Learning real-time ambient occlusion from distance representations.
I3D(2018)
摘要
The computation of partial occlusion, as required for ambient occlusion or soft shadows, provides visually important cues but is notoriously expensive. In this paper we propose a novel solution to the ambient occlusion problem, combining signed distance scene representations and machine learning. We demonstrate how to learn and apply mappings which approximate a ray traced ground truth occlusion using only a few nearby samples of a signed distance representation. As representation for our trained mappings we use small feed-forward neural networks which are fast to evaluate, allowing for real-time occlusion queries. Our ambient occlusion approximation outperforms state-of-the-art methods in both quality and performance, yielding temporally stable and smooth results. Since our training data is different from typical machine learning approaches which mostly deal with 2D/3D image data and our techniques are also applicable to other occlusion problems (e.g. soft shadows), we give an in-depth overview of our framework. Furthermore, we discuss arising artifacts and possible extensions of our approach.
更多查看译文
关键词
real-time rendering,neural networks,machine learning,ambient occlusion,signed distance representations
AI 理解论文
溯源树
样例
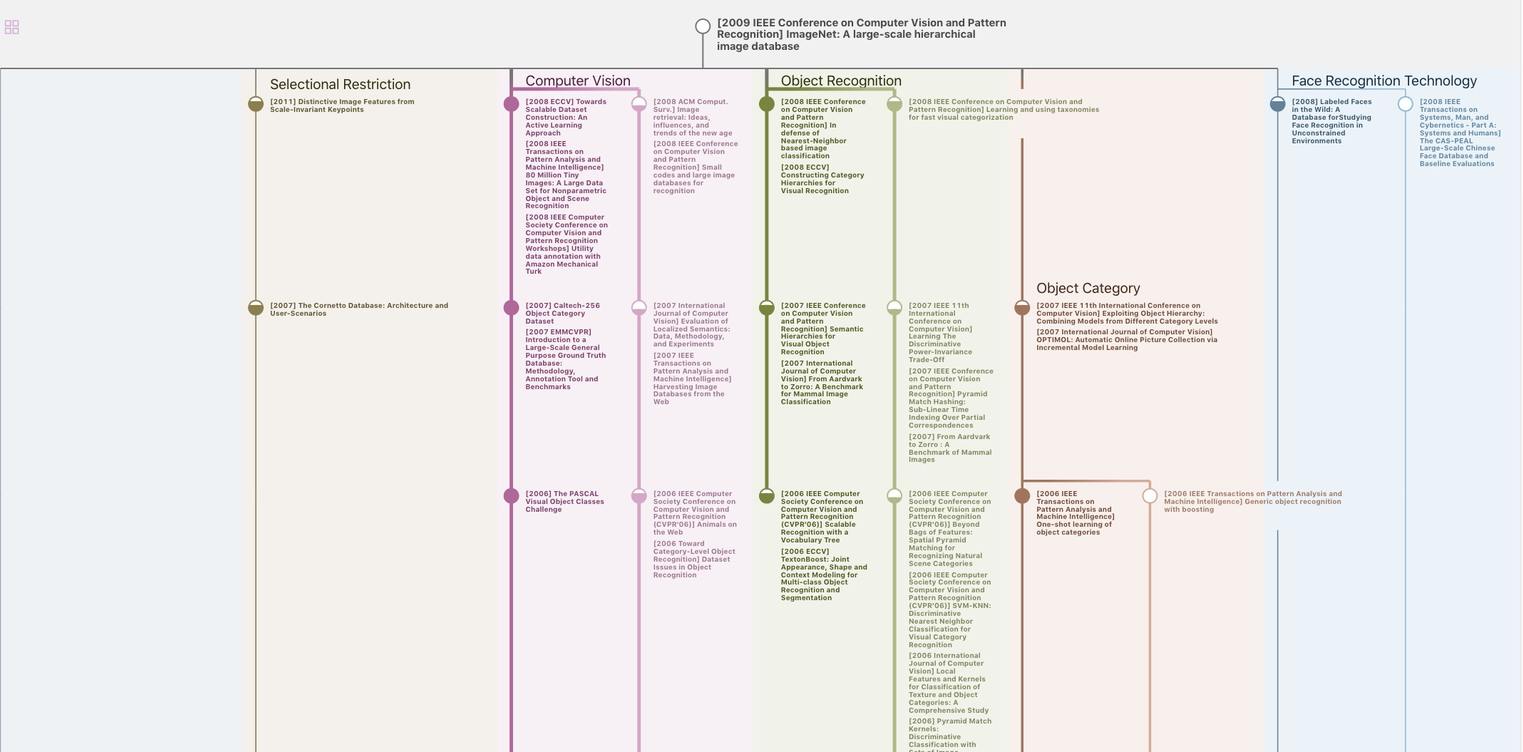
生成溯源树,研究论文发展脉络
Chat Paper
正在生成论文摘要