An Unsupervised Degradation Estimation Framework For Diagnostics And Prognostics In Cyber-Physical System
2018 IEEE 4TH WORLD FORUM ON INTERNET OF THINGS (WF-IOT)(2018)
摘要
In order to learn the performance degradation mode of machines for diagnostics and prognostics in Cyber-Physical System (CPS), it is necessary to analyze observed sensor data to find the internal run-to-failure states of a system. In this paper, the research goal focuses on learning the internal state and transition of the degraded states from original data, which has some advantages to reveal the working dynamics of the system. Due to the existence of unlabeled data, the paper proposes an unsupervised framework based on clustering and Hidden Markov Model, named Cluster-based Hidden Markov Model (cHMM). The cHMM aims at converting raw sensory stream into a sequence of symbols as the initial observation and hidden state sequences, and then an extended Viterbi algorithm based on Hidden Markov Model (HMM) is used to discover the final stable hidden states and transitional rules in a dynamic programming way. Based on the learned model and expert's knowledge, performance degradation failure and the root-cause could be predicted and reasoned. Finally, experiments and proof-of-concept demonstration are given to validate the feasibility and effectiveness of the framework based on C-MAPSS turbofan engine dataset.
更多查看译文
关键词
degraded states,working dynamics,unlabeled data,cHMM,hidden state sequences,performance degradation failure,C-MAPSS turbofan engine dataset,stable hidden states,internal run-to-failure states,cyber-physical system diagnostics,cyber-physical system prognostics,cluster-based hidden Markov model,unsupervised degradation estimation framework
AI 理解论文
溯源树
样例
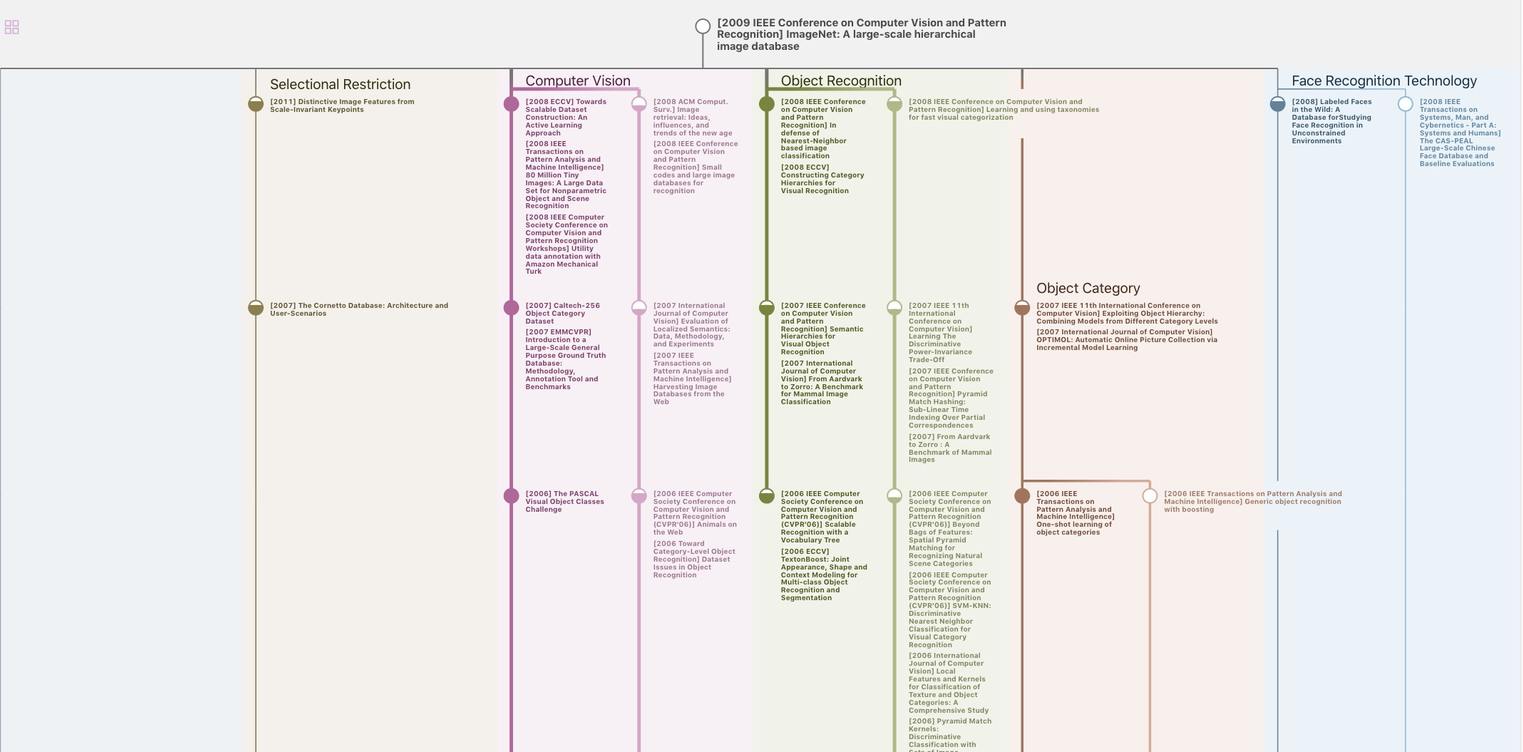
生成溯源树,研究论文发展脉络
Chat Paper
正在生成论文摘要