Observation impact, domain length and parameter estimation in data assimilation for flood forecasting.
Environmental Modelling & Software(2018)
摘要
Accurate inundation forecasting provides vital information about the behaviour of fluvial flood water. Using data assimilation with an Ensemble Transform Kalman Filter we combine forecasts from a numerical hydrodynamic model with synthetic observations of water levels. We show that reinitialising the model with corrected water levels can cause an initialisation shock and demonstrate a simple novel solution. In agreement with others, we find that although assimilation can accurately correct water levels at observation times, the corrected forecast quickly relaxes to the open loop forecast. Our new work shows that the time taken for the forecast to relax to the open loop case depends on domain length; observation impact is longer-lived in a longer domain. We demonstrate that jointly correcting the channel friction parameter as well as water levels greatly improves the forecast. We also show that updating the value of the channel friction parameter can compensate for bias in inflow.
更多查看译文
关键词
Data assimilation,Inundation forecasting,Fluvial flooding,Observation impact,Joint state-parameter estimation,Ensemble Kalman filter
AI 理解论文
溯源树
样例
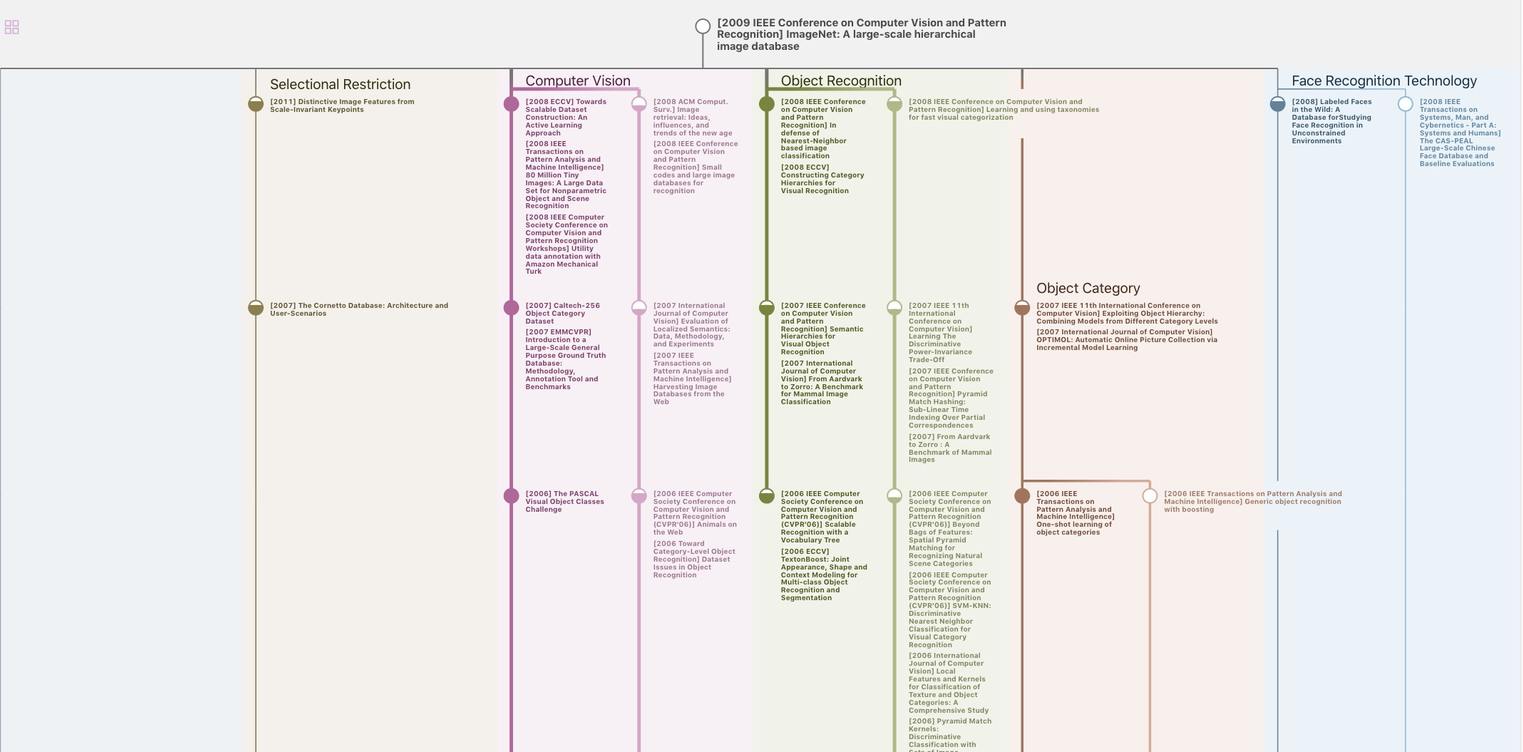
生成溯源树,研究论文发展脉络
Chat Paper
正在生成论文摘要