Linguistic Properties Matter for Implicit Discourse Relation Recognition: Combining Semantic Interaction, Topic Continuity and Attribution.
THIRTY-SECOND AAAI CONFERENCE ON ARTIFICIAL INTELLIGENCE / THIRTIETH INNOVATIVE APPLICATIONS OF ARTIFICIAL INTELLIGENCE CONFERENCE / EIGHTH AAAI SYMPOSIUM ON EDUCATIONAL ADVANCES IN ARTIFICIAL INTELLIGENCE(2018)
摘要
Modern solutions for implicit discourse relation recognition largely build universal models to classify all of the different types of discourse relations. In contrast to such learning models, we build our model from first principles, analyzing the linguistic properties of the individual top-level Penn Discourse Treebank (PDTB) styled implicit discourse relations: Comparison, Contingency and Expansion. We find semantic characteristics of each relation type and two cohesion devices -topic continuity and attribution - work together to contribute such linguistic properties. We encode those properties as complex features and feed them into a Naive Bayes classifier, bettering baselines (including deep neural network ones) to achieve a new state-of-the-art performance level. Over a strong, feature-based baseline, our system outperforms oneversus- other binary classification by 4.83% for Comparison relation, 3.94% for Contingency and 2.22% for four-way classification.
更多查看译文
AI 理解论文
溯源树
样例
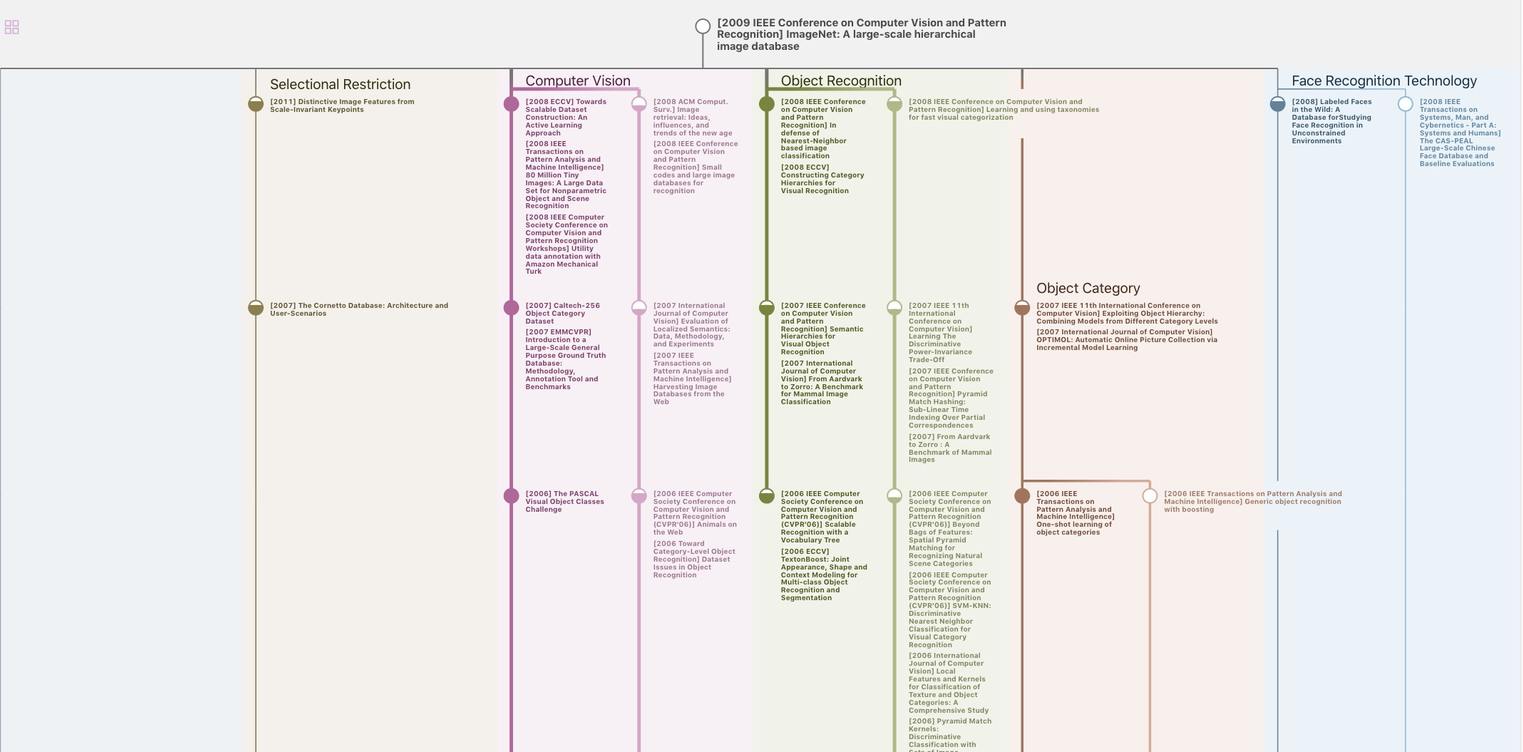
生成溯源树,研究论文发展脉络
Chat Paper
正在生成论文摘要