Unsupervised Cross-Modal Hashing with Soft Constraint.
ADVANCES IN MULTIMEDIA INFORMATION PROCESSING - PCM 2017, PT II(2017)
摘要
The booming demands for cross-modal retrieval tasks can often bring in its wake the development of retrieval technologies, as people turn to pursuing a more effective way to improve the performance of search results in both accuracy and efficiency, for example by unsupervised cross-modal hashing. It's worth noting that most of the cross-modal hashing methods focus on utilizing merely one approach to generate hash codes. However, each approach has its own intrinsic drawback, which would inevitably diminish the quality of hash codes. In this paper, we propose a state-of-the-art model named Soft Constraint Hashing (SCH), using a special soft constraint term defined as an "information tunnel" to achieve the goal that conveys information from one approach to another. In particular, this "tunnel" can eliminate potential data noises to some extent and bridge the gap between two unsupervised discrete hashing allocation approaches to simultaneously reinforce the quality of hash codes. The empirical results on publicly available datasets illustrate that our proposed model outperforms all the existing unsupervised cross-model hashing methods.
更多查看译文
关键词
Unsupervised hashing,Multi-modal data,Soft constraint
AI 理解论文
溯源树
样例
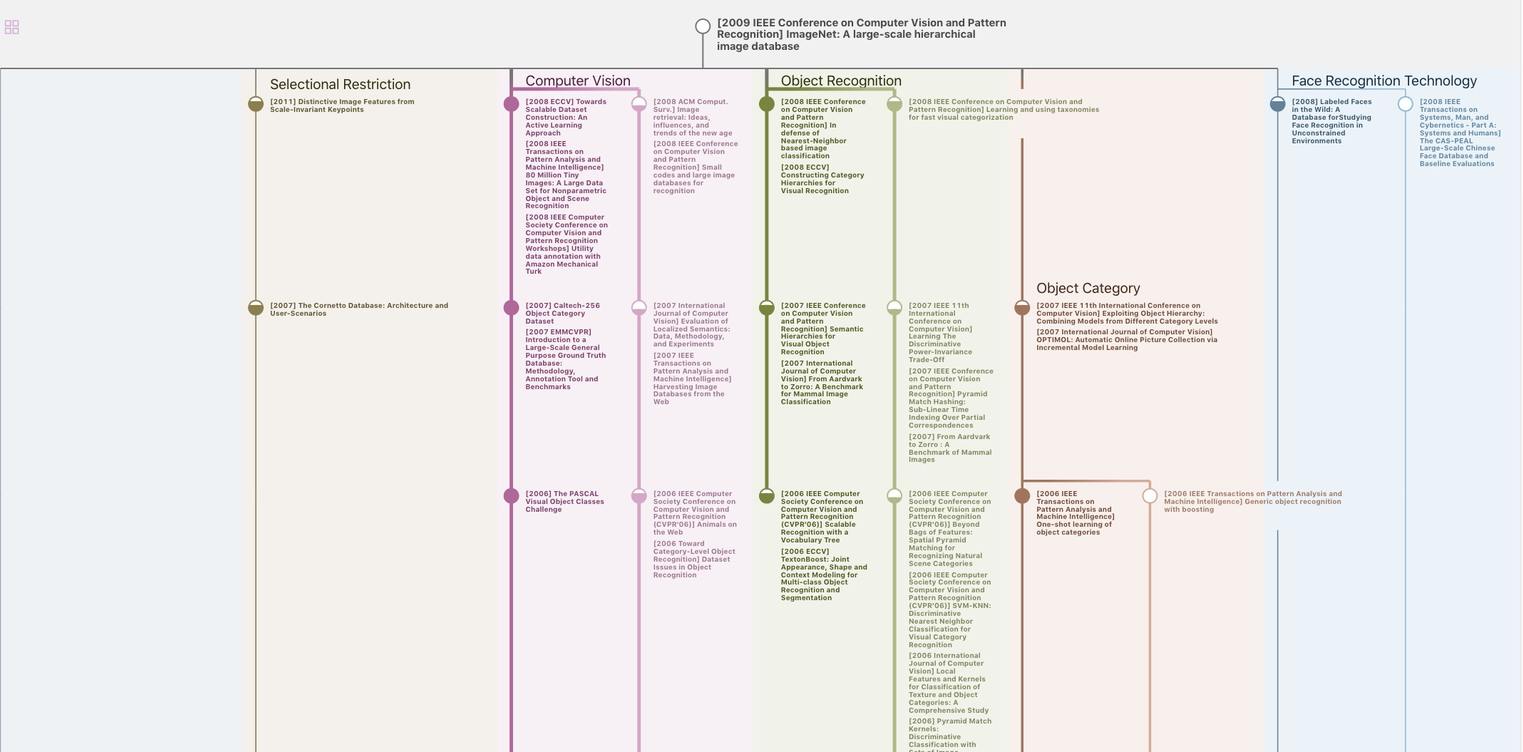
生成溯源树,研究论文发展脉络
Chat Paper
正在生成论文摘要