Using Statistical Models of Morphology in the Search for Optimal Units of Representation in the Human Mental Lexicon.
COGNITIVE SCIENCE(2018)
摘要
Determining optimal units of representing morphologically complex words in the mental lexicon is a central question in psycholinguistics. Here, we utilize advances in computational sciences to study human morphological processing using statistical models of morphology, particularly the unsupervised Morfessor model that works on the principle of optimization. The aim was to see what kind of model structure corresponds best to human word recognition costs for multimorphemic Finnish nouns: a model incorporating units resembling linguistically defined morphemes, a whole-word model, or a model that seeks for an optimal balance between these two extremes. Our results showed that human word recognition was predicted best by a combination of two models: a model that decomposes words at some morpheme boundaries while keeping others unsegmented and a whole-word model. The results support dual-route models that assume that both decomposed and full-form representations are utilized to optimally process complex words within the mental lexicon.
更多查看译文
关键词
Morphology,Mental lexicon,Statistical language modeling,Minimum Description Length principle,Unsupervised learning,Lexical decision,Word recognition,Psycholinguistics
AI 理解论文
溯源树
样例
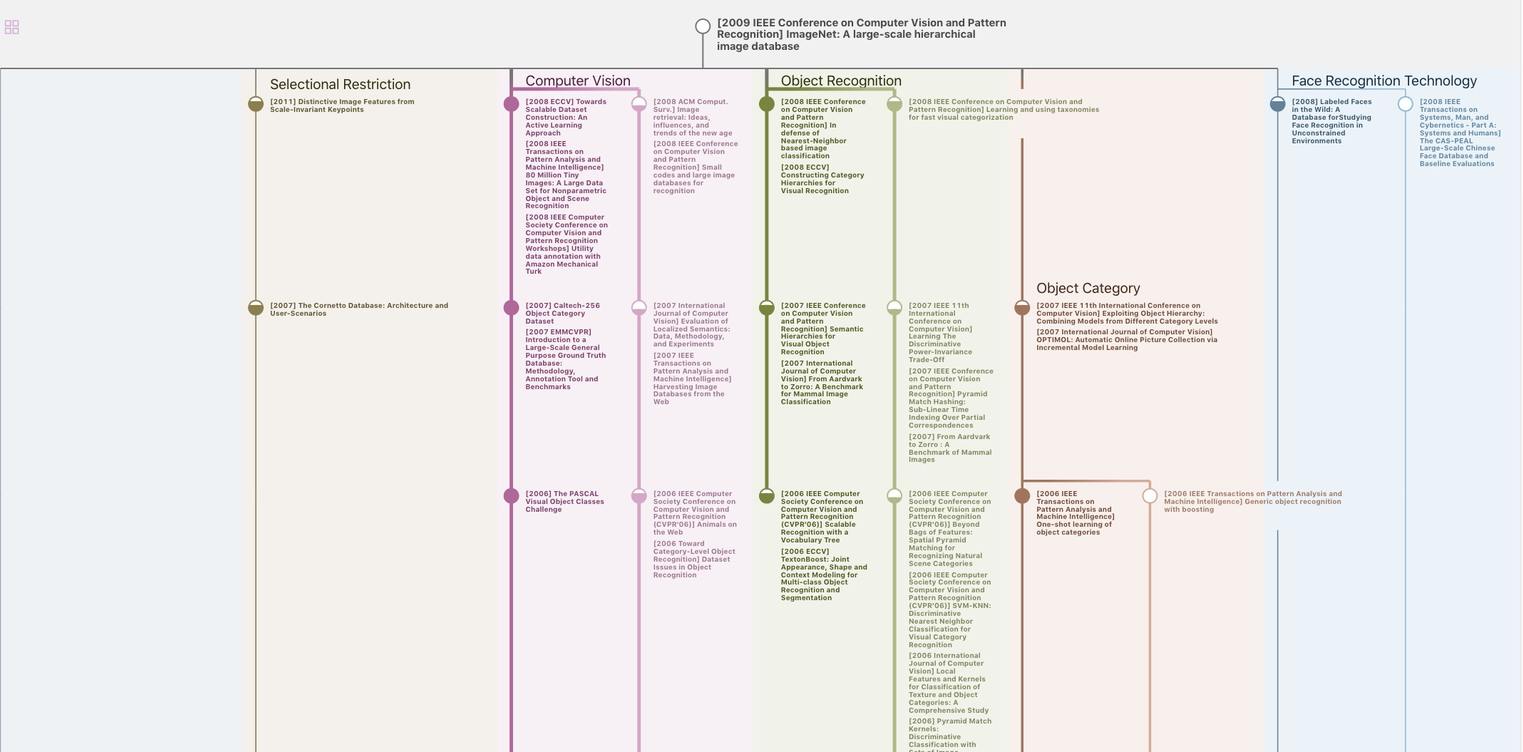
生成溯源树,研究论文发展脉络
Chat Paper
正在生成论文摘要