Learning Distribution-Matched Landmarks For Unsupervised Domain Adaptation
DATABASE SYSTEMS FOR ADVANCED APPLICATIONS (DASFAA 2018), PT II(2018)
摘要
Domain adaptation is widely used in database applications, especially in data mining. The basic assumption of domain adaptation (DA) is that some latent factors are shared by the source domain and the target domain. Revealing these shared factors, as a result, is the core operation of many DA approaches. This paper proposes a novel approach, named Learning Distribution-Matched Landmarks (LDML), for unsupervised DA. LDML reveals the latent factors by learning a domain-invariant subspace where the two domains are well aligned at both feature level and sample level. At the feature level, the divergences of both the marginal distribution and the conditional distribution are mitigated. At the sample level, each sample is evaluated so that we can take full advantage of the pivotal samples and filter out the outliers. Extensive experiments on two standard benchmarks verify that our approach can outperform state-of-the-art methods with significant advantages.
更多查看译文
关键词
Domain adaptation, Transfer learning, Landmark selection
AI 理解论文
溯源树
样例
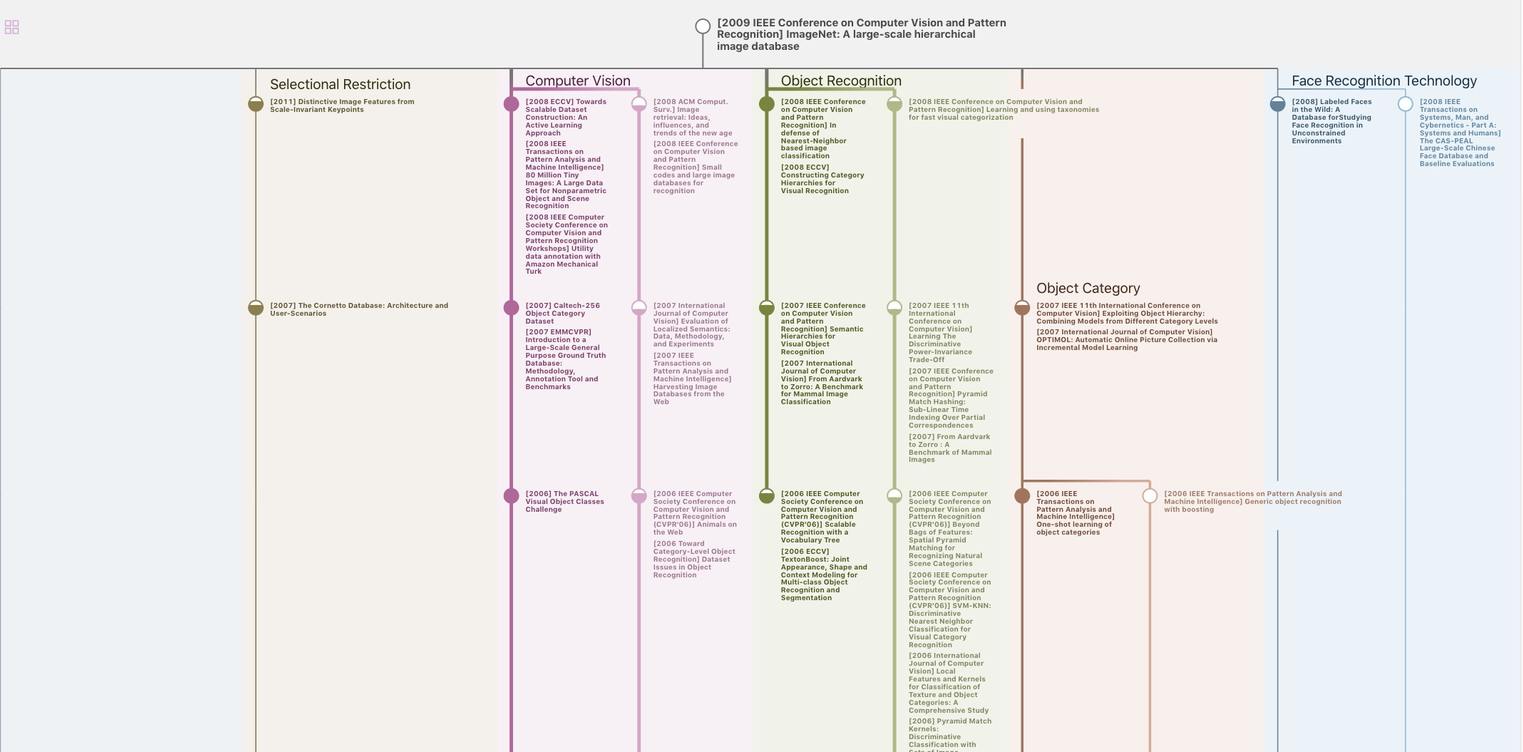
生成溯源树,研究论文发展脉络
Chat Paper
正在生成论文摘要