Multi-View Discriminative Learning Via Joint Non-Negative Matrix Factorization
DATABASE SYSTEMS FOR ADVANCED APPLICATIONS (DASFAA 2018), PT II(2018)
摘要
Multi-view learning attempts to generate a classifier with a better performance by exploiting relationship among multiple views. Existing approaches often focus on learning the consistency and/or complementarity among different views. However, not all consistent or complementary information is useful for learning, instead, only class-specific discriminative information is essential. In this paper, we propose a new robust multi-view learning algorithm, called DICS, by exploring the Discriminative and non-discriminative Information existing in Common and view-Specific parts among different views via joint non-negative matrix factorization. The basic idea is to learn a latent common subspace and view-specific subspaces, and more importantly, discriminative and non-discriminative information from all subspaces are further extracted to support a better classification. Empirical extensive experiments on seven real-world data sets have demonstrated the effectiveness of DICS, and show its superiority over many state-of-the-art algorithms.
更多查看译文
关键词
Multi-view learning, Matrix factorization, Classification
AI 理解论文
溯源树
样例
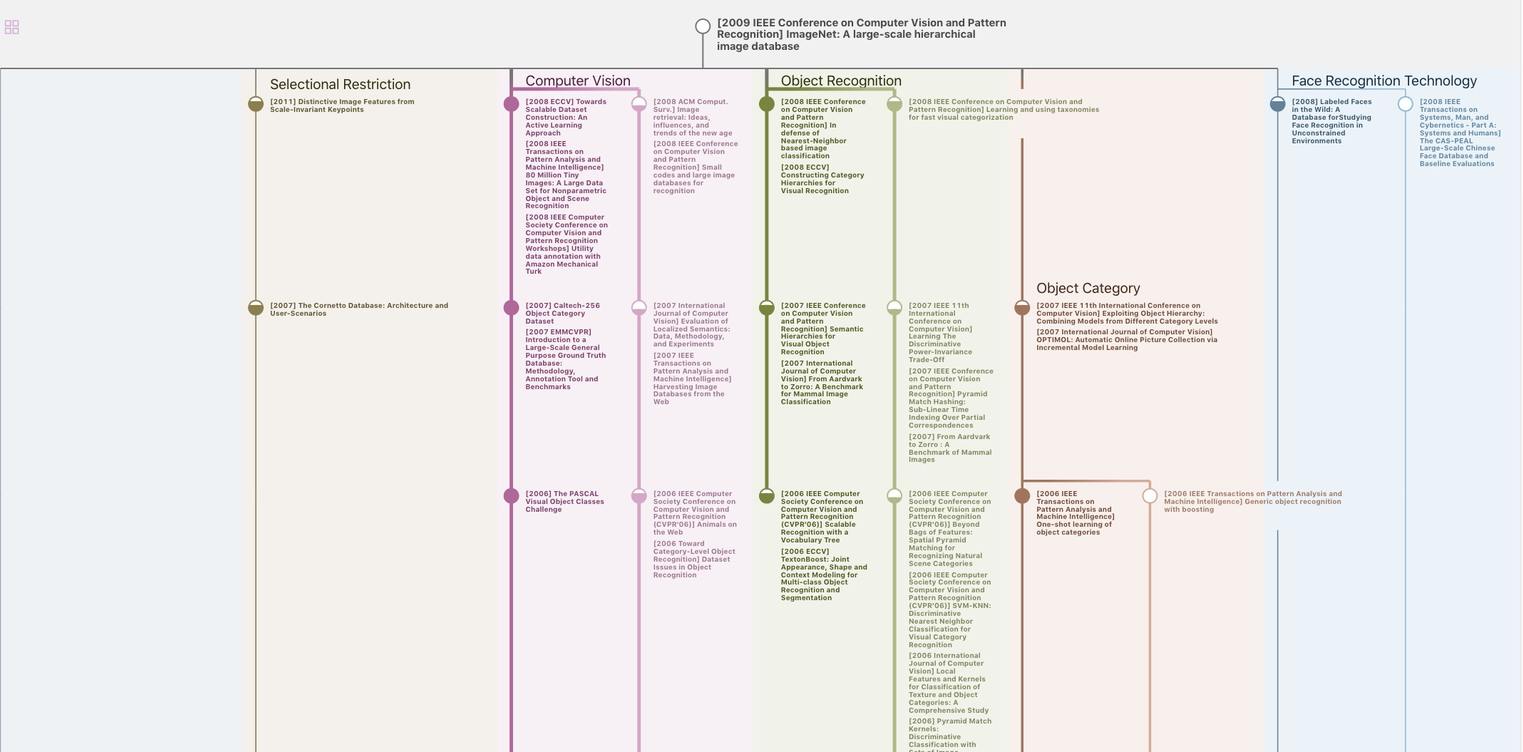
生成溯源树,研究论文发展脉络
Chat Paper
正在生成论文摘要