High Resolution Similarity Directed Adjusted Anchored Neighborhood Regression for Single Image Super-Resolution.
IEEE ACCESS(2018)
摘要
The adjusted anchored neighborhood regression (A+) method is one of the state-of-the-art methods for single image super-resolution. An important implicit assumption of the A+ method is that the high-resolution (HR) image patches corresponding to similar low-resolution (LR) image patches must be similar too; therefore, the neighborhood regressions in HR patch space and LR patch space can share same representing coefficients. However, this assumption is often invalid due to the ill posedness of the super-resolution problem, and non-similar HR sample patches often share large representing coefficients. To remedy this, we propose to improve the A+ method by introducing high-resolution similarity-based adjusting weights into HR representation coefficients to reduce the effect of these non-similar HR sample patches. These adjusting weights are incorporated into the projection matrixes with low computational cost before the super-resolution processing. The numerical results demonstrate that our method can improve the performance of the A+ method effectively with low computational cost.
更多查看译文
关键词
Super-resolution,low-resolution similarity,high-resolution similarity,representation coefficients,projection matrix
AI 理解论文
溯源树
样例
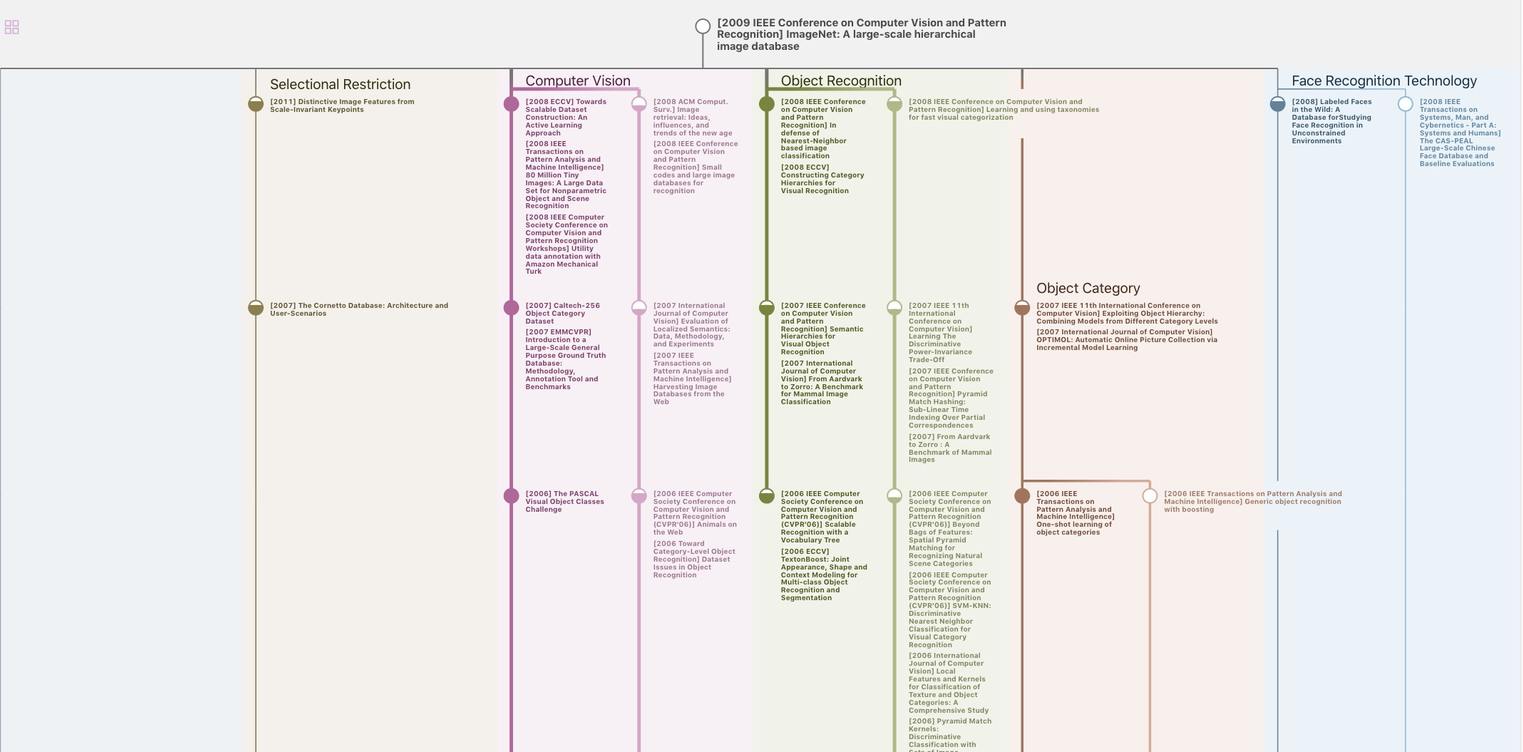
生成溯源树,研究论文发展脉络
Chat Paper
正在生成论文摘要