Learning to See Through Turbulent Water
2018 IEEE Winter Conference on Applications of Computer Vision (WACV)(2018)
摘要
Imaging through dynamic refractive media, such as looking into turbulent water, or through hot air, is challenging since light rays are bent by unknown amounts leading to complex geometric distortions. Inverting these distortions and recovering high quality images is an inherently ill-posed problem, leading previous works to require extra information such as high frame-rate video or a template image, which limits their applicability in practice. This paper proposes training a deep convolution neural network to undistort dynamic refractive effects using only a single image. The neural network is able to solve this ill-posed problem by learning image priors as well as distortion priors. Our network consists of two parts, a warping net to remove geometric distortion and a color predictor net to further refine the restoration. Adversarial loss is used to achieve better visual quality and help the network hallucinate missing and blurred information. To train our network, we collect a large training set of images distorted by a turbulent water surface. Unlike prior works on water undistortion, our method is trained end-to-end, only requires a single image and does not use a ground truth template at test time. Experiments show that by exploiting the structure of the problem, our network outperforms state-of-the-art deep image to image translation.
更多查看译文
关键词
turbulent water surface,water undistortion,image translation,dynamic refractive media,light rays,unknown amounts,complex geometric distortions,high frame-rate video,template image,deep convolution neural network,undistort dynamic refractive effects,image priors,distortion priors,warping net,geometric distortion,color predictor net,visual quality,high quality images,network hallucinate missing information
AI 理解论文
溯源树
样例
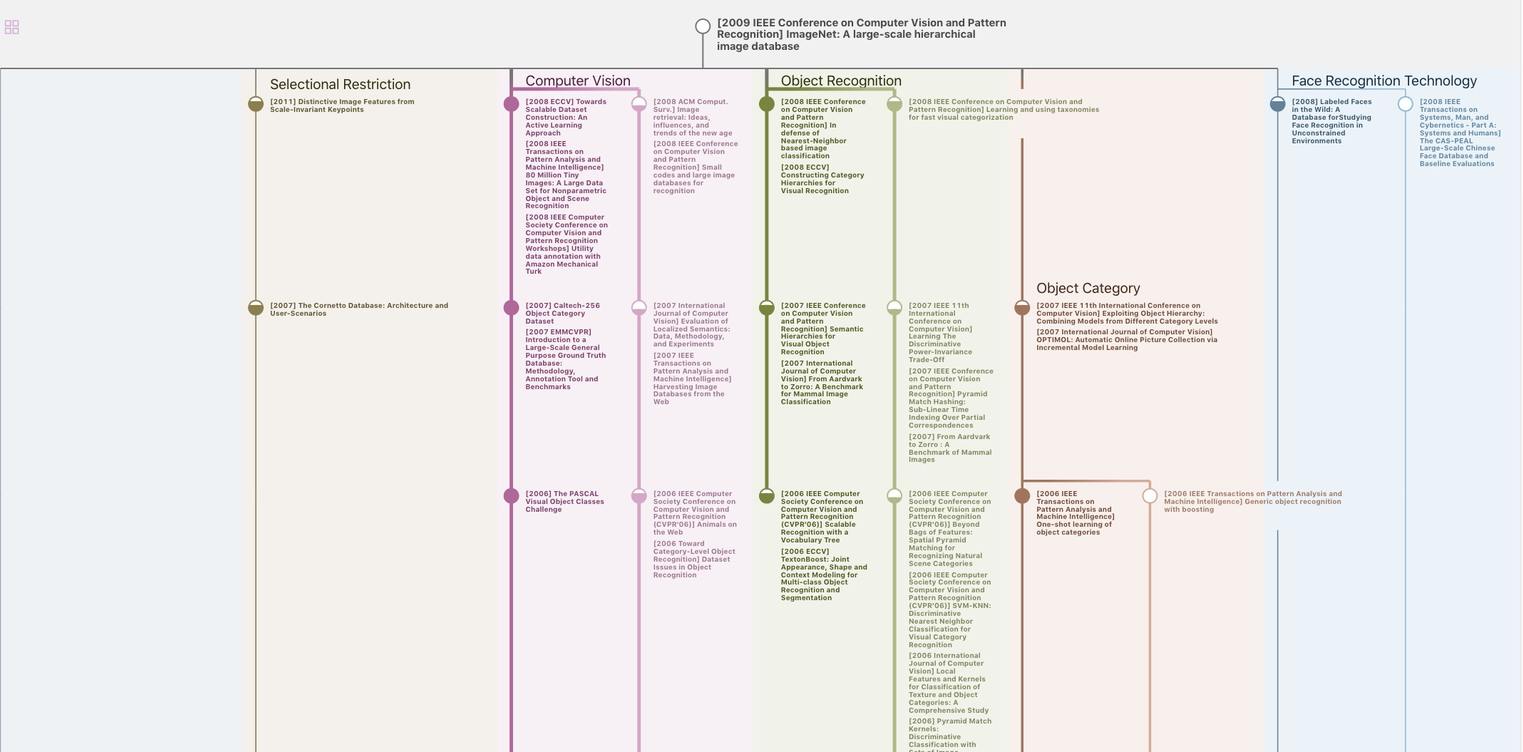
生成溯源树,研究论文发展脉络
Chat Paper
正在生成论文摘要