An Optimal First Order Method Based on Optimal Quadratic Averaging.
SIAM JOURNAL ON OPTIMIZATION(2018)
摘要
In a recent paper, Bubeck, Lee, and Singh introduced a new first order method for minimizing smooth strongly convex functions. Their geometric descent algorithm, largely inspired by the ellipsoid method, enjoys the optimal linear rate of convergence. We show that the same iterate sequence is generated by a scheme that in each iteration computes an optimal average of quadratic lower models of the function. Indeed, the minimum of the averaged quadratic approaches the true minimum at an optimal rate. This intuitive viewpoint reveals clear connections to the original fast gradient methods and cutting plane ideas, and leads to limited-memory extensions with improved performance.
更多查看译文
关键词
first order method,accelerated gradient method,convex quadratic,strong convexity
AI 理解论文
溯源树
样例
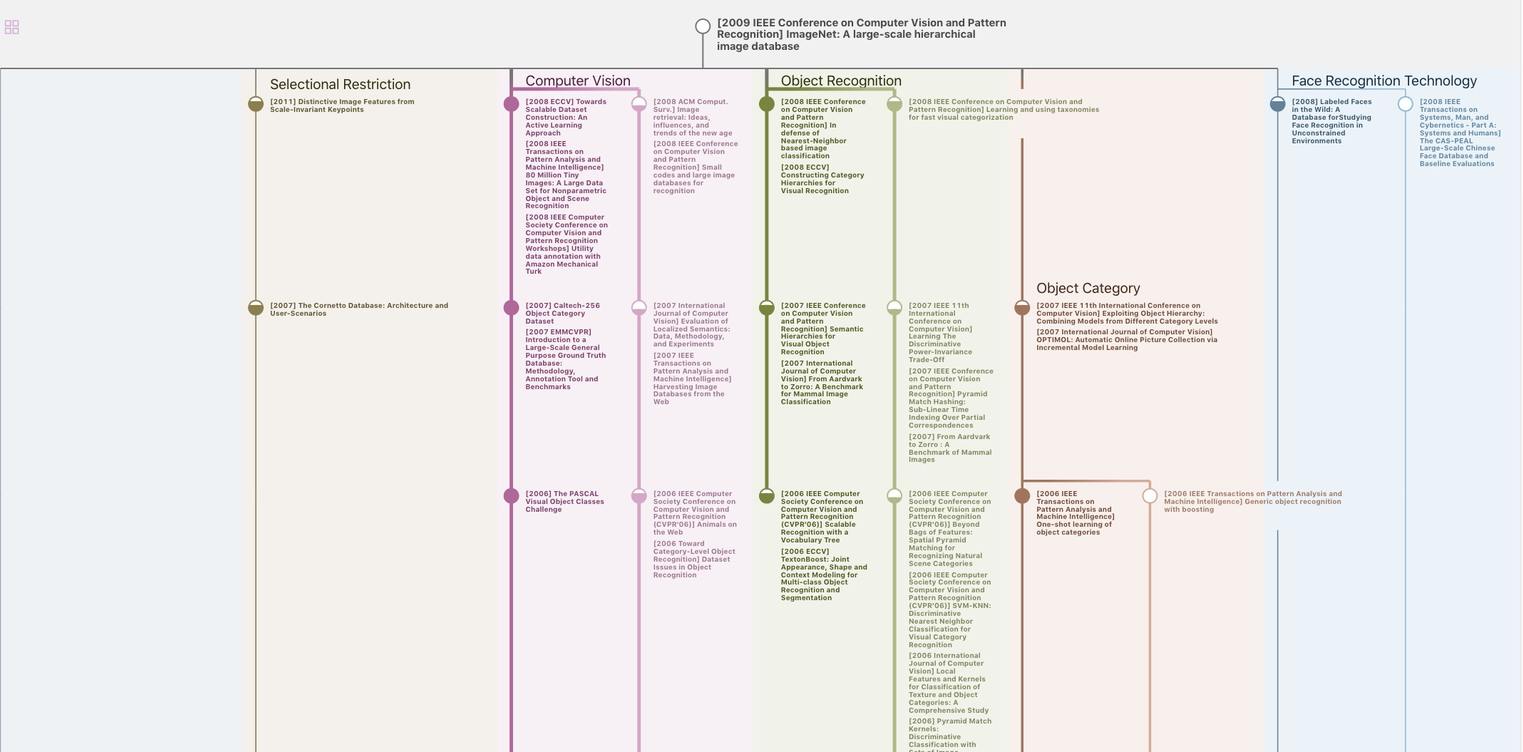
生成溯源树,研究论文发展脉络
Chat Paper
正在生成论文摘要