Bayesian Verb Sense Clustering.
THIRTY-SECOND AAAI CONFERENCE ON ARTIFICIAL INTELLIGENCE / THIRTIETH INNOVATIVE APPLICATIONS OF ARTIFICIAL INTELLIGENCE CONFERENCE / EIGHTH AAAI SYMPOSIUM ON EDUCATIONAL ADVANCES IN ARTIFICIAL INTELLIGENCE(2018)
摘要
This work performs verb sense induction and clustering based on observed syntactic distributions in a large corpus. VerbNet is a hierarchical clustering of verbs and a useful semantic resource. We address the main drawbacks of VerbNet, by proposing a Bayesian model to build VerbNet-like clusters automatically and with full coverage. Relative to the prior state of the art, we improve accuracy on verb sense induction by over 20% absolute FL We then propose a new model, inspired by the positive pointwise mutual information (PPMI). Our PPMI-based mixture model permits an extremely efficient sampler, while improving performance. Our best model shows a 4.5% absolute F1 improvement over the best non-PPMI model, with over an order of magnitude less computation time. Though this model is inspired by clustering verb senses, it may be applicable in other situations where multiple items are being sampled as a group.
更多查看译文
AI 理解论文
溯源树
样例
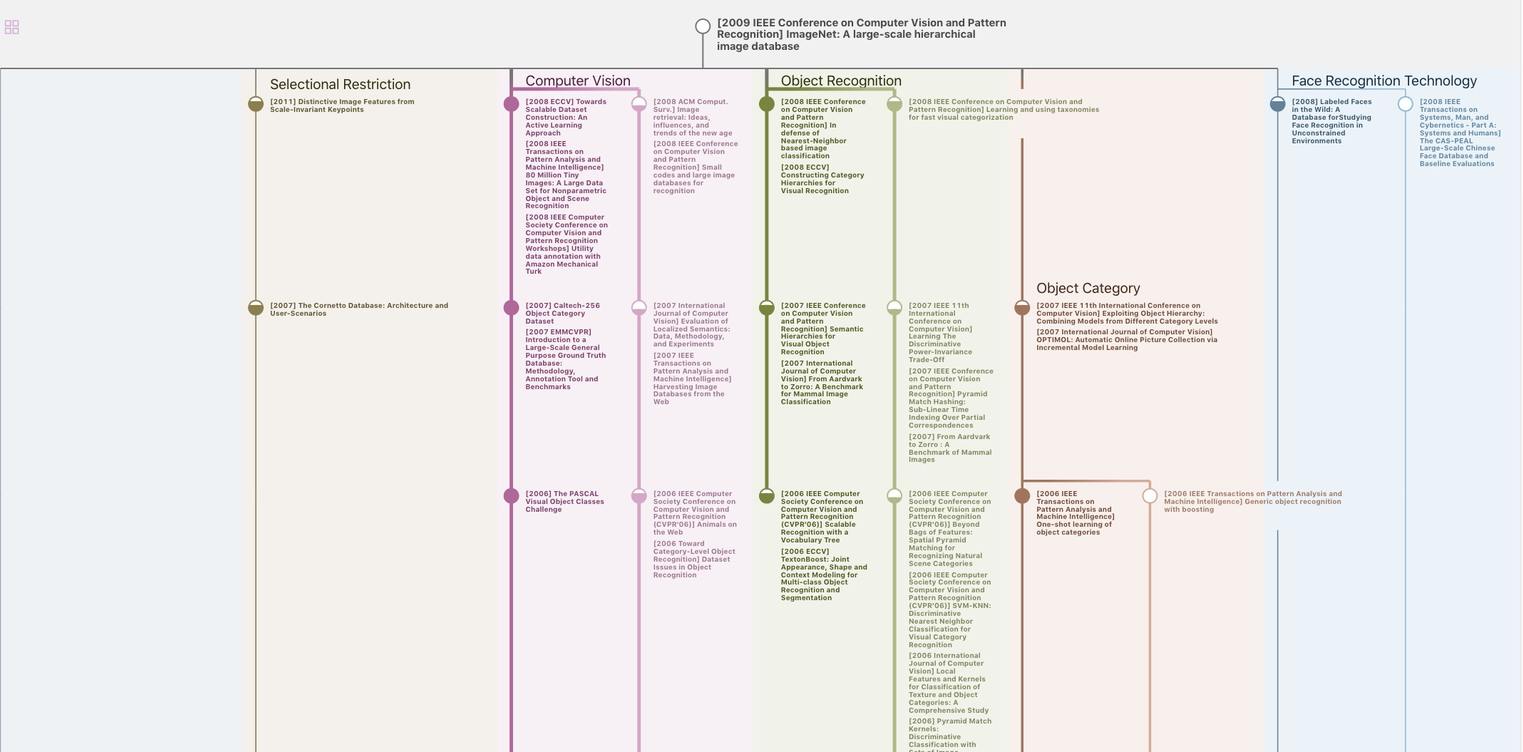
生成溯源树,研究论文发展脉络
Chat Paper
正在生成论文摘要