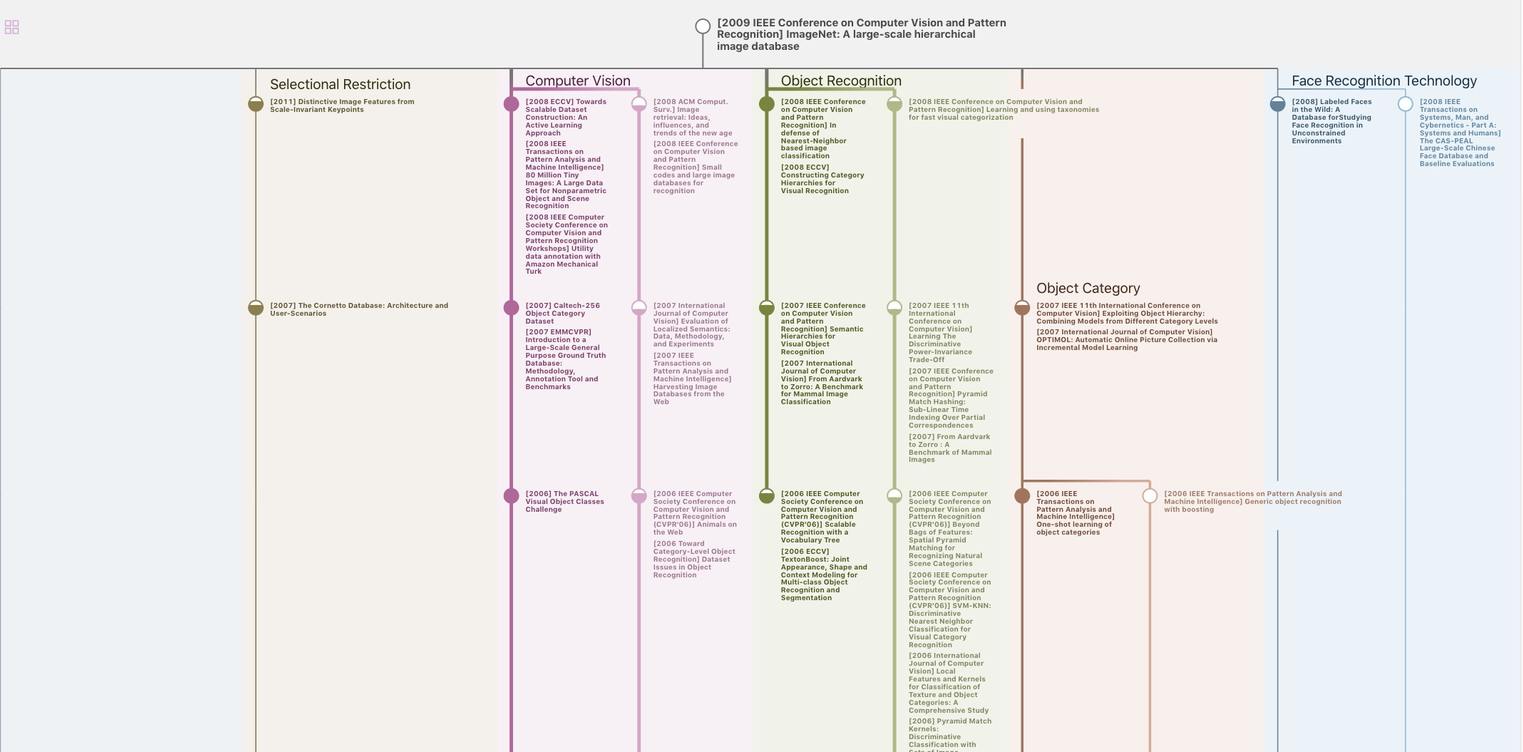
生成溯源树,研究论文发展脉络
Chat Paper
正在生成论文摘要