Swarm Intelligence Optimization Techniques for Obstacle-Avoidance Mobility-Assisted Localization in Wireless Sensor Networks.
IEEE ACCESS(2018)
摘要
In many applications of wireless sensor networks (WSNs), node location is required to locate the monitored event once occurs. Mobility-assisted localization has emerged as an efficient technique for node localization. It works on optimizing a path planning of a location-aware mobile node, called mobile anchor (MA). The task of the MA is to traverse the area of interest (network) in a way that minimizes the localization error while maximizing the number of successful localized nodes. For simplicity, many path planning models assume that the MA has a sufficient source of energy and time, and the network area is obstacle-free. However, in many real-life applications such assumptions are rare. When the network area includes many obstacles, which need to be avoided, and the MA itself has a limited movement distance that cannot be exceeded, a dynamic movement approach is needed. In this paper, we propose two novel dynamic movement techniques that offer obstacle-avoidance path planning for mobility-assisted localization in WSNs. The movement planning is designed in a real-time using two swarm intelligence based algorithms, namely grey wolf optimizer and whale optimization algorithm. Both of our proposed models, grey wolf optimizer-based path planning and whale optimization algorithm-based path planning, provide superior outcomes in comparison to other existing works in several metrics including both localization ratio and localization error rate.
更多查看译文
关键词
Wireless sensor networks,path planning,mobility models,localization models,optimization,grey wolf optimizer,whale optimization algorithm,obstacle-avoidance path planning
AI 理解论文
溯源树
样例
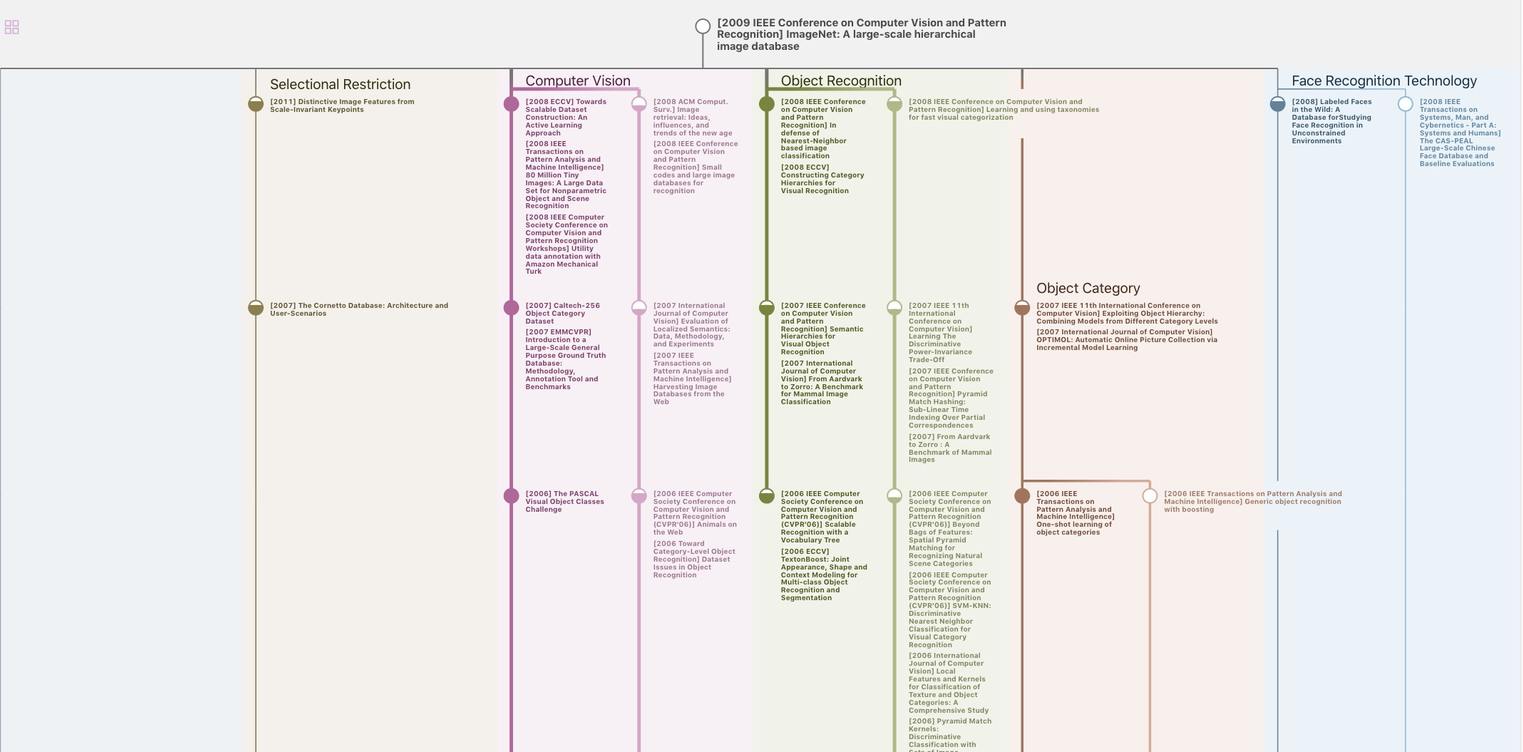
生成溯源树,研究论文发展脉络
Chat Paper
正在生成论文摘要