Randomized learning: Generalization performance of old and new theoretically grounded algorithms.
Neurocomputing(2018)
摘要
In the context of assessing the generalization abilities of a randomized model or learning algorithm, PAC-Bayes and Differential Privacy (DP) theories are the state-of-the-art tools. For this reason, in this paper, we will develop tight DP-based generalization bounds, which improve over the current state-of-the-art ones both in terms of constants and rate of convergence. Moreover, we will also prove that some old and new randomized algorithm, show better generalization performances with respect to their non private counterpart, if the DP is exploited for assessing their generalization ability. Results on a series of algorithms and real world problems show the practical validity of the achieved theoretical results.
更多查看译文
关键词
Generalization performances,Randomized models,Randomized learning algorithms,Differential privacy,PAC-Bayes,Distribution dependent prior,Data dependent posterior
AI 理解论文
溯源树
样例
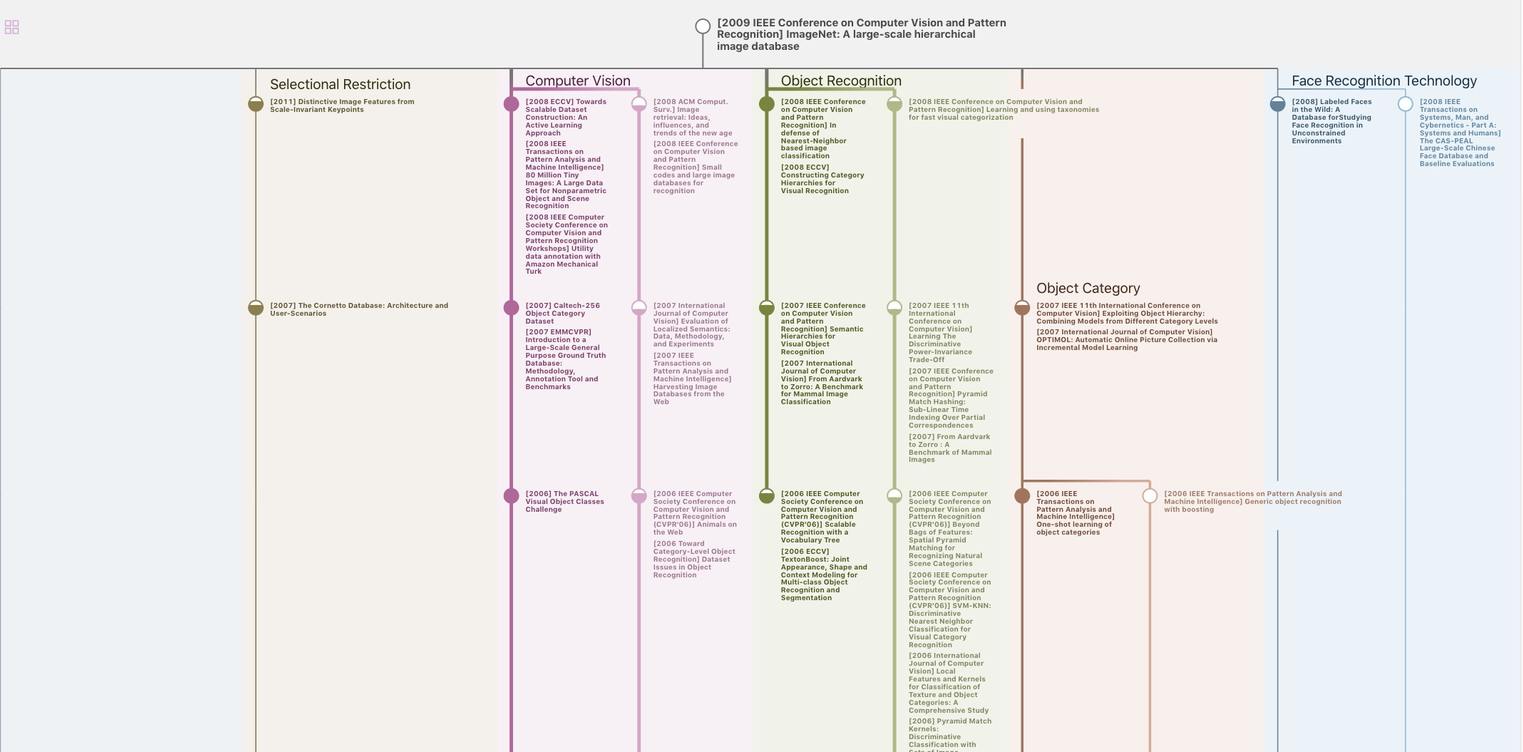
生成溯源树,研究论文发展脉络
Chat Paper
正在生成论文摘要