A Rotationally-Invariant Convolution Module by Feature Map Back-Rotation
2018 IEEE Winter Conference on Applications of Computer Vision (WACV)(2018)
摘要
Despite breakthroughs in image classification due to the evolution of deep learning and, in particular, convolutional neural networks (CNNs), state-of-the-art models only possess a very limited amount of rotational invariance. Known workarounds include artificial rotations of the training data or ensemble approaches, where several models are evaluated. These approaches either increase the workload of the training or inflate the number of parameters. Further approaches add rotational invariance by globally pooling over rotationally equivariant features. Instead, we propose to incorporate rotational invariance into the feature-extraction part of the CNN directly. This allows to train on unrotated data and perform well on a rotated test set. We use rotational convolutions and introduce a rotational pooling layer that performs a pooling over the back-rotated output feature maps. We show that when training on the original, unrotated MNIST training dataset, but evaluating on rotations of the MNIST test dataset, the error rate can be reduced substantially from 58.20% to 12.20%. Similar results are shown for the CIFAR-10 and CIFAR-100 datasets.
更多查看译文
关键词
rotationally-invariant convolution module,feature map back-rotation,artificial rotations,training data,rotationally equivariant features,rotational convolutions,rotational pooling layer,unrotated MNIST training dataset,feature-extraction,image classification,deep learning,convolutional neural networks,CNNs
AI 理解论文
溯源树
样例
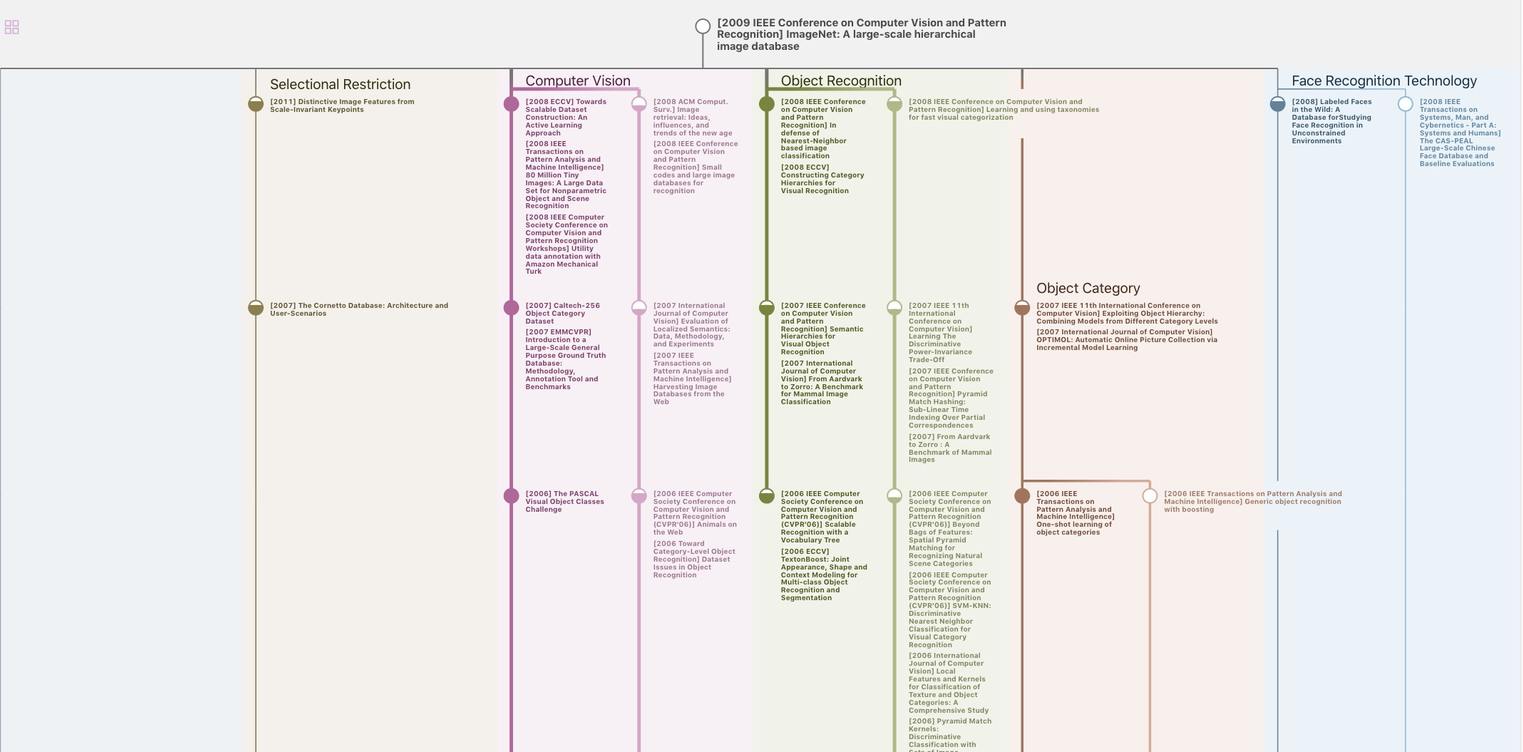
生成溯源树,研究论文发展脉络
Chat Paper
正在生成论文摘要