Algorithmic Identification Of Probabilities Is Hard
JOURNAL OF COMPUTER AND SYSTEM SCIENCES(2018)
摘要
Reading more and more bits from an infinite binary sequence that is random for a Bernoulli measure with parameter p, we can get better and better approximations of p using the strong law of large numbers. In this paper, we study a similar situation from the viewpoint of inductive inference. Assume that p is a computable real, and we have to eventually guess the program that computes p. We show that this cannot be done computably, and extend this result to more general computable distributions. We also provide a weak positive result showing that looking at a sequence X generated according to some computable probability measure, we can guess a sequence of algorithms that, starting from some point, compute a measure that makes X Martin-Lof random. (C) 2018 Elsevier Inc. All rights reserved.
更多查看译文
关键词
Algorithmic learning theory,Algorithmic randomness
AI 理解论文
溯源树
样例
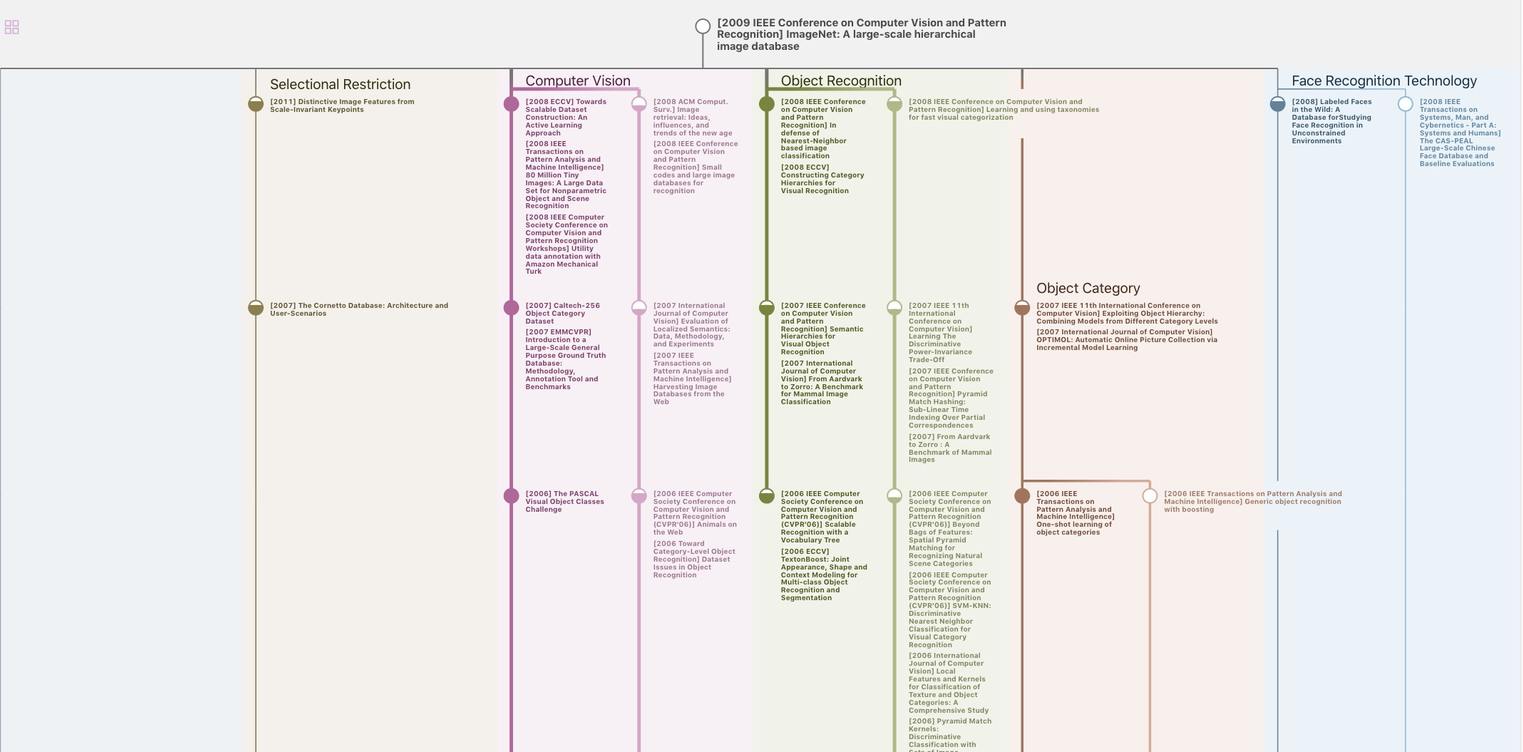
生成溯源树,研究论文发展脉络
Chat Paper
正在生成论文摘要